Optimizing Wind Energy Production: Leveraging Deep Learning Models Informed with On-Site Data and Assessing Scalability through HPC
ACTA POLYTECHNICA HUNGARICA(2024)
摘要
This study suggests employing a deep learning model trained on on-site wind speed measurements to enhance predictions for future wind speeds. The model uses a gated recurrent unit (GRU) derived from the long short-term memory (LSTM) variant, and is trained using actual measured wind velocity data collected at both 10-minute and hourly intervals. The approach relies on using same-season data for predicting wind velocity, necessitating regular updates to the model with recent measurements to ensure accurate predictions in a timely manner. The results from the prediction model, particularly at a 10-minute interval, demonstrate a significant alignment with the actual data during validation. Comparative analysis of the employed model over a two-year span, with a 24-year distinction, indicates its efficiency across different time periods and seasonal conditions, contingent upon frequent updates with recent on-site wind velocity data. Given the reliance of sequential deep learning models on extensive data for enhanced accuracy, this study emphasizes the importance of employing high-performance computing (HPC). As a recommendation, the study proposes equipping the wind farm or wind farm cluster with an HPC machine powered by the wind farm itself, thereby transforming it into a sustainable green energy resource for the HPC application. The recommended approach in this work is enforcing the smart power grid to respond to the power demand that is connected to predictable wind farm production.
更多查看译文
关键词
Deep Learning,Wind Energy,Wind Turbine,Smart Grid,Renewable Energy Prediction,High-performance computing
AI 理解论文
溯源树
样例
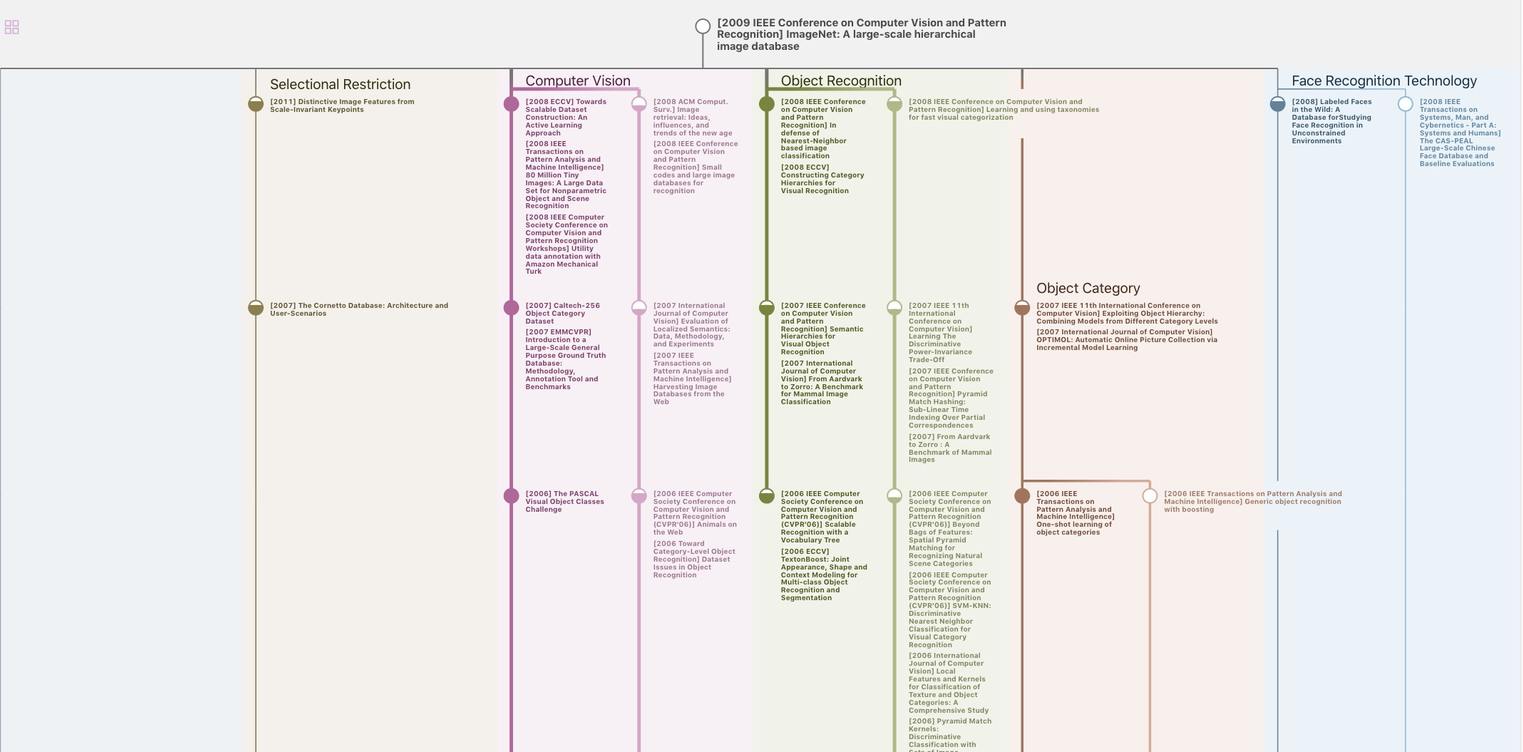
生成溯源树,研究论文发展脉络
Chat Paper
正在生成论文摘要