Comparison of Machine Learning Tree-Based Algorithms to Predict Future Paratuberculosis ELISA Results Using Repeat Milk Tests
ANIMALS(2024)
摘要
Simple Summary Johne's disease is a chronic progressive gastrointestinal disease of ruminants. The diagnosis and control of this disease remains a challenge for cattle producers and herd veterinarians. Machine learning is a broad class of algorithms and statistical analysis belonging to the area of artificial intelligence. These techniques allow for the recognition of patterns in large datasets that are not found by traditional analytical and statistical methods. While artificial intelligence and machine learning have been used in human medicine and in various aspects of animal husbandry, they have only just started to be used in Johne's disease research. By using results from milk component tests and past Johne's individual test results, tree-based models, a type of machine learning, were used to predict future Johne's results. This type of predictive algorithm may help producers and veterinarians more reliably identify those animals most likely to yield a positive result. This will, in turn, help improve control efforts and reduce the testing burden for herds working towards disease control and eradication.Abstract Machine learning algorithms have been applied to various animal husbandry and veterinary-related problems; however, its use in Johne's disease diagnosis and control is still in its infancy. The following proof-of-concept study explores the application of tree-based (decision trees and random forest) algorithms to analyze repeat milk testing data from 1197 Canadian dairy cows and the algorithms' ability to predict future Johne's test results. The random forest models using milk component testing results alongside past Johne's results demonstrated a good predictive performance for a future Johne's ELISA result with a dichotomous outcome (positive vs. negative). The final random forest model yielded a kappa of 0.626, a roc AUC of 0.915, a sensitivity of 72%, and a specificity of 98%. The positive predictive and negative predictive values were 0.81 and 0.97, respectively. The decision tree models provided an interpretable alternative to the random forest algorithms with a slight decrease in model sensitivity. The results of this research suggest a promising avenue for future targeted Johne's testing schemes. Further research is needed to validate these techniques in real-world settings and explore their incorporation in prevention and control programs.
更多查看译文
关键词
paratuberculosis,Johne's disease,disease control,machine learning,random forest,decision tree,diagnostics,dairy farming,cattle
AI 理解论文
溯源树
样例
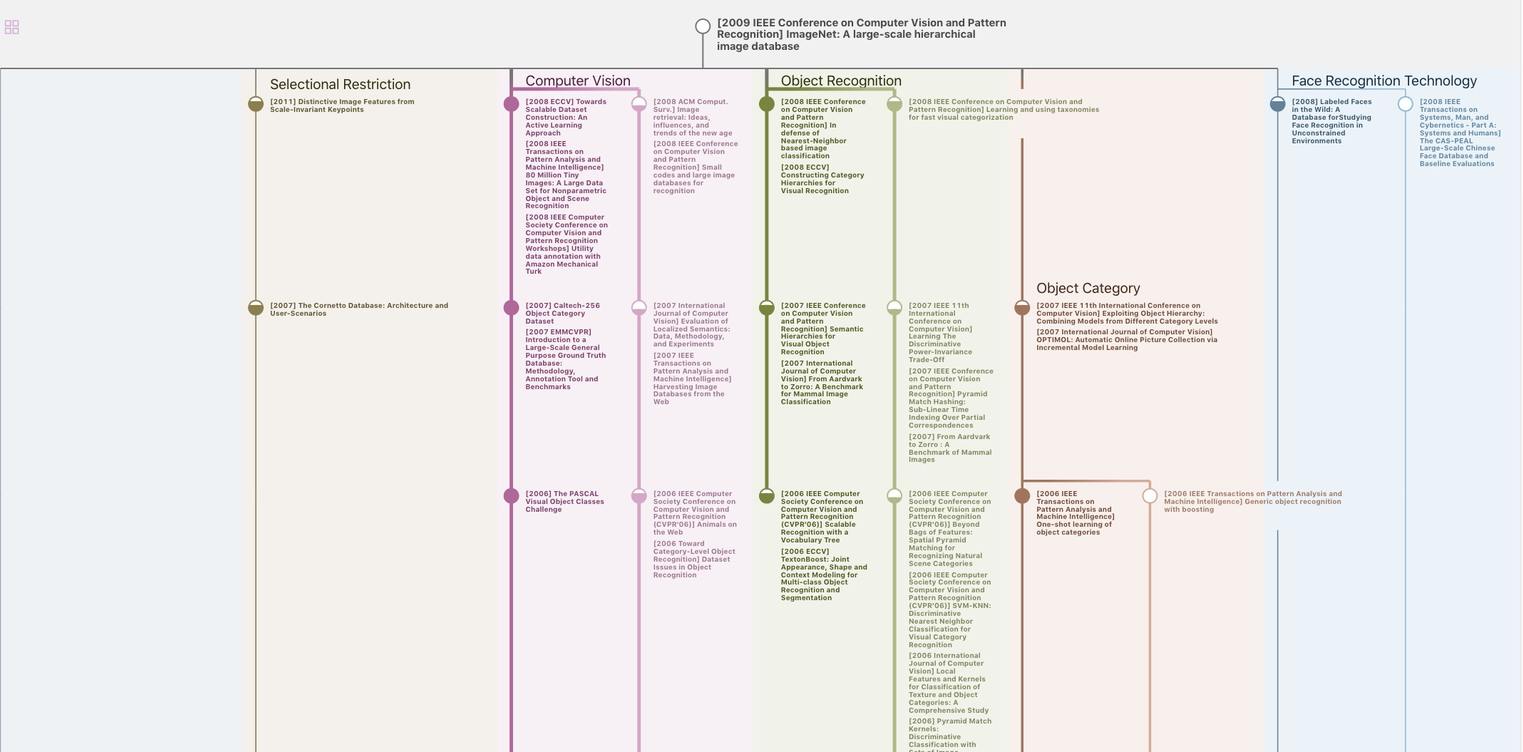
生成溯源树,研究论文发展脉络
Chat Paper
正在生成论文摘要