A novel hybrid ensemble model for mineral prospectivity prediction: A case study in the Malipo W-Sn mineral district, Yunnan Province, China
Ore Geology Reviews(2024)
Abstract
The utilization of machine learning and data-driven approaches for mineral prospectivity modeling has become increasingly important in supporting regional mineral exploration. However, supervised learning methods have encountered several common challenges, such as overfitting and underfitting, in the process of mineral prospectivity modeling, particularly due to imbalanced sample sizes used for model training. This imbalance could limit the generalization capability of models and result in inadequate predictive performance of individual models. The present study introduced a novel hybrid ensemble model (HEM) that integrates various learner methods, including generative adversarial network (GAN) and transfer learning techniques, to effectively address the issues above. Intelligent prediction techniques were applied to analyze the geological, geochemical, and geophysical dataset in order to delineate potential areas for W-Sn mineralization in Malipo County, Yunnan Province, China. Meanwhile, some well-established supervised learning methods such as Convolutional Neural Networks (CNN), Boosting-based model (e.g., LightGBM), and Bagging-based models, which demonstrated exceptional performance in dealing with classification issues were also integrated into the HEM. The weights for integrating these models were determined automatically using the equidistant-sampling technique. The superior performance of the HEM in identifying known deposits made it an efficient tool for mineral prediction mapping. Additionally, this model also offered high flexibility and scalability by allowing adjustments to the types and quantities of base learners based on data characteristics.
MoreTranslated text
Key words
Mineral prospectivity prediction,Ensemble model,Generative adversarial networks,Transfer learning
AI Read Science
Must-Reading Tree
Example
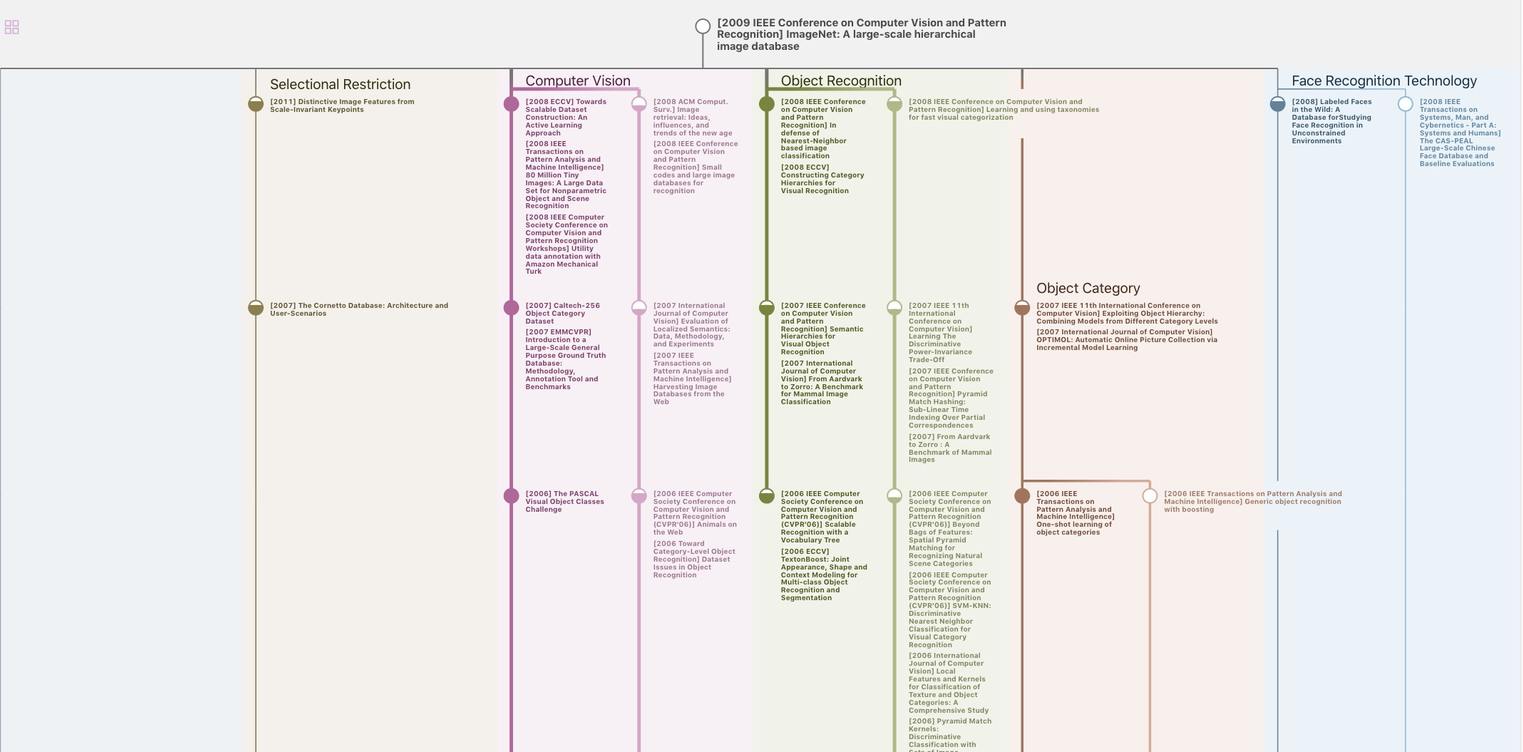
Generate MRT to find the research sequence of this paper
Chat Paper
Summary is being generated by the instructions you defined