Symptom-based drug prediction of lifestyle-related chronic diseases using unsupervised machine learning techniques
Computers in Biology and Medicine(2024)
摘要
Background and objectives
Lifestyle-related diseases (LSDs) impose a substantial economic burden on patients and health care services. LSDs are chronic in nature and can directly affect the heart and lungs. Therapeutic interventions only based on symptoms can be crucial for prompt treatment initiation in LSDs, as symptoms are the first information available to clinicians. So, this work aims to apply unsupervised machine learning (ML) techniques for developing models to predict drugs from symptoms for LSDs, with a specific focus on pulmonary and heart diseases.
Methods
The drug-disease and disease-symptom associations of 143 LSDs, 1271 drugs, and 305 symptoms were used to compute direct associations between drugs and symptoms. ML models with four different algorithms – K-Means, Bisecting K-Means, Mean Shift, and Balanced Iterative Reducing and Clustering using Hierarchies (BIRCH) – were developed to cluster the drugs using symptoms as features. The optimal model was saved in a server for the development of a web application. A web application was developed to perform the prediction based on the optimal model.
Results
The Bisecting K-means model showed the best performance with a silhouette coefficient of 0.647 and generated 138 drug clusters. The drugs within the optimal clusters showed good similarity based on i) gene ontology annotations of the gene targets, ii) chemical ontology annotations, and iii) maximum common substructure of the drugs. In the web application, the model also provides a confidence score for each predicted drug while predicting from a new set of input symptoms.
Conclusion
In summary, direct associations between drugs and symptoms were computed, and those were used to develop a symptom-based drug prediction tool for LSDs with unsupervised ML models. The ML-based prediction can provide a second opinion to clinicians to aid their decision-making for early treatment of LSD patients. The web application (URL - http://bicresources.jcbose.ac.in/ssaha4/sdldpred) can provide a simple interface for all end-users to perform the ML-based prediction.
更多查看译文
关键词
Lifestyle-related diseases,Drugs,Symptoms,Machine learning,Clustering
AI 理解论文
溯源树
样例
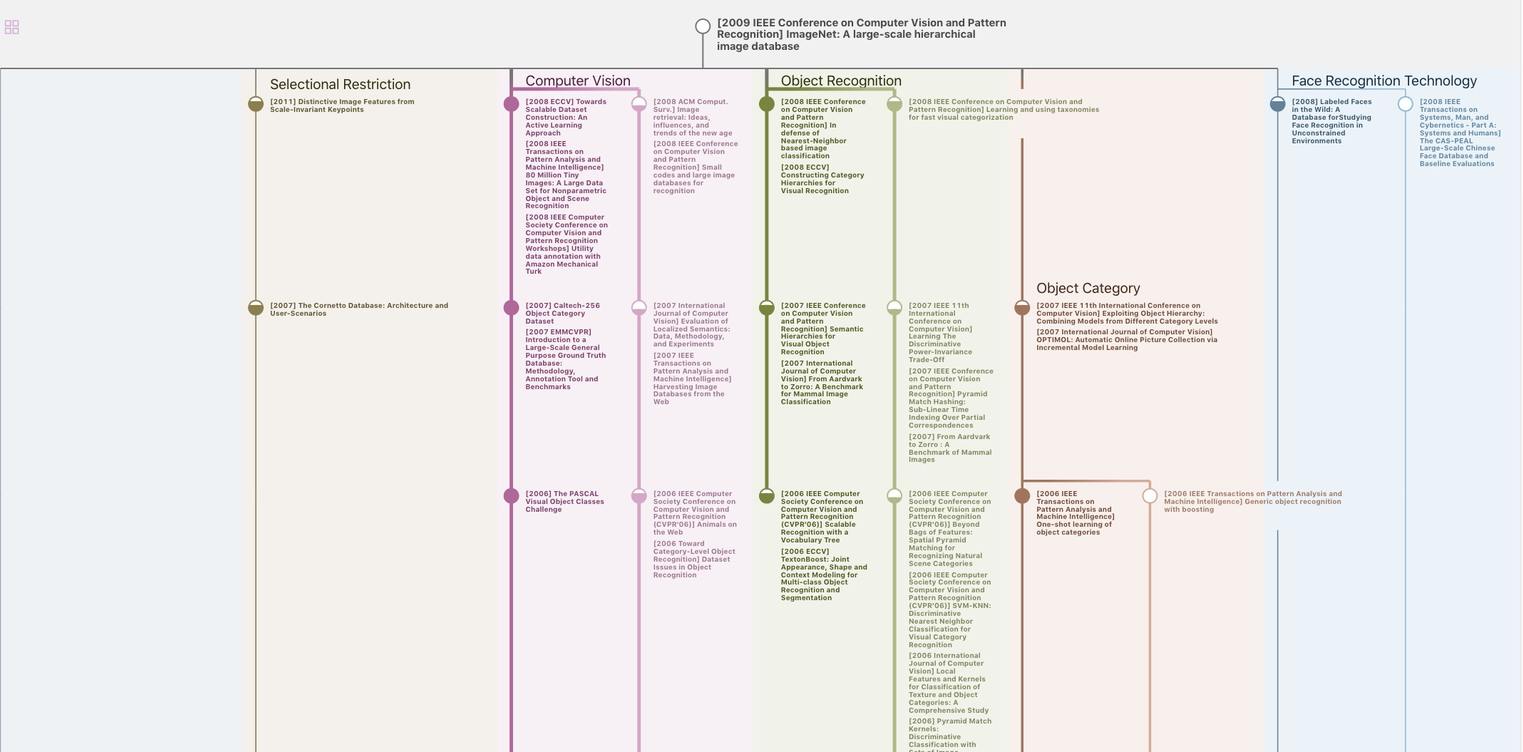
生成溯源树,研究论文发展脉络
Chat Paper
正在生成论文摘要