PPN-Pack: Placement Proposal Network for Efficient Robotic Bin Packing
IEEE ROBOTICS AND AUTOMATION LETTERS(2024)
摘要
Robotic bin packing is a challenging task, requiring compactly packing objects in a container and also efficiently performing the computation, such that the robot arm need not wait too long before taking action. In this work, we introduce PPN-Pack, a novel learning-based approach to improve the efficiency of packing general objects. Our key idea is to learn to predict good placement locations for compact object packing to prune the search space and reduce packing computation. Specifically, we formulate the learning of placement proposals as a ranking task and construct a ranking loss based on the Hinge loss to rank the potentially compact placements. To enhance the placement learning, we further design a multi-resolution cross-correlation module to better exploit the placement compactness between the container and objects. We perform extensive experiments on 2,000 packing cases. By equipping PPN-Pack to prune the proposals for the upper-bound packing heuristic, we can yield a remarkable 76% reduction in computation time, while achieving comparable packing compactness. Compared with the other packing heuristics, PPN-Pack reduces at least 47% computation time, while producing more compact packings.
更多查看译文
关键词
Manipulation planning,visual learning,integrated planning and learning
AI 理解论文
溯源树
样例
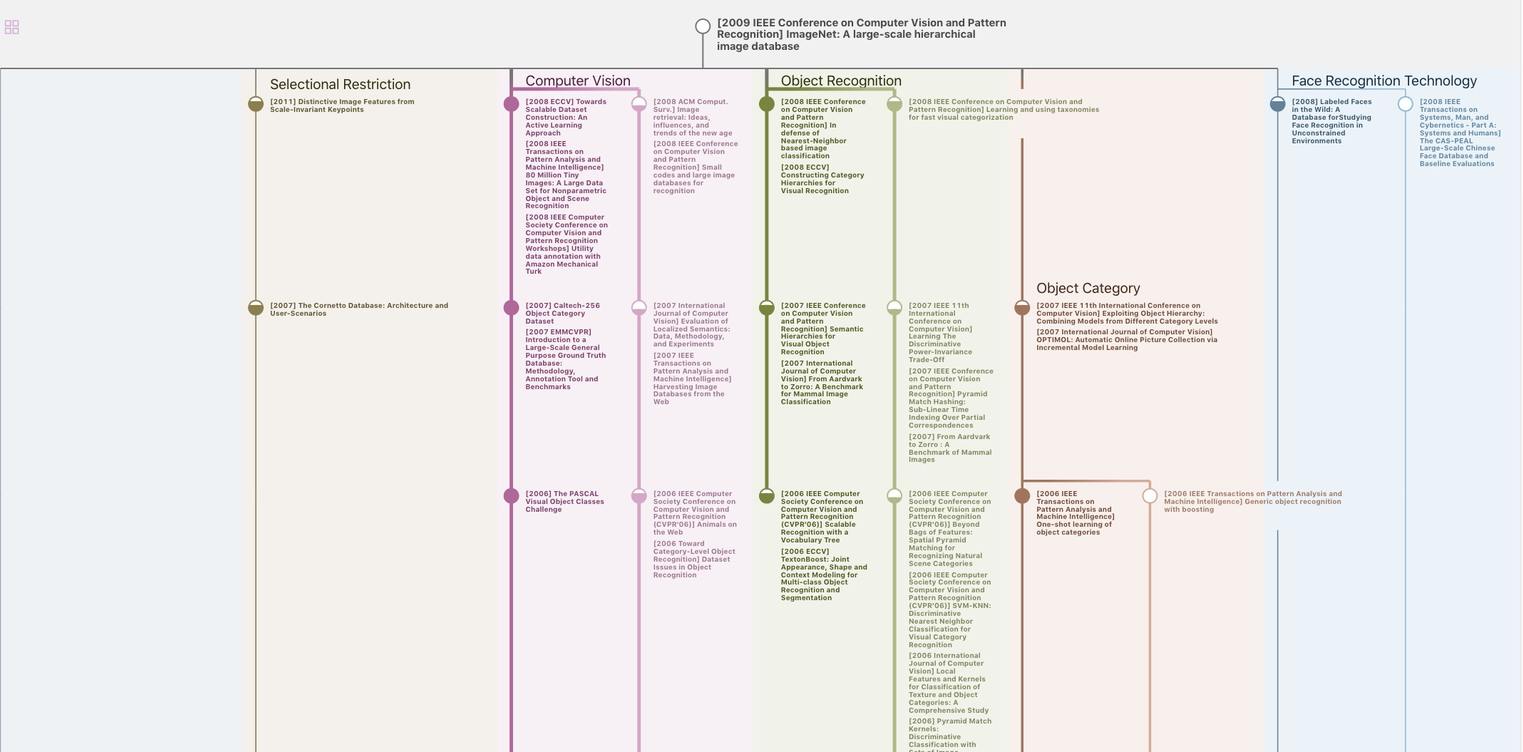
生成溯源树,研究论文发展脉络
Chat Paper
正在生成论文摘要