Advancing Road Infrastructure: an Integrated Approach for Accurate Pothole Detection and Mapping in Developing Countries
2023 IEEE Asia-Pacific Conference on Computer Science and Data Engineering (CSDE)(2023)
Key words
Pothole detection,YOLO,Road distress,Object Detection,Artificial Intelligence
AI Read Science
Must-Reading Tree
Example
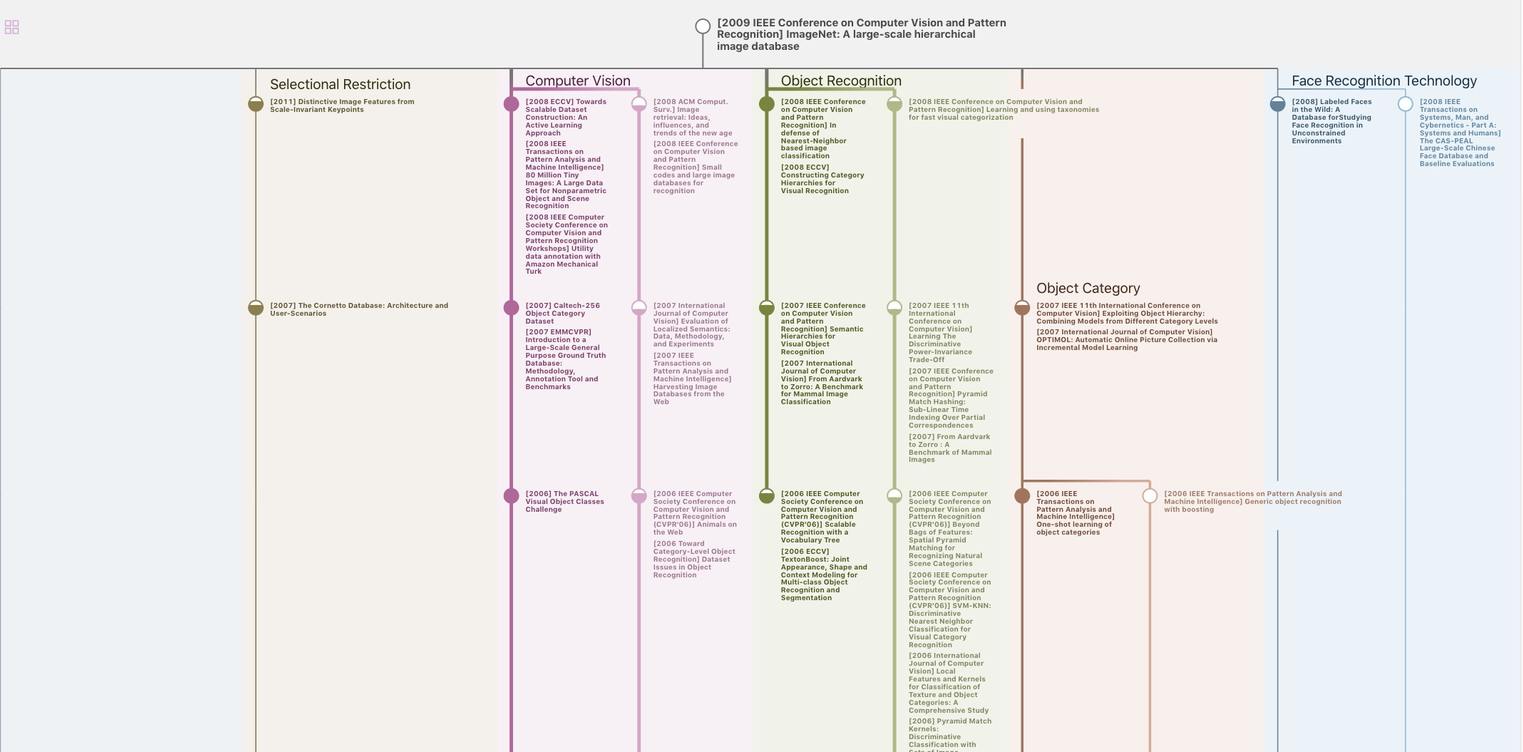
Generate MRT to find the research sequence of this paper
Chat Paper
Summary is being generated by the instructions you defined