Advancing Drug-Target interaction prediction with BERT and subsequence embedding
Computational Biology and Chemistry(2024)
Abstract
Exploring the relationship between proteins and drugs plays a significant role in discovering new synthetic drugs. The Drug-Target Interaction (DTI) prediction is a fundamental task in the relationship between proteins and drugs. Unlike encoding proteins by amino acids, we use amino acid subsequence to encode proteins, which simulates the biological process of DTI better. For this research purpose, we proposed a novel deep learning framework based on Bidirectional Encoder Representation from Transformers (BERT), which integrates high-frequency subsequence embedding and transfer learning methods to complete the DTI prediction task. As the first key module, subsequence embedding allows to explore the functional interaction units from drug and protein sequences and then contribute to finding DTI modules. As the second key module, transfer learning promotes the model learn the common DTI features from protein and drug sequences in a large dataset. Overall, the BERT-based model can learn two kinds features through the multi-head self-attention mechanism: internal features of sequence and interaction features of both proteins and drugs, respectively. Compared with other methods, BERT-based methods enable more DTI-related features to be discovered by means of attention scores which associated with tokenized protein/drug subsequences.We conducted extensive experiments for the DTI prediction task on three different benchmark datasets. The experimental results show that the model achieves an average prediction metrics higher than most baseline methods. In order to verify the importance of transfer learning, we conducted an ablation study on datasets, and the results show the superiority of transfer learning. In addition, we test the scalability of the model on the dataset in unseen drugs and proteins, and the results of the experiments show that it is acceptable in scalability.
MoreTranslated text
Key words
Drug-Target interaction,BERT,Transfer learning,Subsequence embedding,Deep learning
AI Read Science
Must-Reading Tree
Example
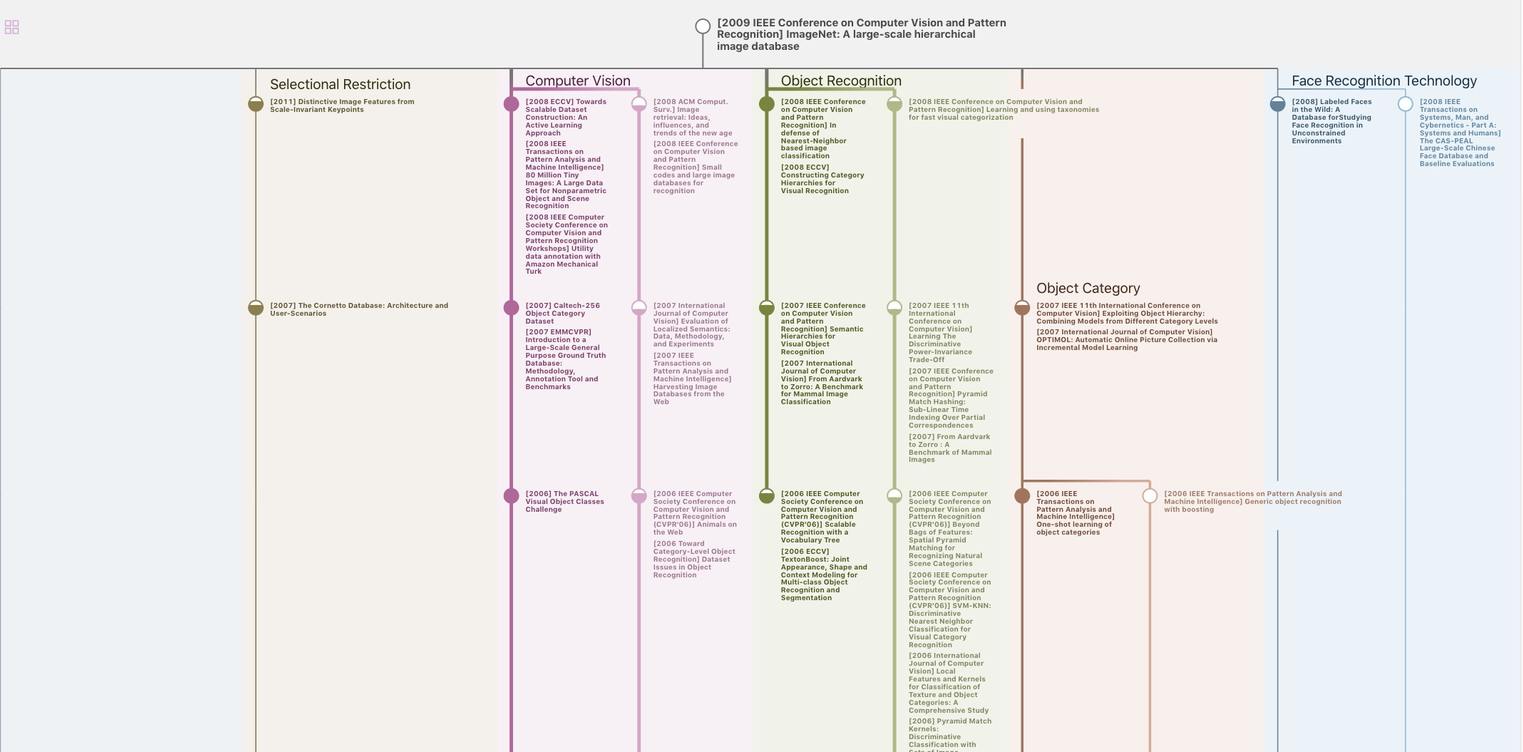
Generate MRT to find the research sequence of this paper
Chat Paper
Summary is being generated by the instructions you defined