Robust condition identification against label noise in industrial processes based on trusted connection dictionary learning
Reliability Engineering & System Safety(2024)
摘要
In the era of big data, the pervasive use of artificial intelligence (AI) technology has revolutionized various industries. AI-powered systems, particularly those utilizing data-driven interpretable and reliable methods for accurate condition identification, have emerged as a crucial factor in achieving intelligent industrial systems. However, in real industrial sites, the labels of offline data often contain incorrect labels, i.e. label noise, due to human error, sensor failure, and other sources, which reduces the accuracy of the model and affects the performance and safety of the industrial system. To tackle the challenge of precarious performance caused by label noise, this paper proposes a robust method called Trusted Connection Dictionary Learning (TCDL) which effectively mitigates the adverse effects of label noise through interpretable constraints and guarantees the trustworthiness of the model for industrial applications. Specifically, to address the issue of data structure and label inconsistency arising from label noise, this paper presents a dynamic graph model that jointly optimizes data features and label information to obtain consistent representations of them. Subsequently, to effectively fit the labeled data, TCDL leverages the label information from the nearest neighbors through the optimized graph structure and facilitates the self-correction of label noise, leading to robust representations of process features and a reliable condition classifier. Finally, a novel iterative optimization algorithm that combines the Block Coordinate Descent (BCD) method and the Alternating Direction Multiplier Method (ADMM) is also developed to ensure the convergence of the algorithm. Due to simple constraints, it can obtain accurately optimized variables with few iterations. Numerical simulations and industrial experiments demonstrate the superiority of the proposed method compared to some state-of-the-art methods.
更多查看译文
关键词
Condition identification,Label noise,Dictionary learning,Dynamic graph
AI 理解论文
溯源树
样例
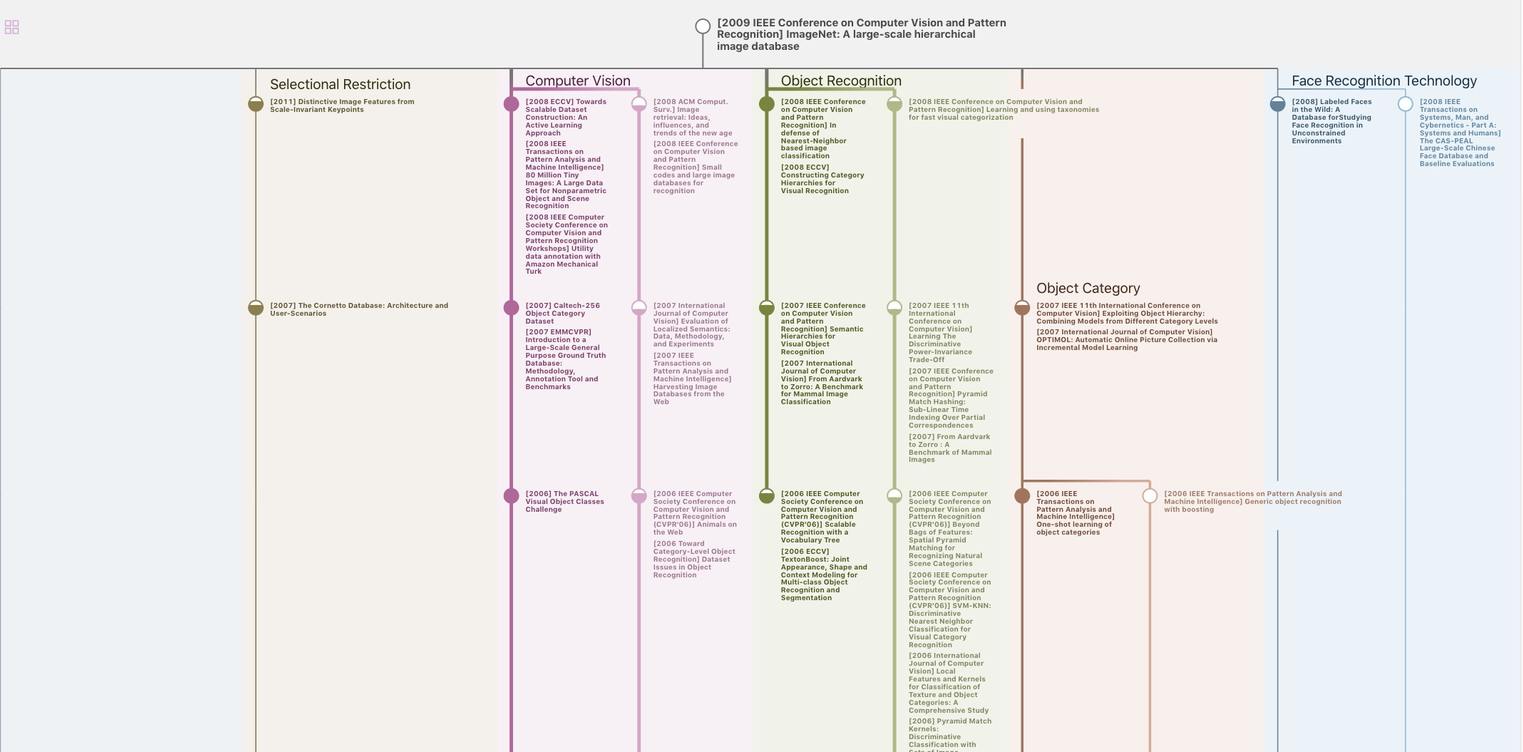
生成溯源树,研究论文发展脉络
Chat Paper
正在生成论文摘要