Analysis of the hyperparameter optimisation of four machine learning satellite imagery classification methods
Computational Geosciences(2024)
摘要
The classification of land use and land cover (LULC) from remotely sensed imagery in semi-arid Mediterranean areas is a challenging task due to the fragmentation of the landscape and the diversity of spatial patterns. Recently, the use of deep learning (DL) for image analysis has increased compared to commonly used machine learning (ML) methods. This paper compares the performance of four algorithms, Random Forest (RF), Support Vector Machine (SVM), Multilayer Perceptron (MLP) and Convolutional Network (CNN), using multi-source data, applying an exhaustive optimisation process of the hyperparameters. The usual approach in the optimisation process of a LULC classification model is to keep the best model in terms of accuracy without analysing the rest of the results. In this study, we have analysed such results, discovering noteworthy patterns in a space defined by the mean and standard deviation of the validation accuracy estimated in a 10-fold cross validation (CV). The point distributions in such a space do not appear to be completely random, but show clusters of points that facilitate the discovery of hyperparameter values that tend to increase the mean accuracy and decrease its standard deviation. RF is not the most accurate model, but it is the less sensitive to changes in hyperparameters. Neural Networks, tend to increase commission and omission errors of the less represented classes because their optimisation lead the model to learn better the most frequent classes. On the other hand, RF and MLP prediction layers are the most accurate from a general qualitative point of view.
更多查看译文
关键词
Machine learning,LULC,Convolutional neuronal networks,Random forest,Support vector machines,Hyperparameter optimisation,62P12,62P25,91D20,68T07
AI 理解论文
溯源树
样例
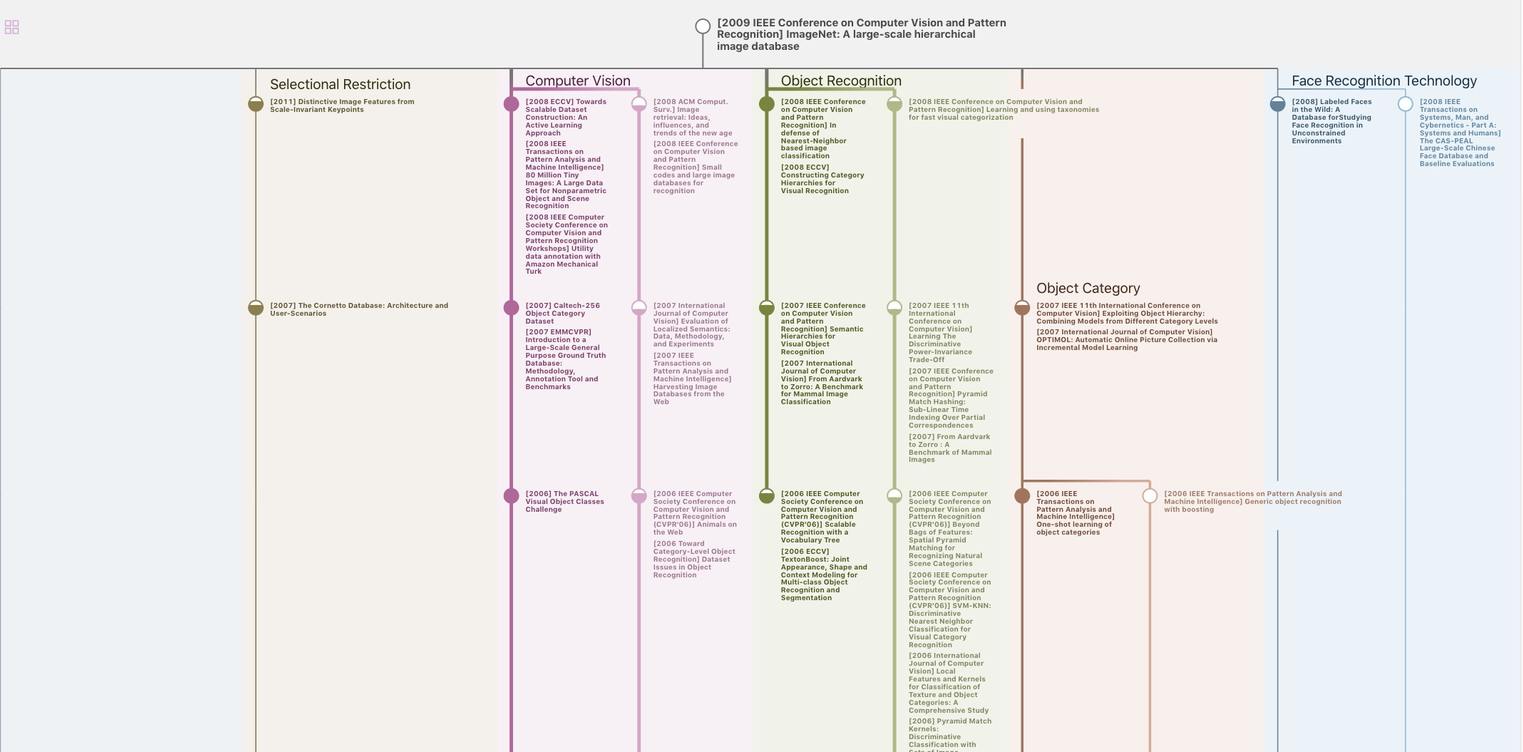
生成溯源树,研究论文发展脉络
Chat Paper
正在生成论文摘要