Differentiating Periodic Drivers of Air Quality Changes: A Two-Step Decomposition Approach Integrating Machine Learning and Wavelet Analysis
JOURNAL OF GEOPHYSICAL RESEARCH-ATMOSPHERES(2024)
摘要
Air quality time series exhibit significant periodic patterns, which are linked to a diverse array of emission sources and atmospheric processes. To discern and distinguish these periodic drivers, we have devised a two-step decomposition approach that integrates a machine learning-based model for weather normalization with Morlet wavelet analysis. This approach was applied to a 7-year data set encompassing six regulated air pollutants across eight Chinese cities. Our analysis revealed distinct periodicities in weather-normalized concentrations of primary air pollutants: a dominant annual cycle with periodicity around 365 days, which accounts for over 50% of the variance on average and is primarily driven by recurrent winter heating activities; daily cycles characterized by regular diurnal patterns attributable to combustion sources such as traffic; and periodicities exceeding 512 days that associated with long-term regulatory policies targeting SO2. Particularly notable was the significant drop in the strength of the annual cycle in northern cities following the implementation of China's clean heating policies in 2017/2018, affirming the success of these initiatives. Additionally, diurnal dispersion and photochemistry, large-scale atmospheric circulation, and synoptical weather patterns are likely responsible for the observed daily cycle (accounting for over 40% of the variance), annual periodicities, and intra-monthly variations in the meteorologically driven concentrations, respectively. The varying power of these periodic drivers across time and locations implies the heterogeneity of emission rates and region-specific climates. This work highlights the efficacy of this decomposition approach in air quality research, warranting attention for its potential value for enhancing our understanding of air pollution dynamics. Periodic patterns can be widely observed in Earth's environment, particularly in the atmosphere, lead to repeating fluctuations in air pollution that correspond to regular time intervals. These changes are driven by a combination of emissions from various sources and weather patterns. Understanding what causes air quality changes is crucial for effective intervention. This study introduces a refined method to analyze air pollution, using machine learning to distinguish between the effects of human activities and natural weather variations on pollution levels. Additionally, Morlet wavelet analysis is used to identify the specific periodic forces driving these changes. Tested on several years of air quality data from eight Chinese cities, the method effectively pinpointed recurring pollution patterns linked to both emissions and weather on different time scales. The study reveals varying impacts of these patterns by region and time, due to differences in emission rates and region-specific climates. The proposed approach is expected to enhance our understanding of air pollution dynamics and inform policy decisions for better air quality management. Drivers of air quality variation can be differentiated and interpreted based on their periodic patterns and powers Yearly recurring winter heating-related activities account for the most significant variation in primary air pollutants in northern Chinese cities The varying power of periodic drivers over time and locations emphasizes the heterogeneity of emission rates and region-specific climates
更多查看译文
AI 理解论文
溯源树
样例
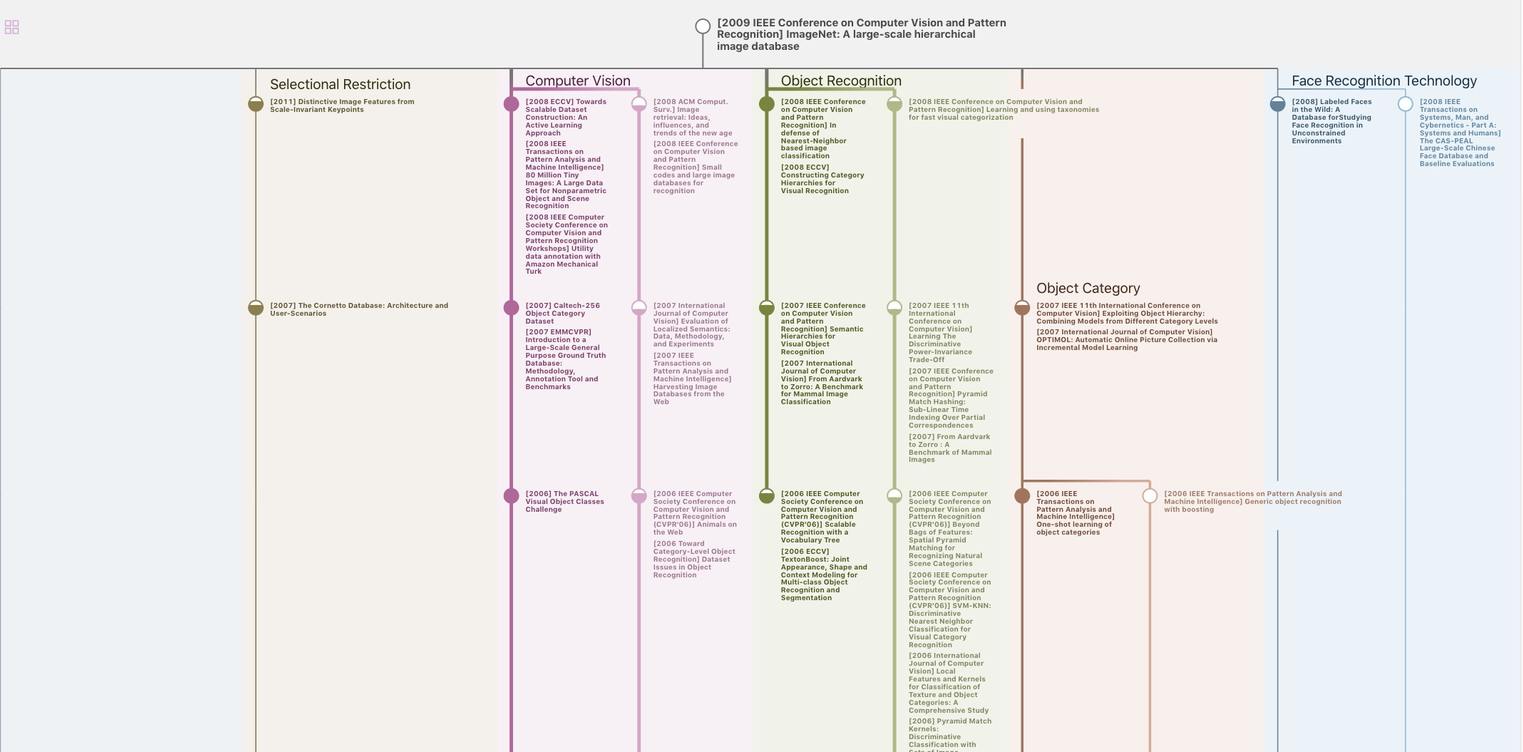
生成溯源树,研究论文发展脉络
Chat Paper
正在生成论文摘要