A Novel Temporal Privacy-Preserving Model for Social Recommendation
IEEE TRANSACTIONS ON COMPUTATIONAL SOCIAL SYSTEMS(2024)
摘要
Social recommendation improved the quality and efficiency of recommendation but increased the risk of privacy leakage, especially with the introduction of social networks. Consequently, the social recommendation considering user privacy has drawn tremendous attention from academia to industry. Nevertheless, most of the existing work regards the recommender systems as static, ignoring the diffusion of social influence over time. In this article, we propose a secure and efficient framework, temporal privacy-preserving social recommendation model (PrivTSR), to capture the changes of user preference for items and item types with time. PrivTSR first utilizes differential privacy to encrypt the data owned by the data owner. Then, inspired by the long short-term memory (LSTM), at each time step the initial user embedding and the initial item embedding are generated via DeepWalk as new ratings of users for items emerges in the user-item-type graph. The initial user-preference embedding is generated randomly at the first time step, and it is equivalent to the updated embedding of the previous time step for the later time steps. Most importantly, on the social graph, PrivTSR updates the user embedding and the user-preference embedding with graph attention convolutional network and graph attention diffused network, which aggregates (diffuses) social influence from (to) neighbors in depth and breadth. On the user-item-type graph, the user embedding and the item embedding are updated by aggregating the embedding of users and items in the six paths. Final, taking into account the users' preference for items and item types, PrivTSR predicts the ratings of users to the items for the next time step. The extensive experiments are conducted on two real-world datasets, which demonstrated the superiority of our model over several competitive baselines.
更多查看译文
关键词
Differential privacy,influence diffusion,network embedding,social recommendation,time series contexts
AI 理解论文
溯源树
样例
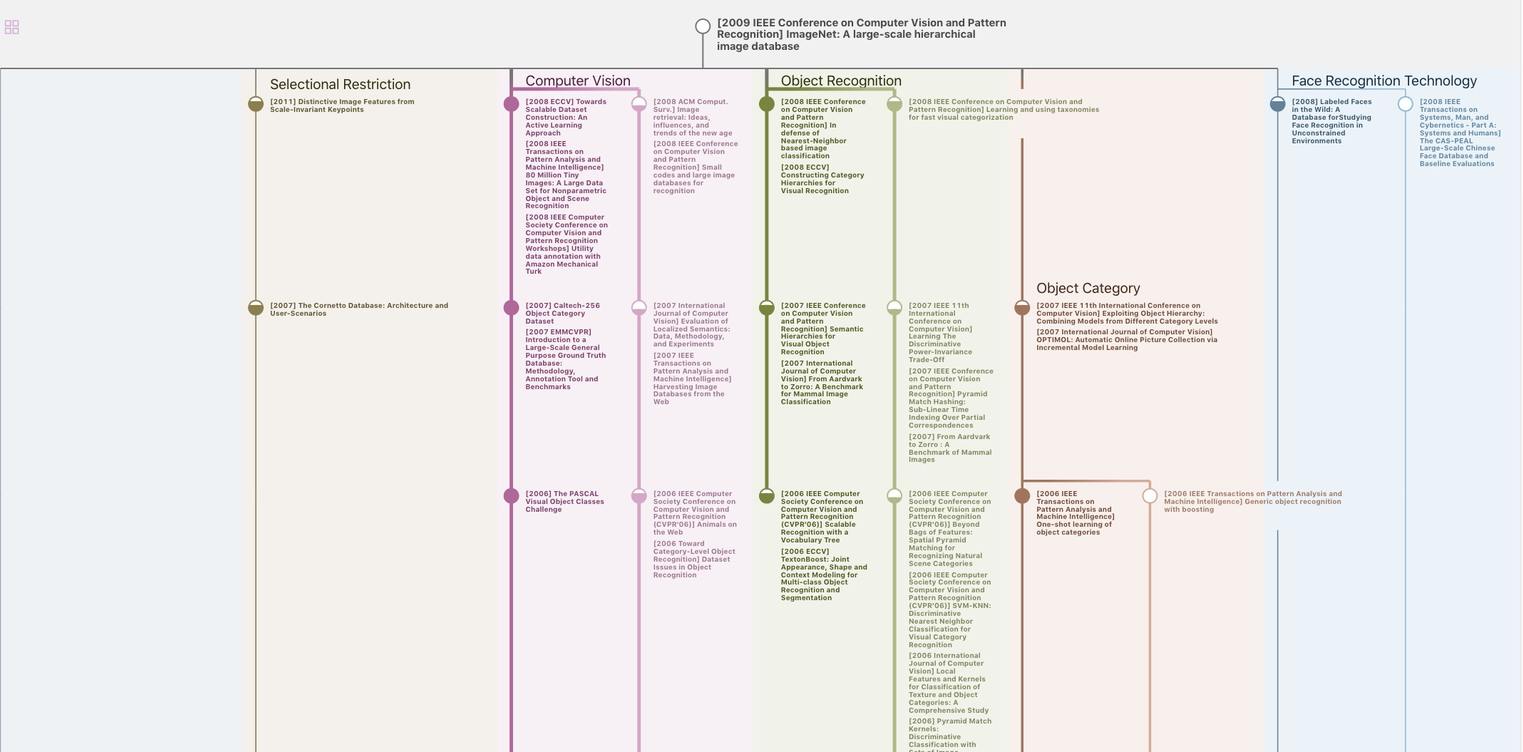
生成溯源树,研究论文发展脉络
Chat Paper
正在生成论文摘要