Filling gaps in cloudy Landsat LST product by spatial-temporal fusion of multi-scale data
Remote Sensing of Environment(2024)
摘要
Land surface temperature (LST) is an important factor in studies of surface energy fluxes between the Earth's surface and atmosphere. The Landsat LST product has been applied widely due to its fine spatial resolution and high data quality. Frequent cloud coverage, however, results in different degrees of gaps in the Landsat LST images, restricting greatly their application in practical cases requiring continuous LST time-series. Gap filling is an important technique used to reconstruct the missing data in the Landsat LST images. Using only temporally neighboring data from the same sensor, however, current gap filling methods struggle to deal with large spatial gaps and large temporal changes in LST. To solve these challenging issues, in this paper, multi-scale data (i.e., the 2 km, all-sky, hourly GOES LST product) were proposed for gap filling of Landsat LST images, and a spatial-temporal random forest (STRF) method was developed. The GOES LST product can provide effective information on LST in the gaps of the target Landsat LST data, with great potential to decreasing the uncertainty in gap filling when large LST change exists or the gap size is large. Through experiments in six regions of the USA, the proposed STRF method was shown to be more accurate than three typical gap filling and spatio-temporal fusion methods, and particularly advantageous for cases involving large temporal changes or large spatial gaps in LST. Specifically, STRF produces an average root mean square error (RMSE) of 1.6444 K and average correlation coefficient (CC) of 0.8653 for the six regions, which are 0.1133 K smaller and 0.0176 larger than the most competitive benchmark method (i.e., the spatial random forest (SRF) method). Conceptually, by spatial-temporal fusion of multi-scale data in gap filling, STRF provides a new paradigm for both spatial-temporal fusion and gap filling. Furthermore, the proposed STRF has the potential to be applied to various gap filling tasks in other situations.
更多查看译文
关键词
Land surface temperature (LST),Gap filling,Spatio-temporal fusion,Landsat,GOES
AI 理解论文
溯源树
样例
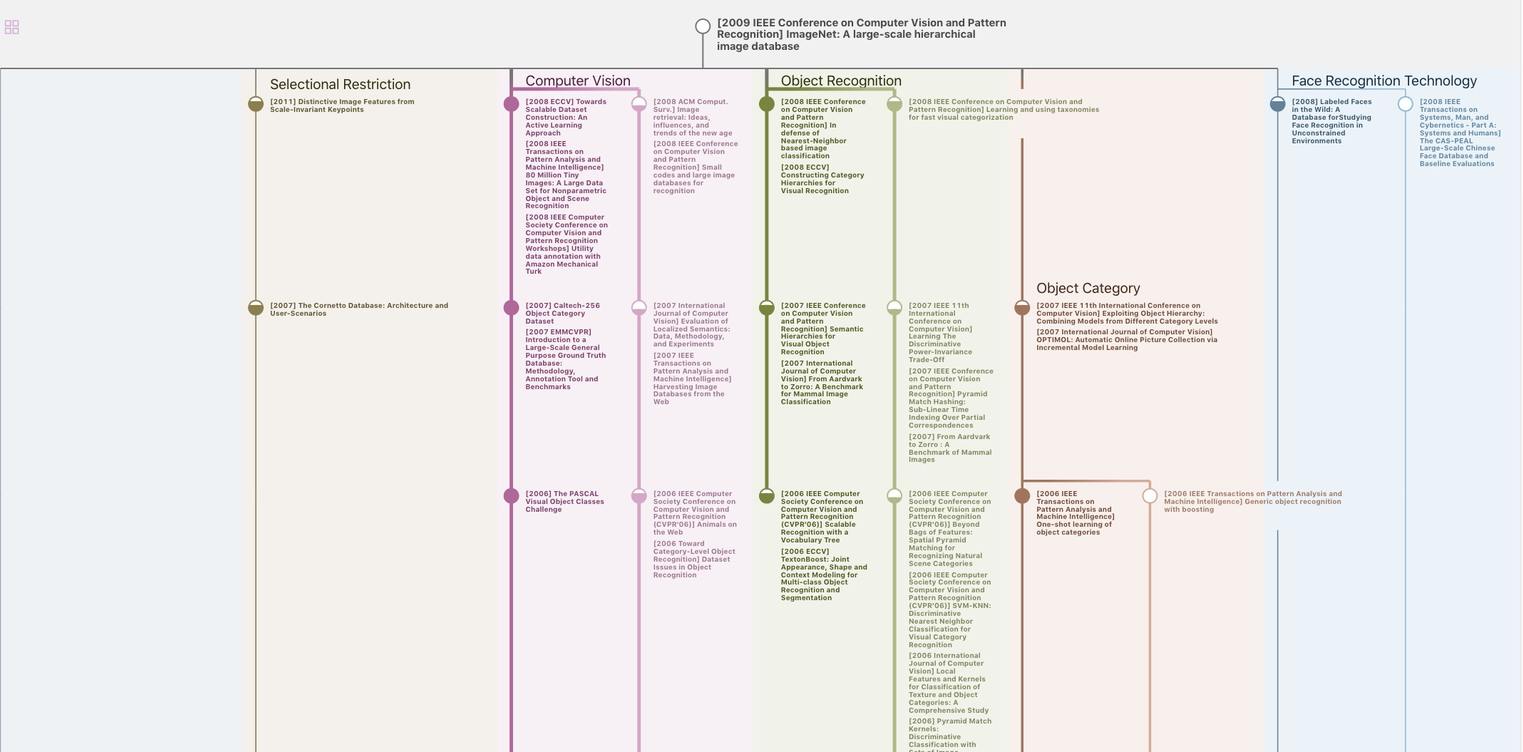
生成溯源树,研究论文发展脉络
Chat Paper
正在生成论文摘要