WeldNet: a lightweight deep learning model for welding defect recognition
Welding in the World(2024)
摘要
Weld defect detection is an important task in the welding process. Although there are many excellent weld defect detection models, there is still much room for improvement in stability and accuracy. In this study, a lightweight deep learning model called WeldNet is proposed to improve the existing weld defect recognition network for its poor generalization performance, overfitting, and large memory occupation, using a design with a small number of parameters but with better performance. We also proposed an ensemble-distillation strategy in the training process, which effectively improved the accuracy rate and proposed an improved model ensemble scheme. The experimental results show that the final designed WeldNet model performs well in detecting weld defects and achieves state-of-the-art performance. Its number of parameters is only 26.8 https://github.com/Wanglaoban3/WeldNet.git .
更多查看译文
关键词
Convolutional neural networks,Weld defect,Model ensemble,Knowledge distillation
AI 理解论文
溯源树
样例
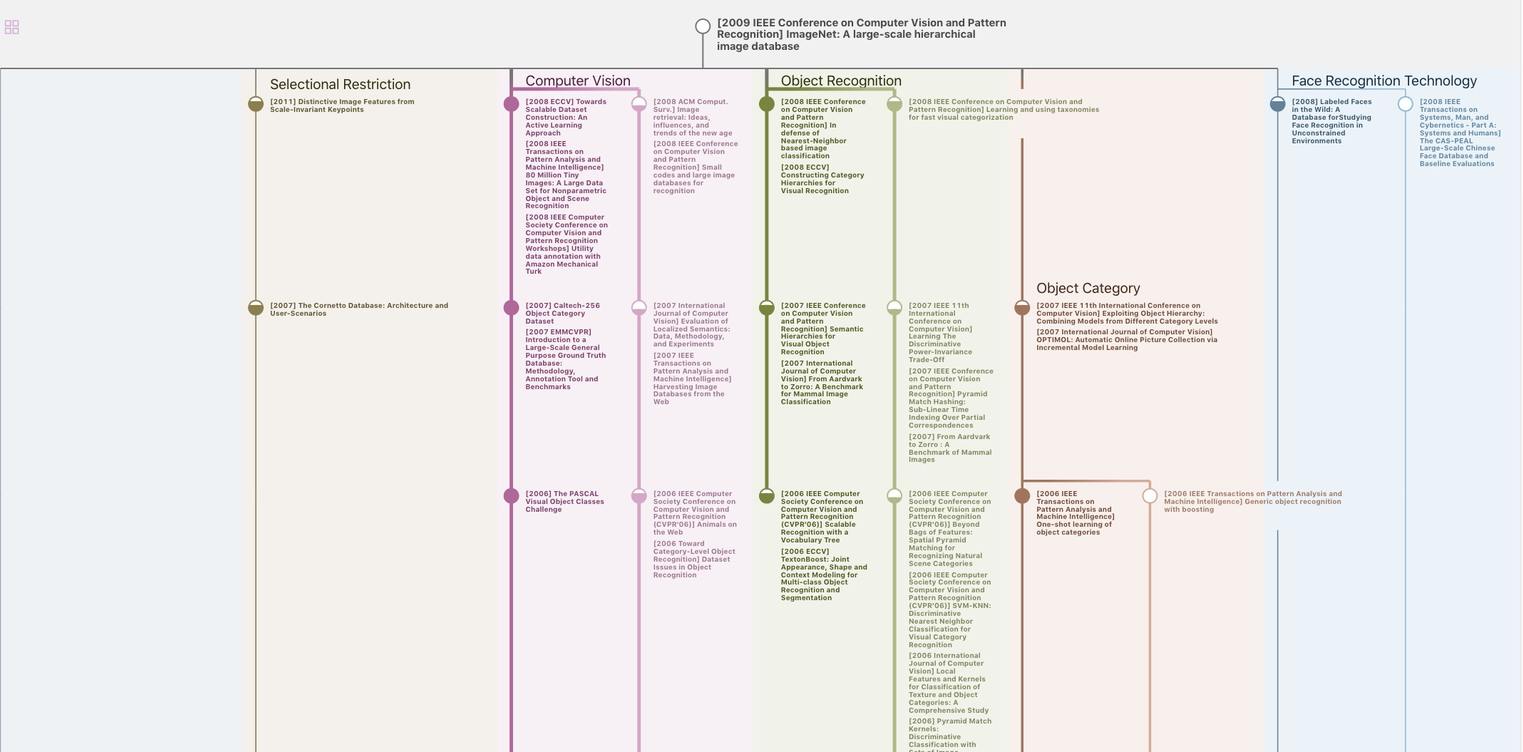
生成溯源树,研究论文发展脉络
Chat Paper
正在生成论文摘要