Data-driven classification of cognitively normal and mild cognitive impairment subtypes predicts progression in the NACC dataset.
Alzheimer's & dementia : the journal of the Alzheimer's Association(2024)
摘要
INTRODUCTION:Data-driven neuropsychological methods can identify mild cognitive impairment (MCI) subtypes with stronger associations to dementia risk factors than conventional diagnostic methods.
METHODS:Cluster analysis used neuropsychological data from participants without dementia (mean age = 71.6 years) in the National Alzheimer's Coordinating Center (NACC) Uniform Data Set (n = 26,255) and the "normal cognition" subsample (n = 16,005). Survival analyses examined MCI or dementia progression.
RESULTS:Five clusters were identified: "Optimal" cognitively normal (oCN; 13.2%), "Typical" CN (tCN; 28.0%), Amnestic MCI (aMCI; 25.3%), Mixed MCI-Mild (mMCI-Mild; 20.4%), and Mixed MCI-Severe (mMCI-Severe; 13.0%). Progression to dementia differed across clusters (oCN < tCN < aMCI < mMCI-Mild < mMCI-Severe). Cluster analysis identified more MCI cases than consensus diagnosis. In the "normal cognition" subsample, five clusters emerged: High-All Domains (High-All; 16.7%), Low-Attention/Working Memory (Low-WM; 22.1%), Low-Memory (36.3%), Amnestic MCI (16.7%), and Non-amnestic MCI (naMCI; 8.3%), with differing progression rates (High-All < Low-WM = Low-Memory < aMCI < naMCI).
DISCUSSION:Our data-driven methods outperformed consensus diagnosis by providing more precise information about progression risk and revealing heterogeneity in cognition and progression risk within the NACC "normal cognition" group.
更多查看译文
AI 理解论文
溯源树
样例
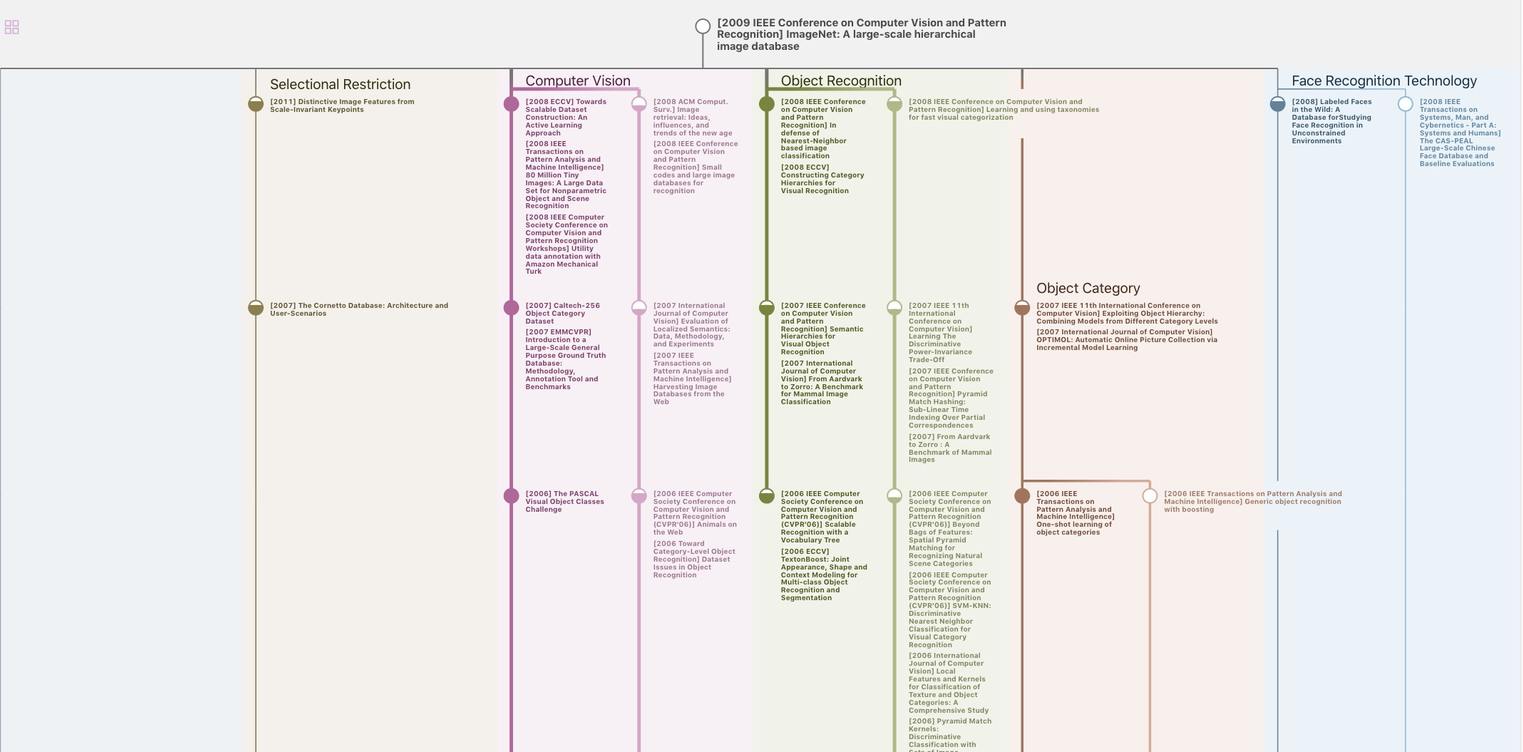
生成溯源树,研究论文发展脉络
Chat Paper
正在生成论文摘要