Ensemble Forecasts of Extreme Flood Events with Weather Forecasts, Land Surface Modeling and Deep Learning
WATER(2024)
摘要
Integrating numerical weather forecasts that provide ensemble precipitation forecasts, land surface hydrological modeling that resolves surface and subsurface hydrological processes, and artificial intelligence techniques that correct the forecast bias, known as the "meteo-hydro-AI" approach, has emerged as a popular flood forecast method. However, its performance during extreme flood events across different interval basins has received less attention. Here, we evaluated the meteo-hydro-AI approach for forecasting extreme flood events from headwater to downstream sub-basins in the Luo River basin during 2010-2017, with forecast lead times up to 7 days. The proposed meteo-hydro approach based on ECMWF weather forecasts and the Conjunctive Surface-Subsurface Process version 2 land surface model with a spatial resolution of 1 km captured the flood hydrographs quite well. Compared with the ensemble streamflow prediction (ESP) approach based on initial conditions, the meteo-hydro approach increased the Nash-Sutcliffe efficiency of streamflow forecasts at the three outlet stations by 0.27-0.82, decreased the root-mean-squared-error by 22-49%, and performed better in reliability and discrimination. The meteo-hydro-AI approach showed marginal improvement, which suggested further evaluations with larger samples of extreme flood events should be carried out. This study demonstrated the potential of the integrated meteo-hydro-AI approach for ensemble forecasting of extreme flood events.
更多查看译文
关键词
flood,forecast,AI,land surface model
AI 理解论文
溯源树
样例
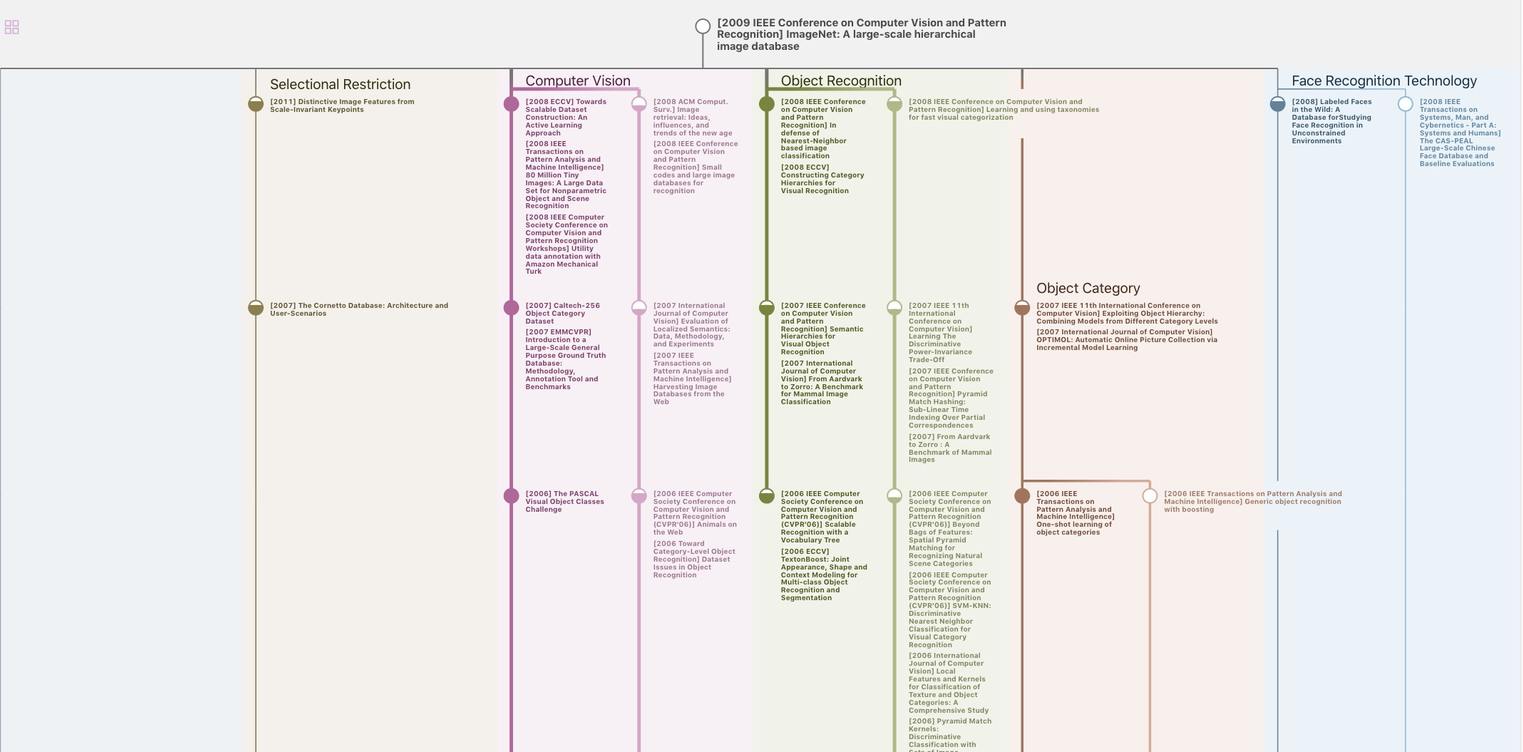
生成溯源树,研究论文发展脉络
Chat Paper
正在生成论文摘要