A New Dual-Domain Signal Collaborative Transfer Network for Bearing Fault Diagnosis.
IEEE Trans. Instrum. Meas.(2024)
摘要
Contemporary methods for fault diagnosis typically employ frequency-domain signals as inputs. However, the effectiveness of those methods can be undermined by the presence of "frequency modulation" and "amplitude modulation", particularly when the equipment is subject to fluctuating speed conditions. As a result, the diagnostic accuracy of those methods is significantly compromised. To tackle this problem, a dual-domain signal collaborative transfer network (DSCTN) is proposed in this paper for the diagnosis of cross-domain bearing faults under fluctuating speed conditions. Firstly, a multi-level fusion feature extraction module is designed based on Swin Transformer and dual-domain signals. It is capable of extracting representative features from time and frequency domain signals and mitigating the impact of speed fluctuation. Moreover, an adversarial training strategy and the Wasserstein distance are jointly leveraged to facilitate domain alignment. Experimental results of bearing fault diagnosis show that the DSCTN approach can reach higher accuracy and achieve superior domain-invariant feature extraction capability compared to existing methods.
更多查看译文
关键词
Bearing fault diagnosis,dual-domain signals collaborative diagnosis,fluctuating speed,transfer learning
AI 理解论文
溯源树
样例
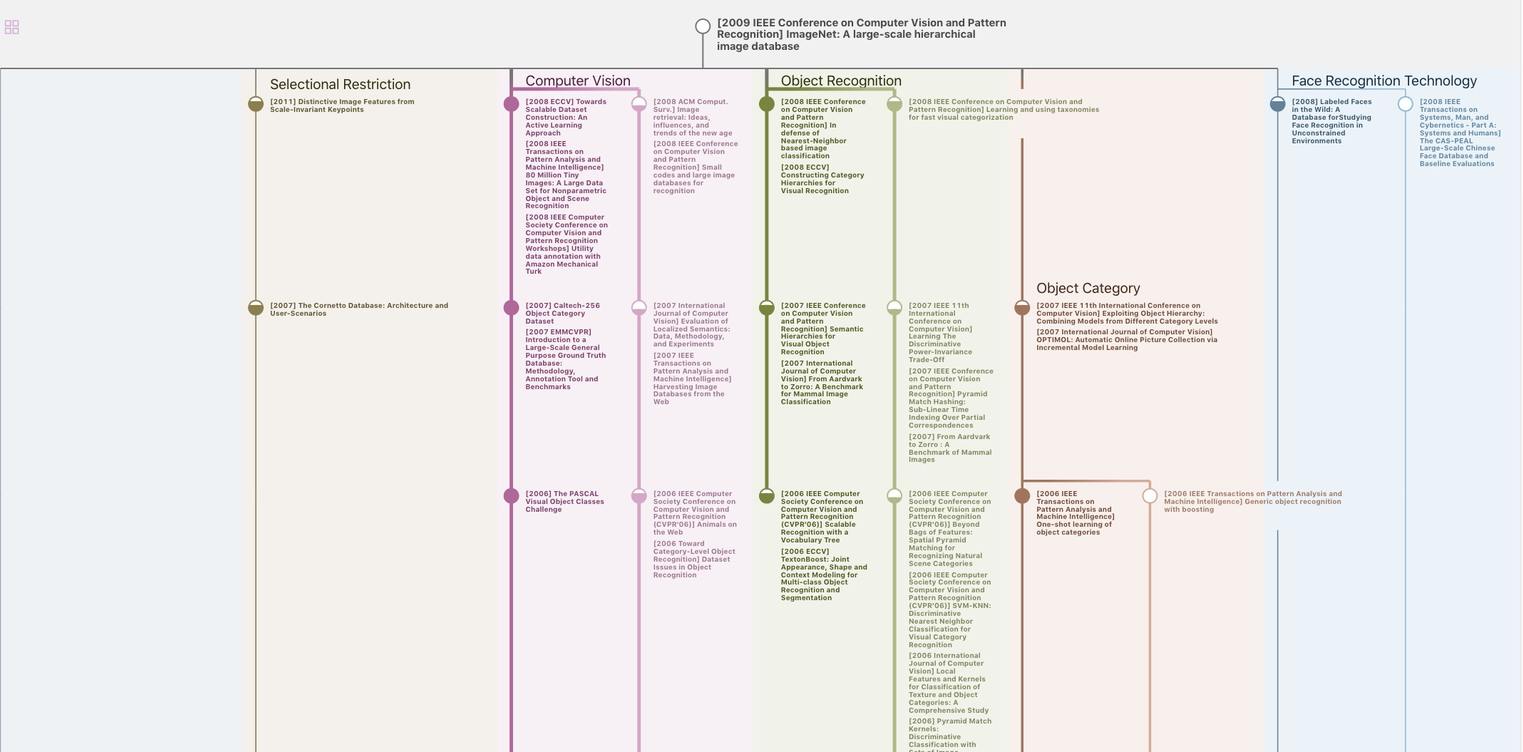
生成溯源树,研究论文发展脉络
Chat Paper
正在生成论文摘要