Efficient Bayesian CNN Model Compression using Bayes by Backprop and L1-Norm Regularization
Neural Processing Letters(2024)
摘要
The swift advancement of convolutional neural networks (CNNs) in numerous real-world utilizations urges an elevation in computational cost along with the size of the model. In this context, many researchers steered their focus to eradicate these specific issues by compressing the original CNN models by pruning weights and filters, respectively. As filter pruning has an upper hand over the weight pruning method because filter pruning methods don’t impact sparse connectivity patterns. In this work, we suggested a Bayesian Convolutional Neural Network (BayesCNN) with Variational Inference, which prefaces probability distribution over weights. For the pruning task of Bayesian CNN, we utilized a combined version of L1-norm with capped L1-norm to help epitomize the amount of information that can be extracted through filter and control regularization. In this formation, we pruned unimportant filters directly without any test accuracy loss and achieved a slimmer model with comparative accuracy. The whole process of pruning is iterative and to validate the performance of our proposed work, we utilized several different CNN architectures on the standard classification dataset available. We have compared our results with non-Bayesian CNN models particularly, datasets such as CIFAR-10 on VGG-16, and pruned 75.8
更多查看译文
关键词
Bayesian CNNs,Bayes by backprop,Capped L1-norm,L1-norm regularization,Model compression,Uncertainty estimation
AI 理解论文
溯源树
样例
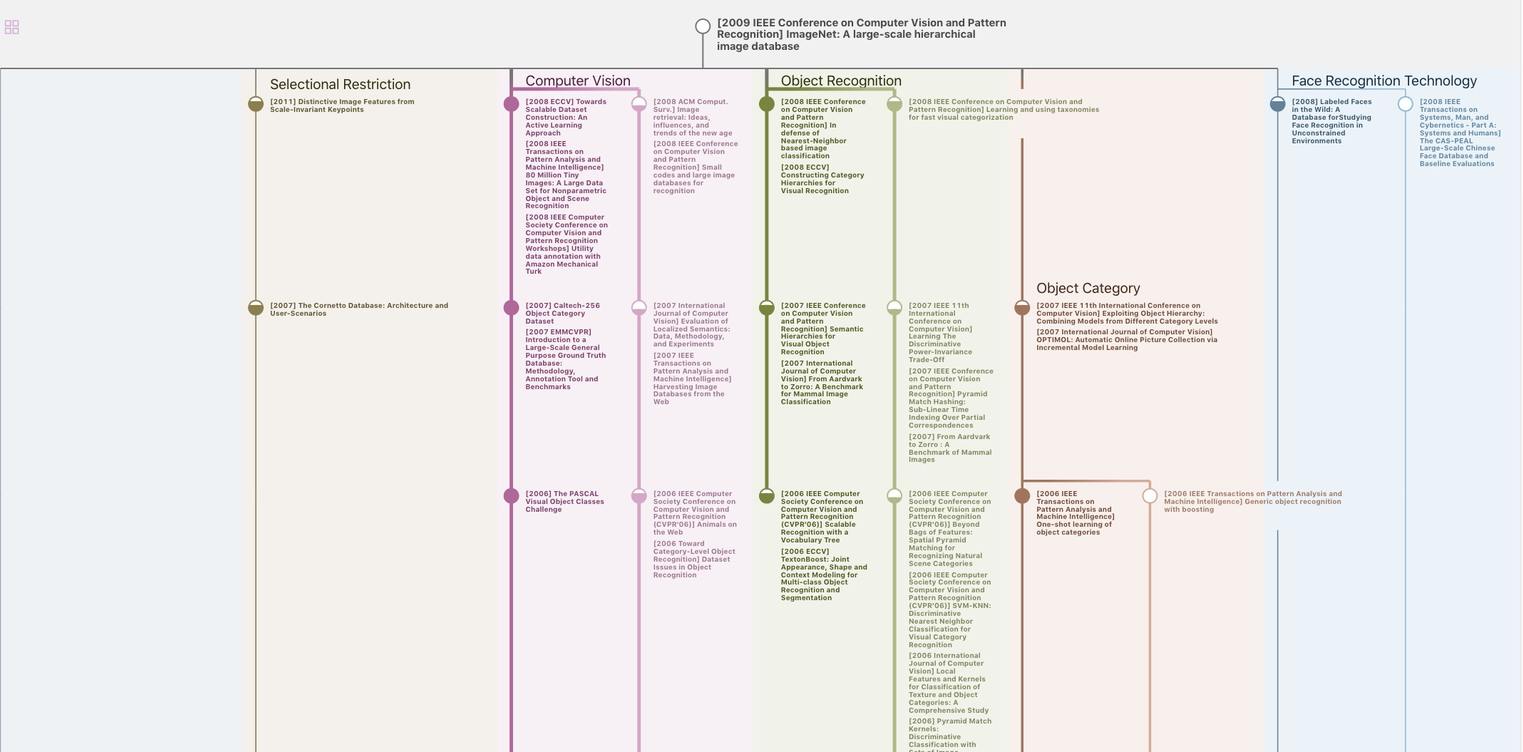
生成溯源树,研究论文发展脉络
Chat Paper
正在生成论文摘要