A Mixed-Criticality Traffic Scheduler with Mitigating Congestion for CAN-to-TSN Gateway
ACM Transactions on Design Automation of Electronic Systems(2024)
摘要
The network architecture that Time-Sensitive Networking (TSN) is used as the backbone network and the Controller Area Network (CAN) serves as the intra-domain network is considered as the CAN-TSN interconnection network architecture, which has gained considerable attention within industrial embedded networks, such as spacecraft, intelligent automobiles, and factory automation. The architecture employs the CAN-TSN gateway as a central hub for transmitting and managing a significant volume of communications between the CAN domains and TSN. However, the CAN-TSN gateway faces a high congestion challenge due to the rapid growth in data volume, making it difficult to effectively support different time planning mechanisms provided by TSN. In this paper, we propose a two-stage mixed-criticality traffic scheduler. The scheduler in the first stage adopts a Message Optimization Algorithm (MOA) to aggregate multiple CAN messages into a single TSN message (including the aggregation of critical and non-critical CAN messages), which reduces the number of CAN messages requiring transmission. In the second stage, the scheduler proposes a Message Scheduling Optimization Algorithm (MSOA) to schedule critical TSN messages. This algorithm reassembles all the critical CAN messages (within the un-schedulable TSN messages) to generate new TSN messages for rescheduling. Experimental results show that our proposed scheduler effectively improves the acceptance ratio of critical and non-critical CAN messages and outperforms the state-of-the-art message scheduling method in terms of acceptance ratio while improving the bandwidth utilization and the number of schedule table entries. We further construct a hardware platform to evaluate the performance of MSOA. The consistency between practical results and theoretical results shows the effectiveness of MSOA.
更多查看译文
AI 理解论文
溯源树
样例
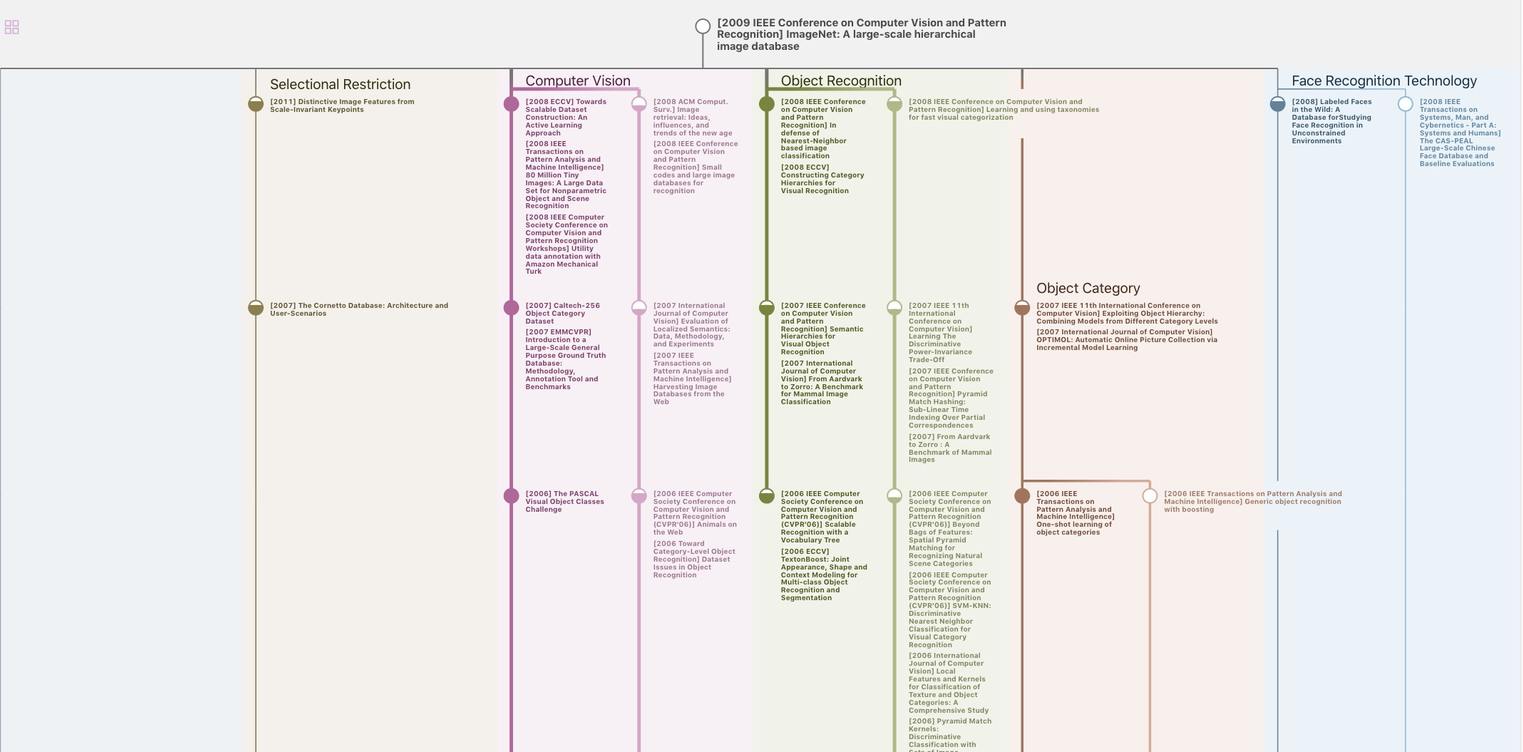
生成溯源树,研究论文发展脉络
Chat Paper
正在生成论文摘要