Community anomaly detection in attribute networks based on refining context
Computing(2024)
Abstract
With the widespread use of attribute networks, anomalous node detection on attribute networks has received increasing attention. By utilizing communities as reference contexts for local anomaly node detection, it is possible to uncover a multitude of significant anomalous nodes. However, most of the current methods that use communities as reference context of anomalous nodes usually do not consider the accuracy of the reference context. The rough classification results obtained from community detection are used as reference contexts for anomalous node detection. The possibility of errors occurring in the reference context may subsequently result in detection errors for anomalous nodes. Based on this, we propose an integrated framework named ADRC (Anomaly Detection in attribute networks based on Refining Context) to simultaneously perform anomalous node detection and detailed adjustment of reference contexts. Meanwhile, to better reflect the anomaly degree of the nodes, we design an evaluation metric and rank the anomalous nodes by it. Comparisons are made with state-of-the-art algorithms on publicly available datasets and the results show that our approach has significant advantages.
MoreTranslated text
Key words
Anomalous node detection,Reference context,Community,Attribute networks,68T09
AI Read Science
Must-Reading Tree
Example
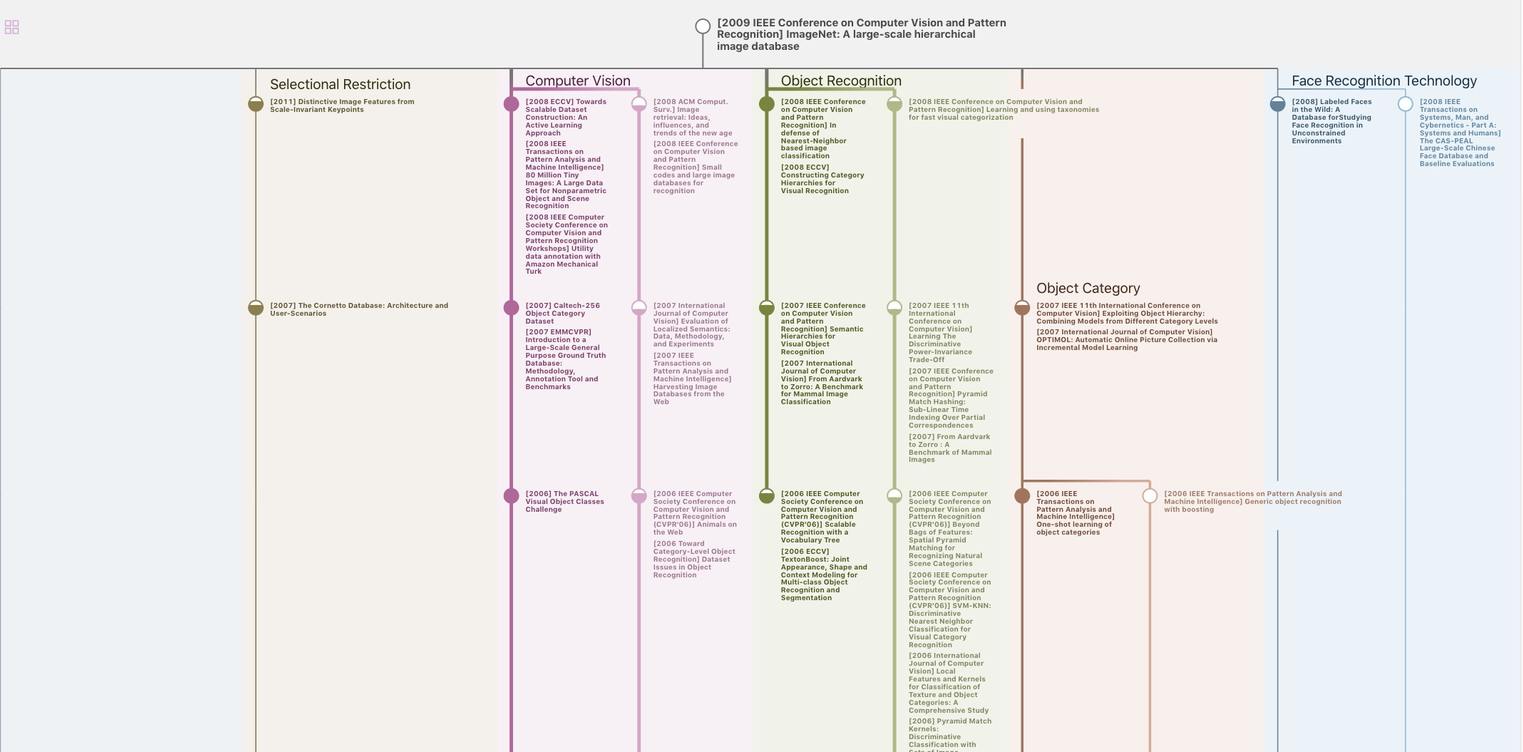
Generate MRT to find the research sequence of this paper
Chat Paper
Summary is being generated by the instructions you defined