A classification and regression assisted optimization algorithm for high-dimensional expensive many-objective problems
Neurocomputing(2024)
摘要
The expensive optimization problem, widespread in real-world applications, poses great challenges to traditional evolutionary algorithms (EAs) due to its costly evaluations. A lot of investigations have proved that it is a practical way to incorporate surrogate models into EAs to reduce the number of time-consuming evaluations. Although various types of surrogate models from the community of machine learning, such as regression models that approximate the fitness values of candidate solutions or classification models that predict candidate solutions’ category, have been widely applied separately, none of them consistently outperforms the other. Considering the intrinsic consistency of alleviating computational burden to improve the optimization efficiency, this paper introduces a two-layer architecture to integrate regression and classification models and synergize global and local evolution for high-dimensional expensive many-objective optimization problem (EMaOP). To implement this idea, the Kriging model based on incremental learning is utilized to explore the potential promising space. Further, multi local support vector machine (SVM) classifiers assisted improved differential evolution is run by turn with the global search to accelerate convergence. Accordingly, a novel surrogate-assisted EA, named Classification- and Regression-assisted evolutionary algorithm (CR-SAEA), is proposed on this basis. Experimental studies on several benchmark functions with up to 11 objectives and 200 dimensions confirm the effectiveness of the proposed algorithm and its competitiveness against state-of-the-art algorithms.
更多查看译文
关键词
Classification- and regression-based surrogate,Surrogate-assisted bilevel evolutionary algorithm,High-dimensional expensive many-objective optimization,Model management
AI 理解论文
溯源树
样例
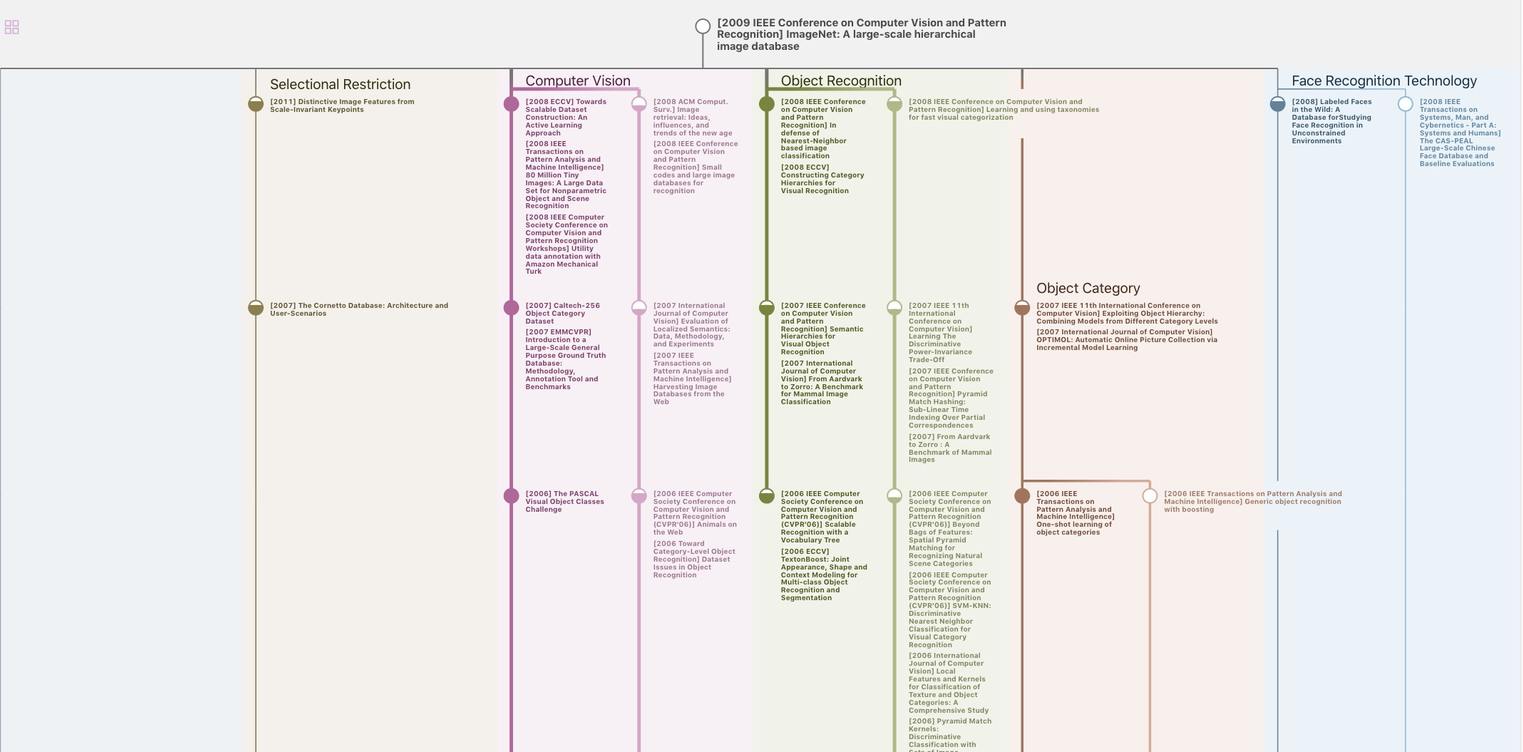
生成溯源树,研究论文发展脉络
Chat Paper
正在生成论文摘要