Simulated multimodal deep facial diagnosis
Expert Systems with Applications(2024)
Abstract
Facial phenotypes are extensively studied in medical and biological research, serving as critical markers that potentially indicate underlying genetic traits or medical conditions. With the recent advancements in big data, algorithms, and hardware, deep facial diagnosis, which employs deep learning techniques to systematically examine facial phenotypes and identify signs of certain diseases or medical conditions, has attracted significant attention and research, gradually emerging as a promising tool in precision medicine. Primarily limited by the scarcity of data for training facial diagnosis models, the accuracy of facial diagnosis for various conditions remains low up to now. In the past decade, RGB-D cameras, measuring depth information along with standard RGB capabilities, have proven superior in processing spatial details with more stability and accuracy. Motivated by the facts mentioned above, in this paper, we propose a Simulated Multimodal Framework, which effectively improves the computer-aided facial diagnosis performance of state-of-the-art models in experiments under different conditions. The underlying principle is to leverage the simulated depth by generative models to improve the performance of RGB image recognition. Furthermore, as a rapid and non-invasive tool for disease screening and detection, our proposal demonstrated an accuracy improvement of over 20% compared to practicing physicians in the study.
MoreTranslated text
Key words
Deep facial diagnosis,Simulated multimodal,Face depth estimation,Facial phenotypes,Condition-specific faces,Bilinear
AI Read Science
Must-Reading Tree
Example
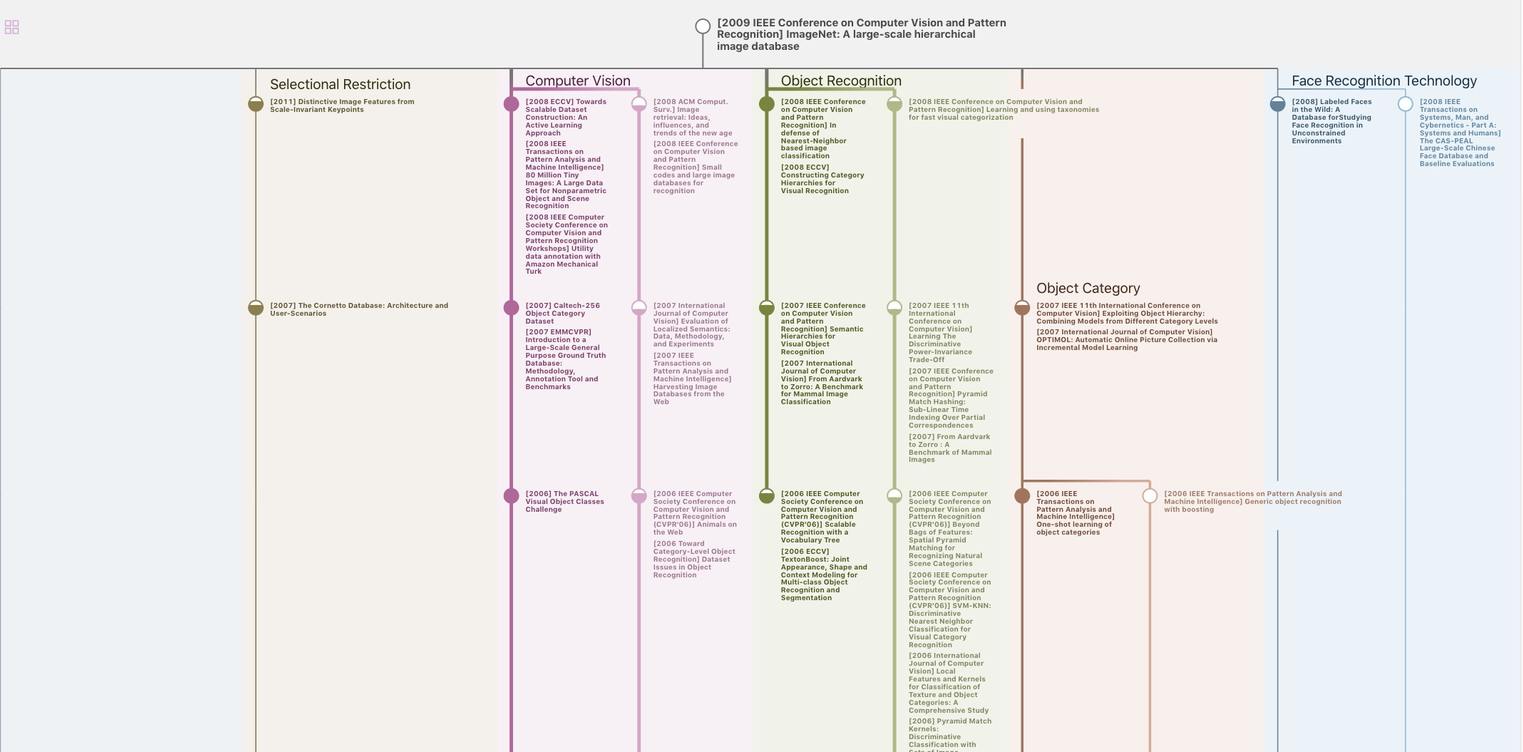
Generate MRT to find the research sequence of this paper
Chat Paper
Summary is being generated by the instructions you defined