Defend against Adversarial Attacks in Malware Detection through Attack Space Management
Computers & Security(2024)
摘要
In recent years, the application of machine learning techniques based on byte sequences in malware detection has become a prominent research area. However, relevant studies have shown that machine learning methods are susceptible to adversarial examples, and the use of byte sequences provides attackers with a convenient avenue for manipulation. Current research efforts primarily focus on data augmentation techniques to enhance detection capabilities. But these approaches require significant computational resources and lack robustness. In this paper, we propose a novel defense mechanism against adversarial attacks in the context of malware detection. Our approach effectively thwarts adversarial attacks by scanning the functionality-preserving attack space. Unlike existing methods, our approach eliminates the need for repetitive retraining, significantly reducing computational demands. Theoretically, it can also withstand unknown adversarial perturbations. Experimental validation demonstrates that our method not only maintains the prediction accuracy of MalConv but also enhances it. Furthermore, our best method successfully defended against almost all existing black-box and white-box attacks, reducing the number of escaping files from multiple to zero.
更多查看译文
关键词
Malware detection,Adversarial attacks,Machine learning security,Deep learning,Cyber security
AI 理解论文
溯源树
样例
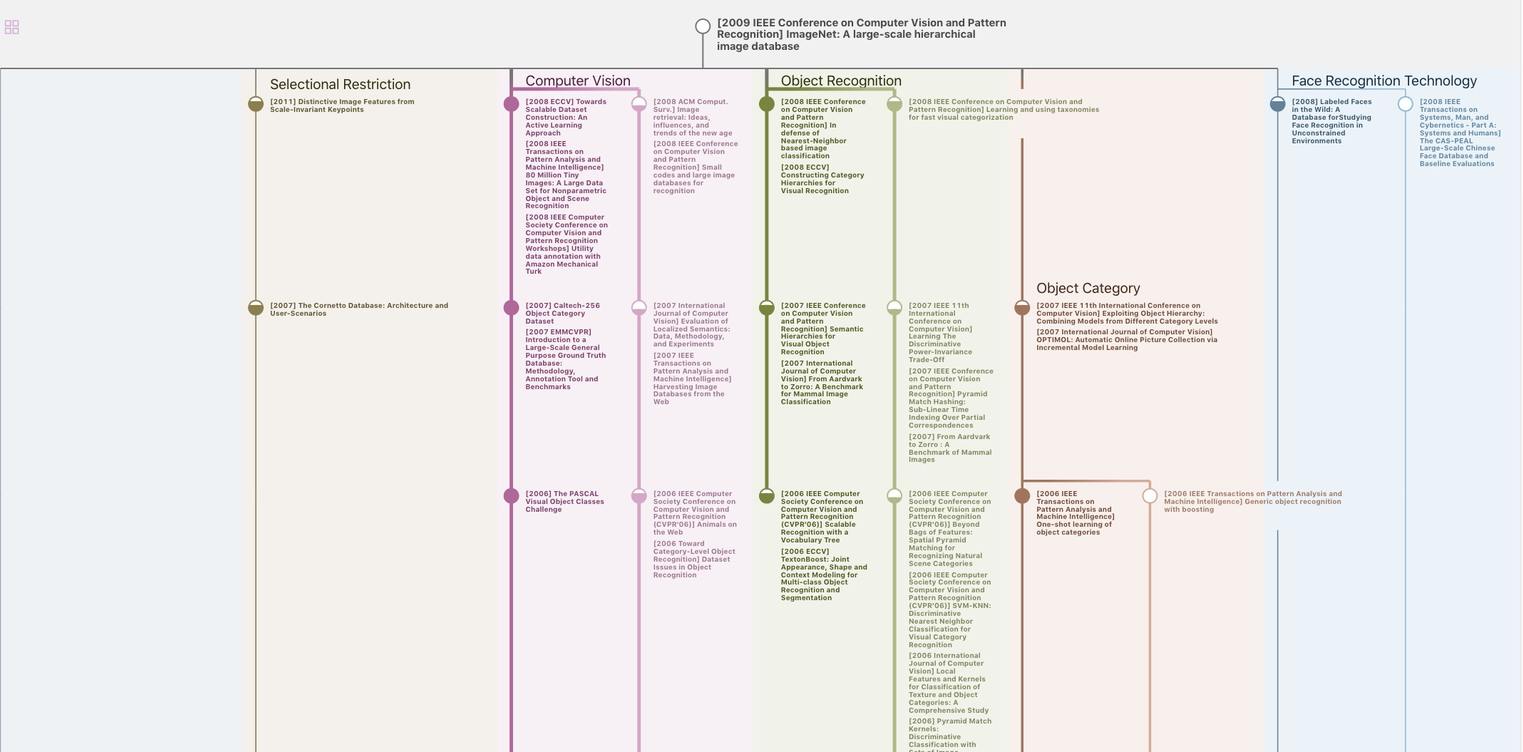
生成溯源树,研究论文发展脉络
Chat Paper
正在生成论文摘要