An all-inclusive model for predicting invasive bacterial infection in febrile infants age 7-60 days
PEDIATRIC RESEARCH(2024)
摘要
Background: Invasive bacterial infections (IBIs) in febrile infants are rare but potentially devastating. We aimed to derive and validate a predictive model for IBI among febrile infants age 7-60 days. Methods: Data were abstracted retrospectively from electronic records of 37 emergency departments (EDs) for infants with a measured temperature >=100.4 F who underwent an ED evaluation with blood and urine cultures. Models to predict IBI were developed and validated respectively using a random 80/20 dataset split, including 10-fold cross-validation. We used precision recall curves as the classification metric. Results: Of 4411 eligible infants with a mean age of 37 days, 29% had characteristics that would likely have excluded them from existing risk stratification protocols. There were 196 patients with IBI (4.4%), including 43 (1.0%) with bacterial meningitis. Analytic approaches varied in performance characteristics (precision recall range 0.04-0.29, area under the curve range 0.5-0.84), with the XGBoost model demonstrating the best performance (0.29, 0.84). The five most important variables were serum white blood count, maximum temperature, absolute neutrophil count, absolute band count, and age in days. Conclusion: A machine learning model (XGBoost) demonstrated the best performance in predicting a rare outcome among febrile infants, including those excluded from existing algorithms.
更多查看译文
AI 理解论文
溯源树
样例
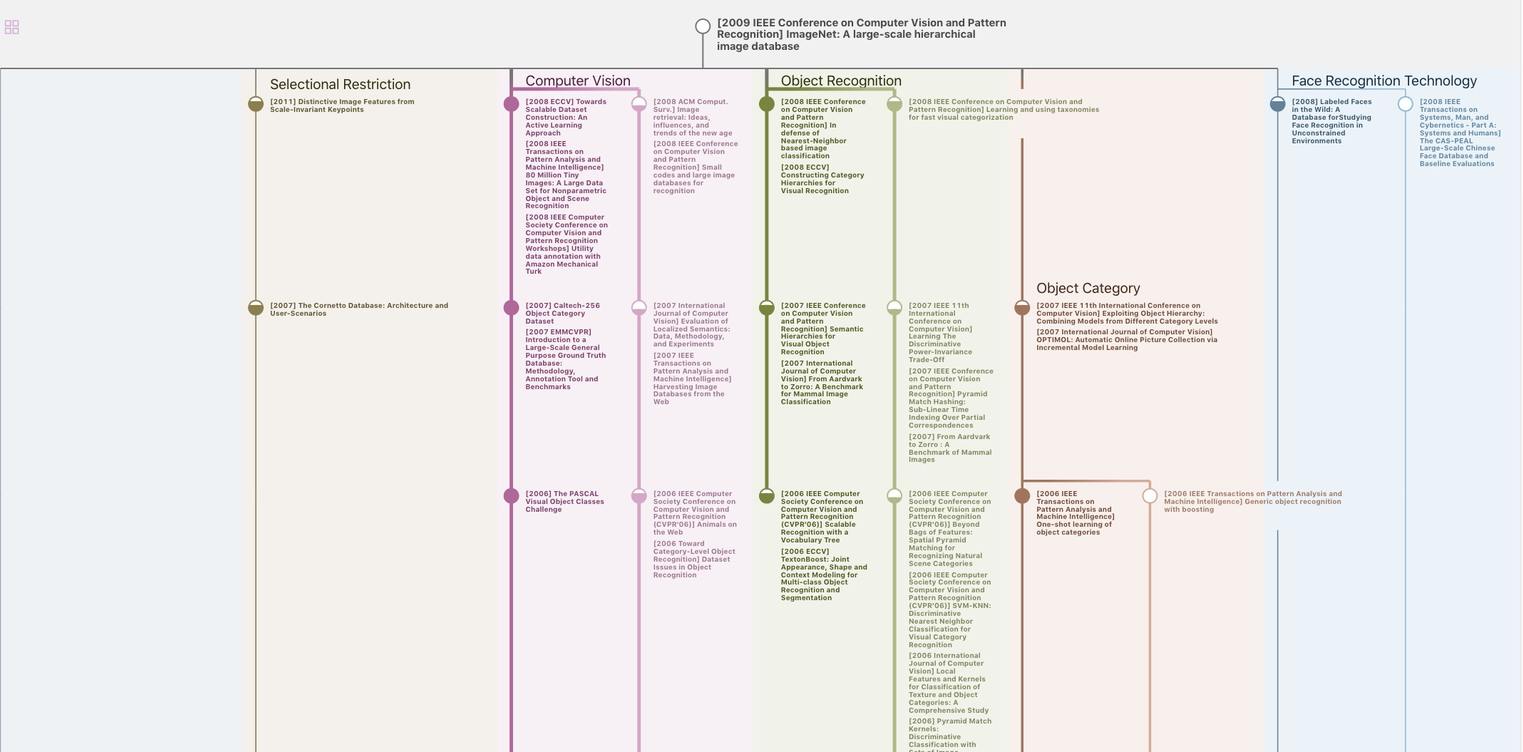
生成溯源树,研究论文发展脉络
Chat Paper
正在生成论文摘要