DeViDe: Faceted medical knowledge for improved medical vision-language pre-training
arxiv(2024)
摘要
Vision-language pre-training for chest X-rays has made sig- nificant strides,
primarily by utilizing paired radiographs and radiology reports. However,
existing approaches often face challenges in encoding medical knowledge
effectively. While radiology reports provide insights into the current disease
manifestation, medical definitions (as used by contemporary methods) tend to be
overly abstract, creating a gap in knowledge. To address this, we propose
DeViDe, a novel transformer- based method that leverages radiographic
descriptions from the open web. These descriptions outline general visual
characteristics of diseases in radiographs, and when combined with abstract
definitions and radiol- ogy reports, provide a holistic snapshot of knowledge.
DeViDe incorpo- rates three key features for knowledge-augmented vision
language align- ment: First, a large-language model-based augmentation is
employed to homogenise medical knowledge from diverse sources. Second, this
knowl- edge is aligned with image information at various levels of granularity.
Third, a novel projection layer is proposed to handle the complexity of
aligning each image with multiple descriptions arising in a multi-label
setting. In zero-shot settings, DeViDe performs comparably to fully su-
pervised models on external datasets and achieves state-of-the-art results on
three large-scale datasets. Additionally, fine-tuning DeViDe on four downstream
tasks and six segmentation tasks showcases its superior per- formance across
data from diverse distributions.
更多查看译文
AI 理解论文
溯源树
样例
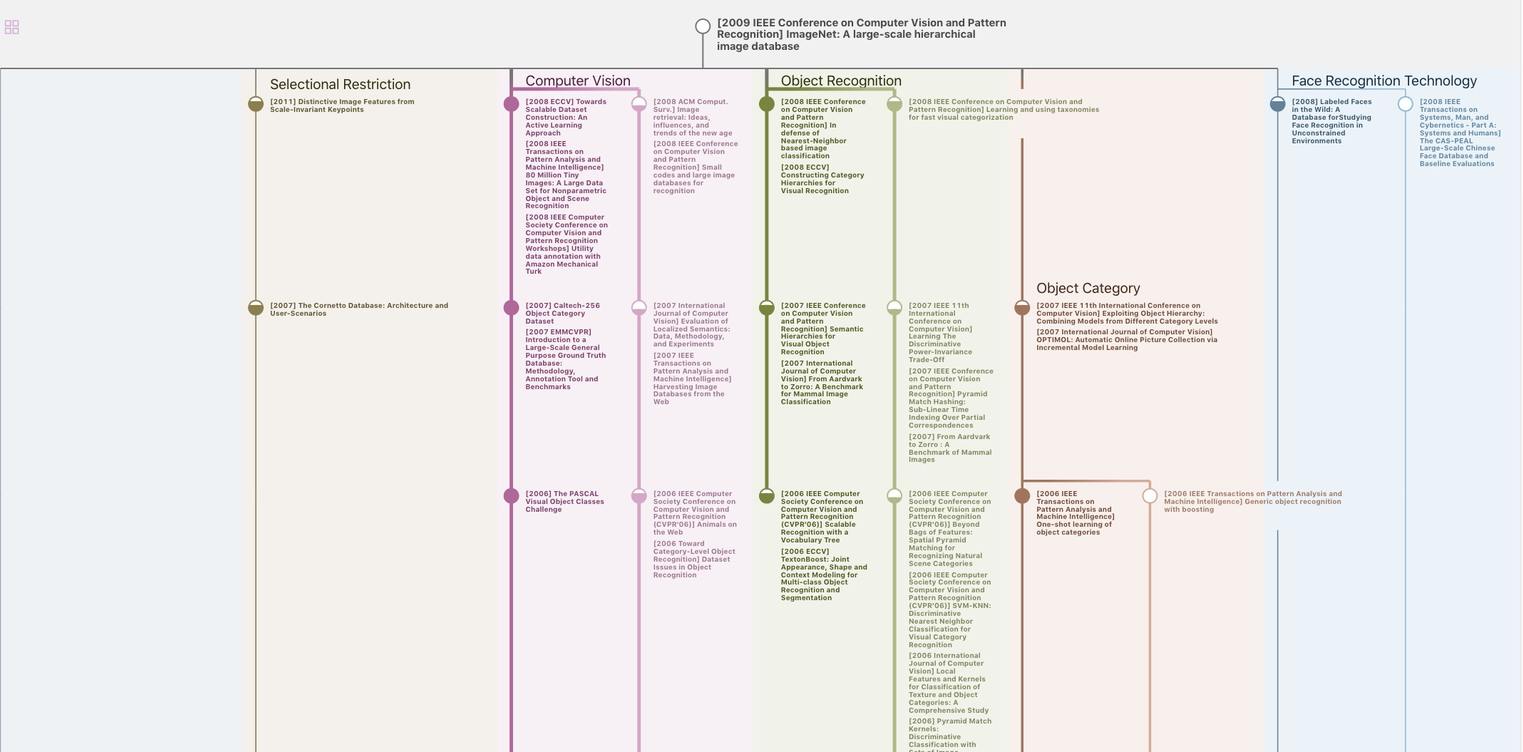
生成溯源树,研究论文发展脉络
Chat Paper
正在生成论文摘要