HiMAL: A Multimodal Hierarchical Multi-task Auxiliary Learning framework for predicting and explaining Alzheimer disease progression
arxiv(2024)
摘要
Objective: We aimed to develop and validate a novel multimodal framework
HiMAL (Hierarchical, Multi-task Auxiliary Learning) framework, for predicting
cognitive composite functions as auxiliary tasks that estimate the longitudinal
risk of transition from Mild Cognitive Impairment (MCI) to Alzheimer Disease
(AD).
Methods: HiMAL utilized multimodal longitudinal visit data including imaging
features, cognitive assessment scores, and clinical variables from MCI patients
in the Alzheimer Disease Neuroimaging Initiative (ADNI) dataset, to predict at
each visit if an MCI patient will progress to AD within the next 6 months.
Performance of HiMAL was compared with state-of-the-art single-task and
multi-task baselines using area under the receiver operator curve (AUROC) and
precision recall curve (AUPRC) metrics. An ablation study was performed to
assess the impact of each input modality on model performance. Additionally,
longitudinal explanations regarding risk of disease progression were provided
to interpret the predicted cognitive decline.
Results: Out of 634 MCI patients (mean [IQR] age : 72.8 [67-78], 60
209 (32
to all single-modality singe-task baselines (AUROC = 0.923 [0.915-0.937];
AUPRC= 0.623 [0.605-0.644]; all p<0.05). Ablation analysis highlighted that
imaging and cognition scores with maximum contribution towards prediction of
disease progression.
Discussion: Clinically informative model explanations anticipate cognitive
decline 6 months in advance, aiding clinicians in future disease progression
assessment. HiMAL relies on routinely collected EHR variables for proximal (6
months) prediction of AD onset, indicating its translational potential for
point-of-care monitoring and managing of high-risk patients.
更多查看译文
AI 理解论文
溯源树
样例
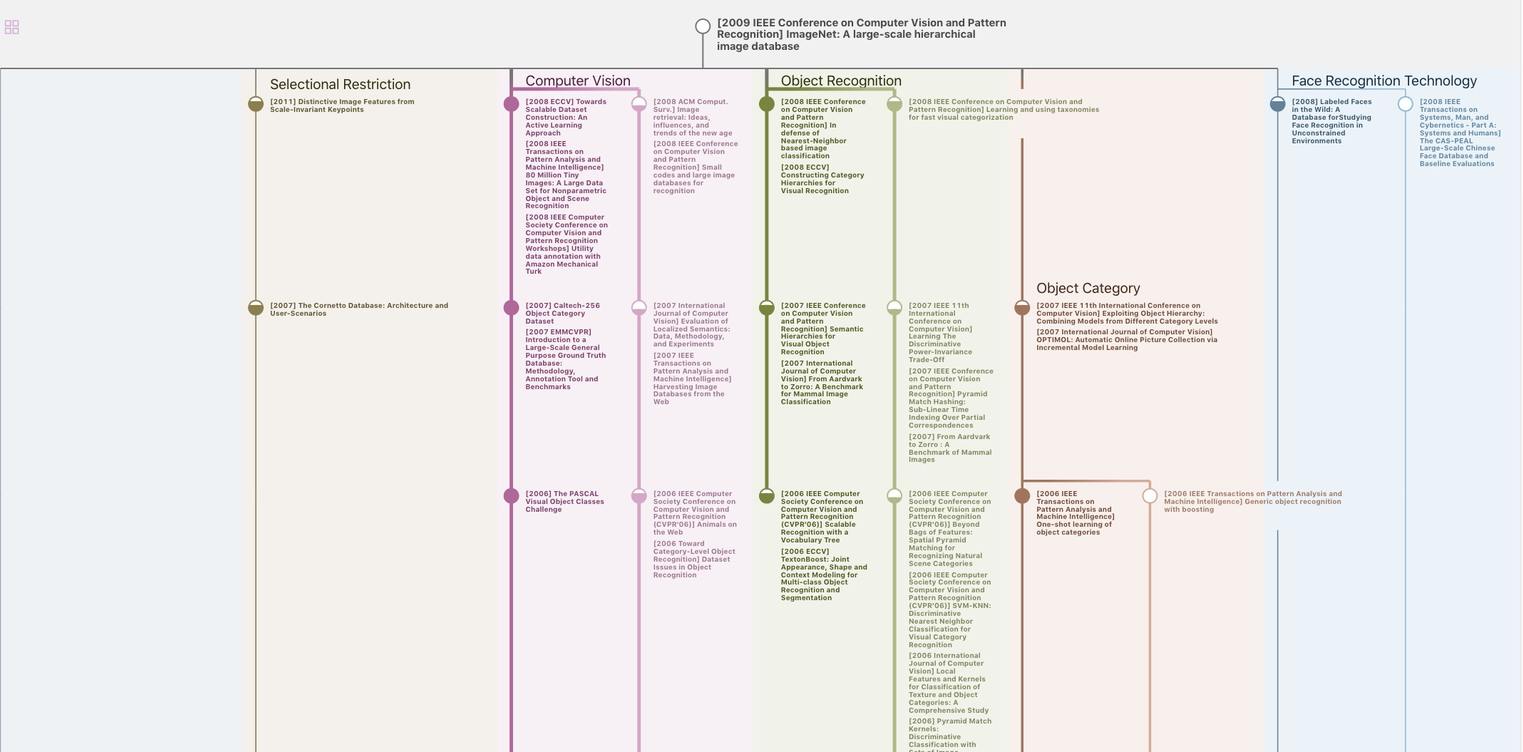
生成溯源树,研究论文发展脉络
Chat Paper
正在生成论文摘要