Foundation Models for Structural Health Monitoring
CoRR(2024)
Abstract
Structural Health Monitoring (SHM) is a critical task for ensuring the safety
and reliability of civil infrastructures, typically realized on bridges and
viaducts by means of vibration monitoring. In this paper, we propose for the
first time the use of Transformer neural networks, with a Masked Auto-Encoder
architecture, as Foundation Models for SHM. We demonstrate the ability of these
models to learn generalizable representations from multiple large datasets
through self-supervised pre-training, which, coupled with task-specific
fine-tuning, allows them to outperform state-of-the-art traditional methods on
diverse tasks, including Anomaly Detection (AD) and Traffic Load Estimation
(TLE). We then extensively explore model size versus accuracy trade-offs and
experiment with Knowledge Distillation (KD) to improve the performance of
smaller Transformers, enabling their embedding directly into the SHM edge
nodes. We showcase the effectiveness of our foundation models using data from
three operational viaducts. For AD, we achieve a near-perfect 99.9
with a monitoring time span of just 15 windows. In contrast, a state-of-the-art
method based on Principal Component Analysis (PCA) obtains its first good
result (95.03
tasks, our models obtain state-of-the-art performance on multiple evaluation
metrics (R^2 score, MAE
R^2 score of 0.97 and 0.85 for light and heavy vehicle traffic, respectively,
while the best previous approach stops at 0.91 and 0.84. On the second one, we
achieve an R^2 score of 0.54 versus the 0.10 of the best existing method.
MoreTranslated text
AI Read Science
Must-Reading Tree
Example
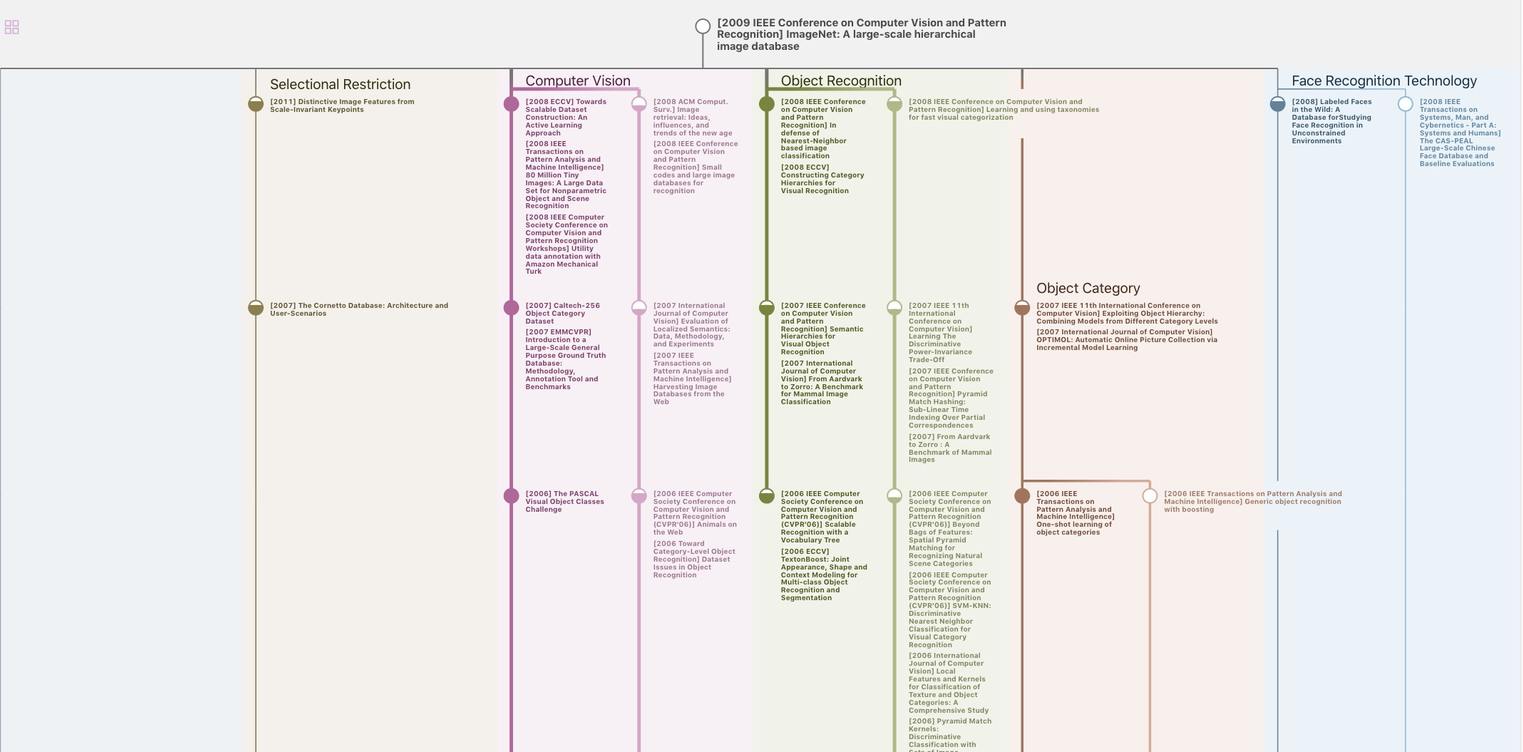
Generate MRT to find the research sequence of this paper
Chat Paper
Summary is being generated by the instructions you defined