Min-K Language Models
arxiv(2024)
摘要
The problem of pre-training data detection for large language models (LLMs)
has received growing attention due to its implications in critical issues like
copyright violation and test data contamination. A common intuition for this
problem is to identify training data by checking if the input comes from a mode
of the LLM's distribution. However, existing approaches, including the
state-of-the-art Min-K
are less robust in determining local maxima than second-order statistics. In
this work, we propose a novel methodology Min-K
detection that measures how sharply peaked the likelihood is around the input,
a measurement analogous to the curvature of continuous distribution. Our method
is theoretically motivated by the observation that maximum likelihood training
implicitly optimizes the trace of the Hessian matrix of likelihood through
score matching. Empirically, the proposed method achieves new SOTA performance
across multiple settings. On the WikiMIA benchmark, Min-K
runner-up by 6.2
more challenging MIMIR benchmark, it consistently improves upon reference-free
methods while performing on par with reference-based method that requires an
extra reference model.
更多查看译文
AI 理解论文
溯源树
样例
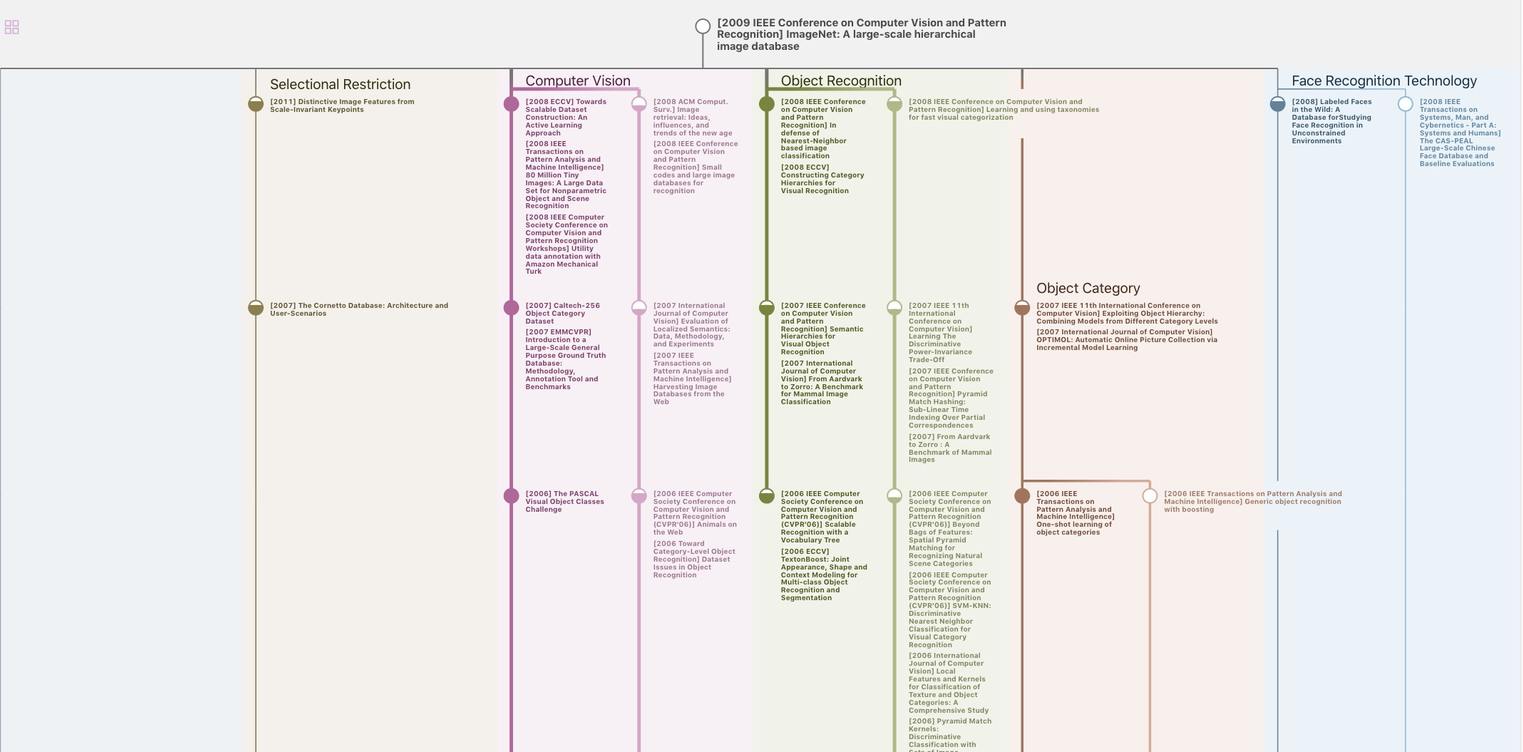
生成溯源树,研究论文发展脉络
Chat Paper
正在生成论文摘要