Trish: an efficient activation function for CNN models and analysis of its effectiveness with optimizers in diagnosing glaucoma
JOURNAL OF SUPERCOMPUTING(2024)
摘要
Glaucoma is an eye disease that spreads over time without showing any symptoms at an early age and can result in vision loss in advanced ages. The most critical issue in this disease is to detect the symptoms of the disease at an early age. Various researches are carried out on machine learning approaches that will provide support to the expert for this diagnosis. The activation function plays a pivotal role in deep learning models, as it introduces nonlinearity, enabling neural networks to learn complex patterns and relationships within data, thus facilitating accurate predictions and effective feature representations. In this study, it is focused on developing an activation function that can be used in CNN architectures using glaucoma disease datasets. The developed function (Trish) was compared with ReLU, LReLU, Mish, Swish, Smish, and Logish activation functions using SGD, Adam, RmsProp, AdaDelta, AdaGrad, Adamax, and Nadam optimizers in CNN architectures. Datasets consisting of retinal fundus images named ACRIMA and HRF were used within the scope of the experiments. These datasets are widely known and currently used in the literature. To strengthen the test validity, the proposed function was also tested on the CIFAR-10 dataset. As a result of the study, 97.22% validation accuracy performance was obtained. It should be stated that the acquired performance value is at a significant level for the detection of glaucoma.
更多查看译文
关键词
Activation function,Artificial learning,Convolutional neural network (CNN),Glaucoma diagnosis,Optimizer
AI 理解论文
溯源树
样例
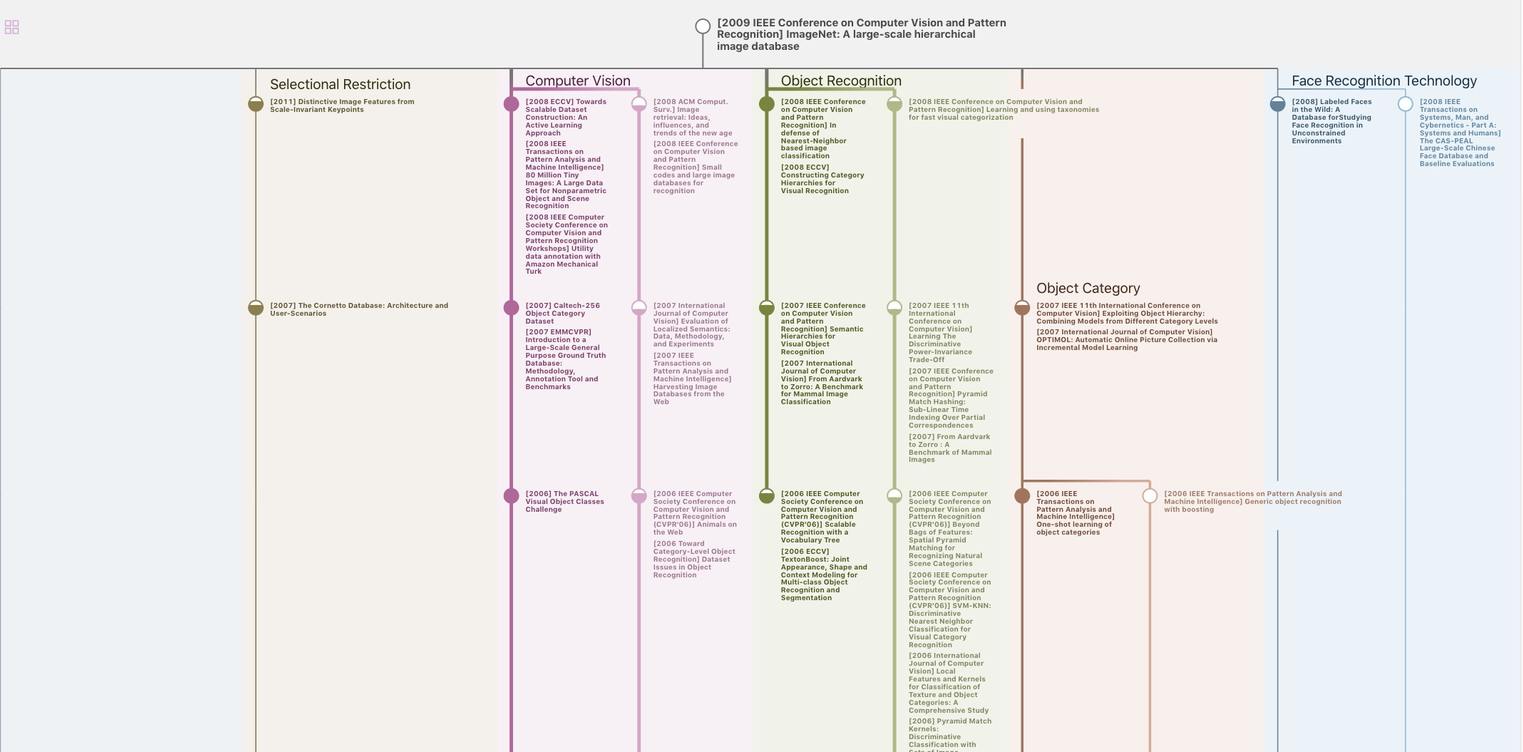
生成溯源树,研究论文发展脉络
Chat Paper
正在生成论文摘要