Dissecting Renewable Uncertainty via Deconstructive Analysis-Based Data Valuation
IEEE Transactions on Industry Applications(2024)
摘要
Integrating renewable energy sources into power systems is crucial to lower carbon emissions, yet the resulting uncertainty presents challenges to network reliability. In the era of digital energy, Big Data models help reduce uncertainty by identifying data patterns for accurate predictions. Yet, the efficacy of data-driven models is constrained by the scarcity of high-quality training data, underscoring the significance of identifying and selecting datasets of superior quality. This paper proposes a novel data valuation framework based on deep reinforcement learning for the analysis and decomposition of uncertainty in renewable energy datasets. Our framework merges meteorological and power uncertainty through predictive tasks, training a neural network to uncover the intrinsic relationships between data features and their value via a sampling-feedback mechanism. By incorporating policy gradient and other optimization techniques, we enhance the algorithm's stability and efficiency, supplemented by comparative experiments for validation. We tested our valuation approach using 2017-2018 aggregate wind related data from Yunnan province for power forecasting. The results demonstrate that our proposed data value approach effectively enhances the quality of the dataset, leading to a proportional improvement of 7.69% in prediction accuracy.
更多查看译文
关键词
Data quality,data valuation,meteorological feature,policy gradient,reinforcement learning,renewable uncertainty,wind power forecasting
AI 理解论文
溯源树
样例
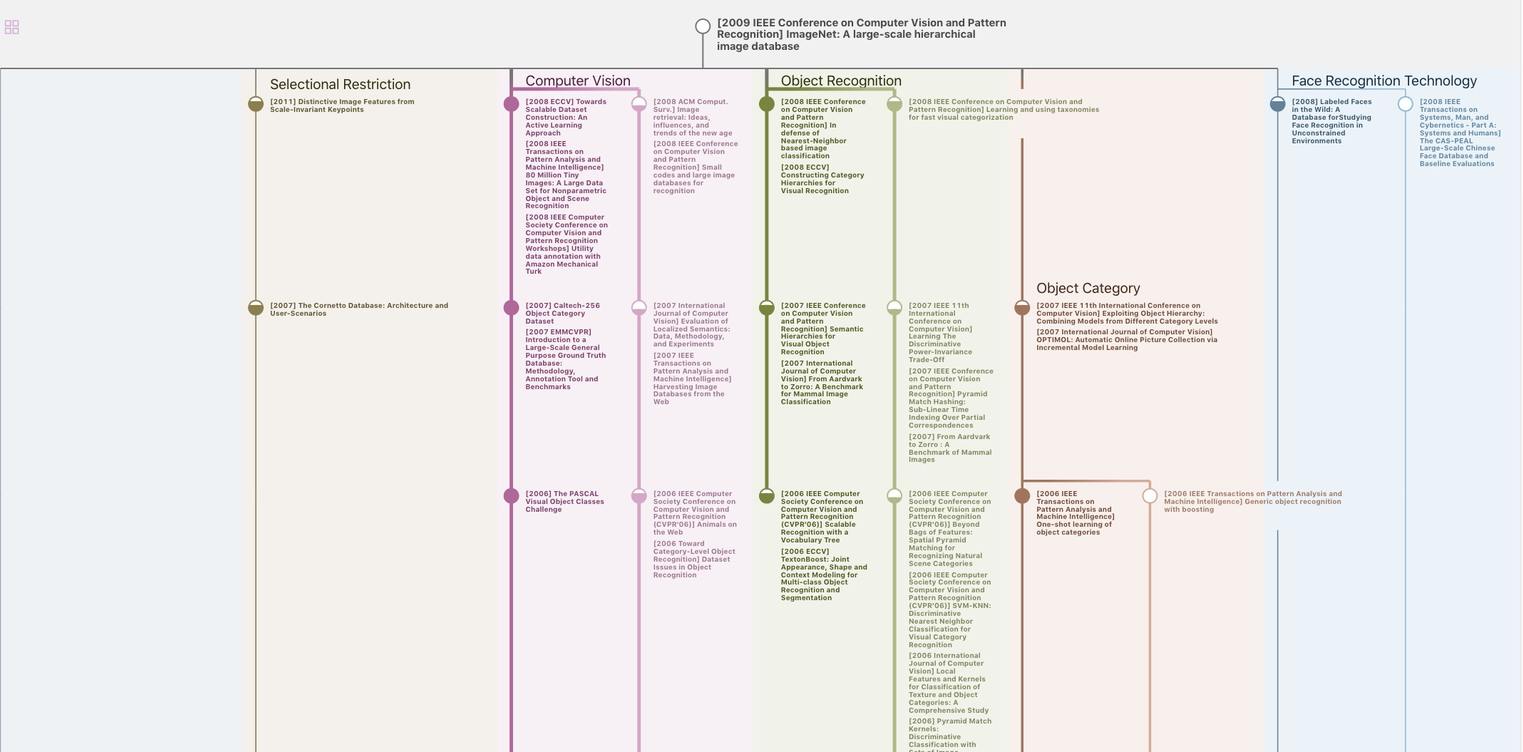
生成溯源树,研究论文发展脉络
Chat Paper
正在生成论文摘要