Generalized Rapid TFT Modeling (GRTM) Framework for Agile Device Modeling with Thin Film Transistors
IEEE Journal on Flexible Electronics(2024)
摘要
This paper introduces the Generalized Rapid TFT Modeling (GRTM) framework, an innovative approach utilizing deep learning (DL) techniques for efficient and accurate modeling and generation of Verilog-A code of Thin-Film-Transistor (TFT) devices. Traditional TFT modeling methods, such as physics-based and Look-Up Table (LUT)-based models, often involve complex, manual parameter tuning and struggle with generalizability across different device types. The GRTM framework streamlines the modeling process by leveraging DL algorithms to automatically learn from input datasets, significantly reducing human effort in parameter extraction and fitting. Thus, a new aspect of GRTM is its compatibility with commercial SPICE simulators, achieved by converting DL models into Verilog-A SPICE code. The framework’s efficacy is demonstrated through its application to Low-Temperature Polysilicon (LTPS) TFT devices, showing a fourfold increase in accuracy and a substantial reduction in model development time compared to conventional physics-based models. The performance and features of the GRTM framework are compared with existing methods, highlighting its potential to revolutionize TFT device modeling.
更多查看译文
关键词
Thin-Film Transistors (TFT),Low-Temperature Polysilicon (LTPS),Field-Effect Transistors (FETs),Current-Voltage (I-V) Surrogate Model,Deep Learning (DL),Design Technology Co-Optimization (DTCO),Device Modeling,Circuit Design
AI 理解论文
溯源树
样例
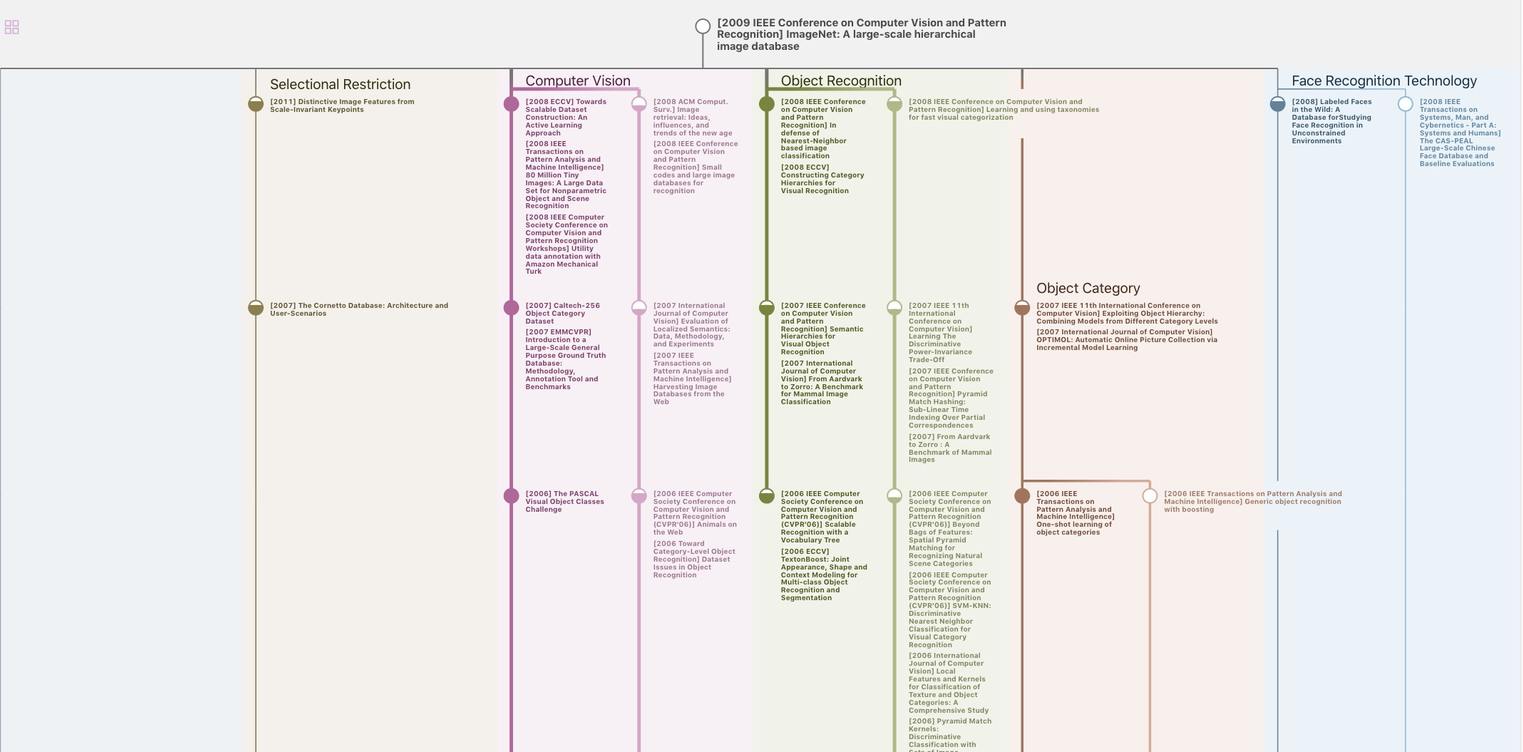
生成溯源树,研究论文发展脉络
Chat Paper
正在生成论文摘要