De-biased Disentanglement Learning for Pulmonary Embolism Survival Prediction on Multimodal Data.
IEEE journal of biomedical and health informatics(2024)
摘要
Health disparities among marginalized populations with lower socioeconomic status significantly impact the fairness and effectiveness of healthcare delivery. The increasing integration of artificial intelligence (AI) into healthcare presents an opportunity to address these inequalities, provided that AI models are free from bias. This paper aims to address the bias challenges by population disparities within healthcare systems, existing in the presentation of and development of algorithms, leading to inequitable medical implementation for conditions such as pulmonary embolism (PE) prognosis. In this study, we explore the diversity of biases in healthcare systems, which highlights the need for a holistic framework to reduce bias by complementary aggregation. By leveraging de-biasing deep survival prediction models, we propose a framework that disentangles identifiable information from images, text reports, and clinical variables to mitigate potential biases within multimodal datasets. Our study offers several advantages over traditional clinical-based survival prediction methods, including richer survival-related characteristics and bias-complementary predicted results. By improving the robustness of survival analysis through this framework, we aim to benefit patients, clinicians, and researchers by improving fairness and accuracy in healthcare AI systems. The code is available at https://github.com/zzs95/fairPE-SA.
更多查看译文
关键词
Survival Analysis,Fairness,Disentangled Representation Learning,Multimodal Fusion,Pulmonary Embolism,Survival Prediction
AI 理解论文
溯源树
样例
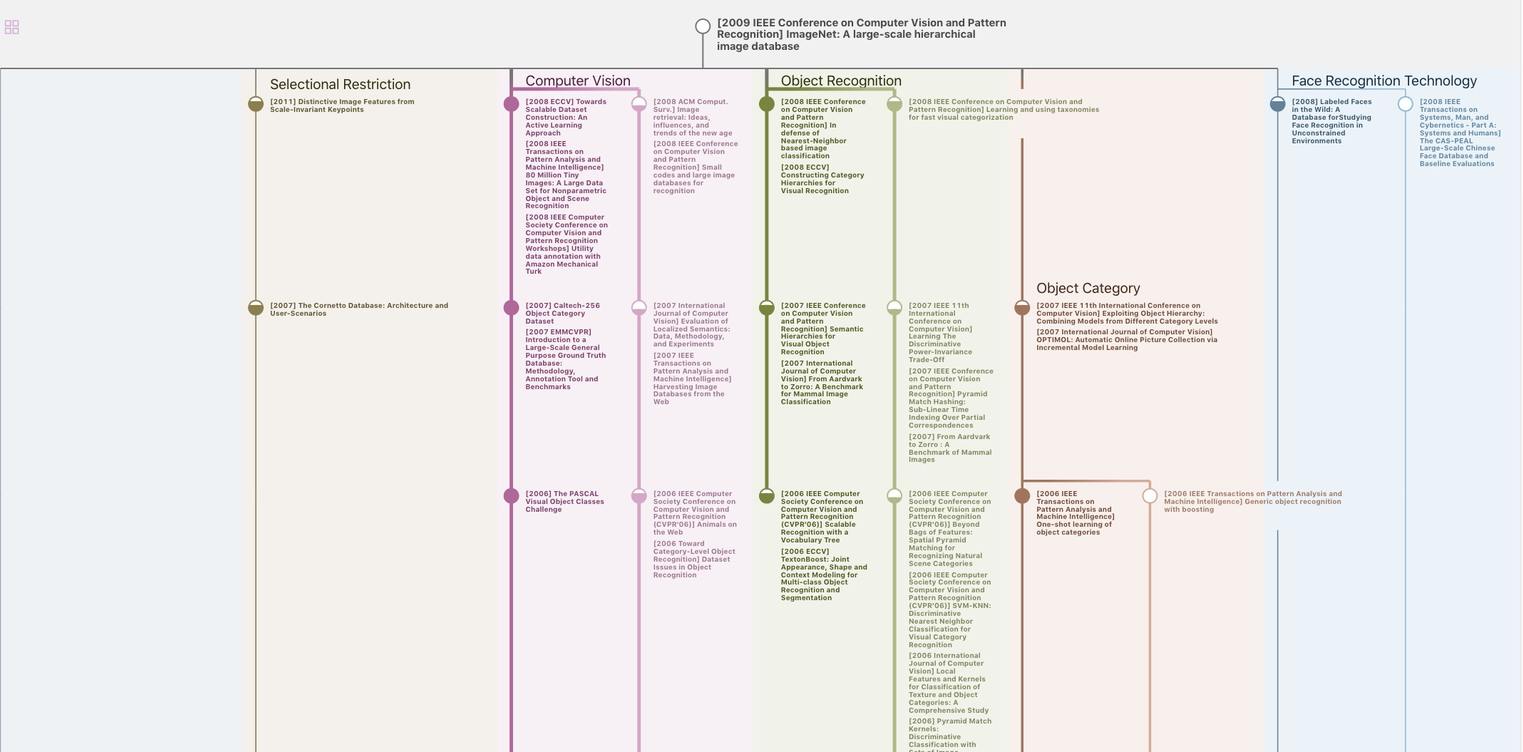
生成溯源树,研究论文发展脉络
Chat Paper
正在生成论文摘要