SDN-based detection and mitigation of DDoS attacks on smart homes
Computer Communications(2024)
摘要
The adoption of the Internet of Things (IoT) has proliferated across various domains, where everyday objects like refrigerators and washing machines are now equipped with sensors and connected to the internet. Undeniably, the security of such devices, which were not primarily designed for internet connectivity, is of utmost importance but has been largely neglected. In this paper, we propose a framework for real-time DDoS attack detection and mitigation in SDN-enabled smart home networks. We capture network traffic during regular operations and during DDoS attacks. This captured traffic is used to train several machine learning (ML) models, including Support Vector Machine (SVM), Logistic Regression, Decision Trees, and K-Nearest Neighbors (KNN) algorithms. These trained models are executed as SDN controller applications and subsequently employed for real-time attack detection. While we utilize ML techniques to protect IoT devices, we propose the use of SNORT, a signature-based detection technique, to secure the SDN controller itself. Real-world experiments demonstrate that without SNORT, the SDN controller goes offline shortly after an attack, resulting in a 100% packet loss. Furthermore, we show that ML algorithms can efficiently classify traffic into benign and attack traffic, with the Decision Tree algorithm outperforming others with an accuracy of 99%.
更多查看译文
关键词
Software-defined networks,Internet of Things,DDoS attacks,Intrusion detection system,Supervised classification
AI 理解论文
溯源树
样例
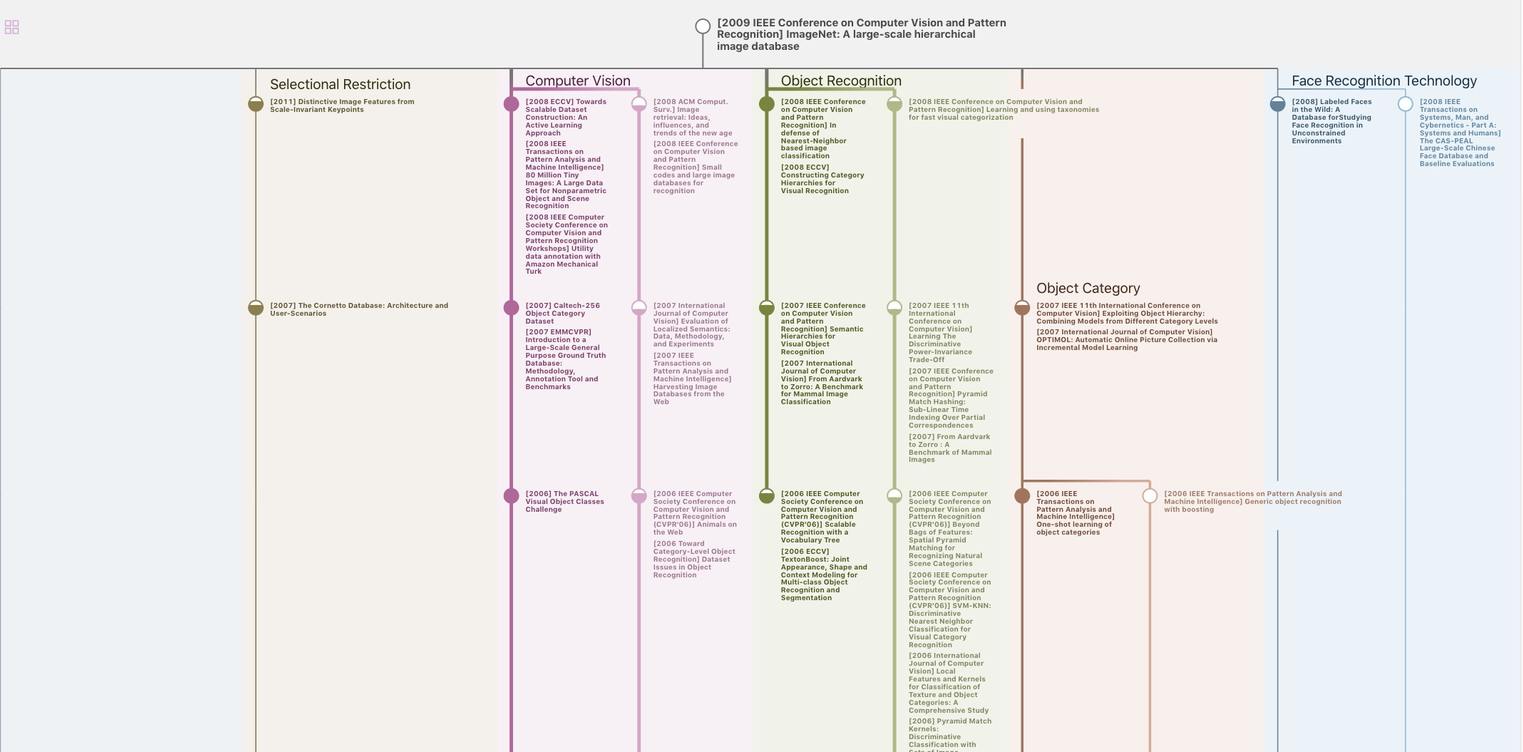
生成溯源树,研究论文发展脉络
Chat Paper
正在生成论文摘要