Machine learning based detection of T–wave alternans in real ambulatory conditions
Computer Methods and Programs in Biomedicine(2024)
摘要
Background and objective
T-wave alternans (TWA) is a fluctuation in the repolarization morphology of the ECG. It is associated with cardiac instability and sudden cardiac death risk. Diverse methods have been proposed for TWA analysis. However, TWA detection in ambulatory settings remains a challenge due to the absence of standardized evaluation metrics and detection thresholds.
Methods
In this work we use traditional TWA analysis signal processing-based methods for feature extraction, and two machine learning (ML) methods, namely, K–nearest–neighbor (KNN) and random forest (RF), for TWA detection, addressing hyper–parameter tuning and feature selection. The final goal is the detection in ambulatory recordings of short, non-sustained and sparse TWA events.
Results
We train ML methods to detect a wide variety of alternant voltage from 20 to 100 μV, i.e., ranging from non–visible micro–alternans to TWA of higher amplitudes, to recognize a wide range in concordance to risk stratification. In classification, RF outperforms significantly the recall in comparison with the signal processing methods, at the expense of a small lost in precision. Despite ambulatory detection stands for an imbalanced category context, the trained ML systems always outperform signal processing methods.
Conclusions
We propose a comprehensive integration of multiple variables inspired from TWA signal processing methods to fed learning-based methods. ML models consistently outperform the best signal processing methods, yielding superior recall scores.
更多查看译文
关键词
Machine Learning (ML),Spectral Method (SM),Modified Moving Average Method (MMA),Time Method (TM),Cross Validation (CV),Repolarization,T–Wave Alternans (TWA),Electrocardiogram (ECG)
AI 理解论文
溯源树
样例
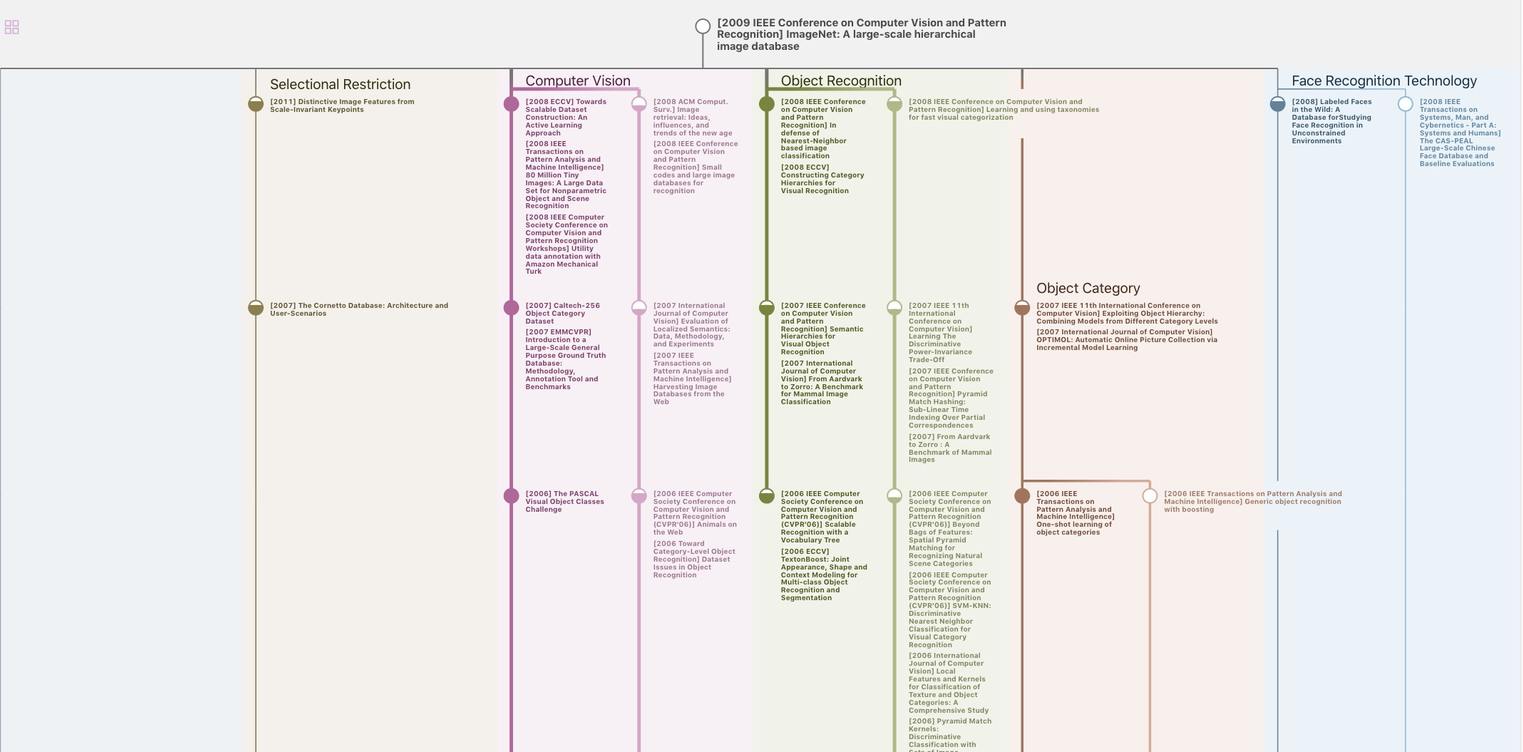
生成溯源树,研究论文发展脉络
Chat Paper
正在生成论文摘要