Landslide susceptibility prediction and mapping in Loess Plateau based on different machine learning algorithms by hybrid factors screening: Case Study of Xunyi County, Shaanxi Province, China
Advances in Space Research(2024)
Abstract
Machine learning models are widely used for landslide susceptibility prediction (LSP). To better develop a landslide susceptibility prediction model for the Loess Plateau, this study compared different machine-learning models (Logistic regression (LR), support vector machines (SVM), random forests (RF), Naïve Bayes (NB), and multilayer perceptron (MLP)). Using Xunyi County, China as the study area, 1096 landslides were collected and divided into a training set (70%) and a test set (30%). Significant influencing factors were selected from 20 landslide factors using GeoDetector. The relationships between these factors and landslides were determined by normalized frequency ratios. Five trained machine learning models calculated landslide susceptibility indices and generated landslide susceptibility maps. Additionally, the accuracy (ACC), precision (PRE), recall (REC), F1 score, AUC values, LSI distribution characteristics, and overlay analysis of historical landslides with LSM were used to evaluate the performance of the models. The results show that the LSP results of the five models are generally reasonable. The results further indicated that the use of GeoDetector was able to filter out the main factors of eolian landslides, improving the predictive performance of the model and obtaining better LSMs. The RF model had the best overall performance, followed by the SVM, and the MLP model created LSM high susceptibility zones that could contain the highest number of historical landslides. The q-values of factor contributions indicate that elevation, NDVI, rainfall, POI kernel density, and RDLS are the main factors controlling landslides, which need to be paid attention to for landslide control in the Loess Plateau region. The results of this study can provide a reference for the follow-up study of landslide prevention and control, especially landslide susceptibility modeling in the Loess Plateau.
MoreTranslated text
Key words
Landslide susceptibility,Loess Plateau,Machine learning
AI Read Science
Must-Reading Tree
Example
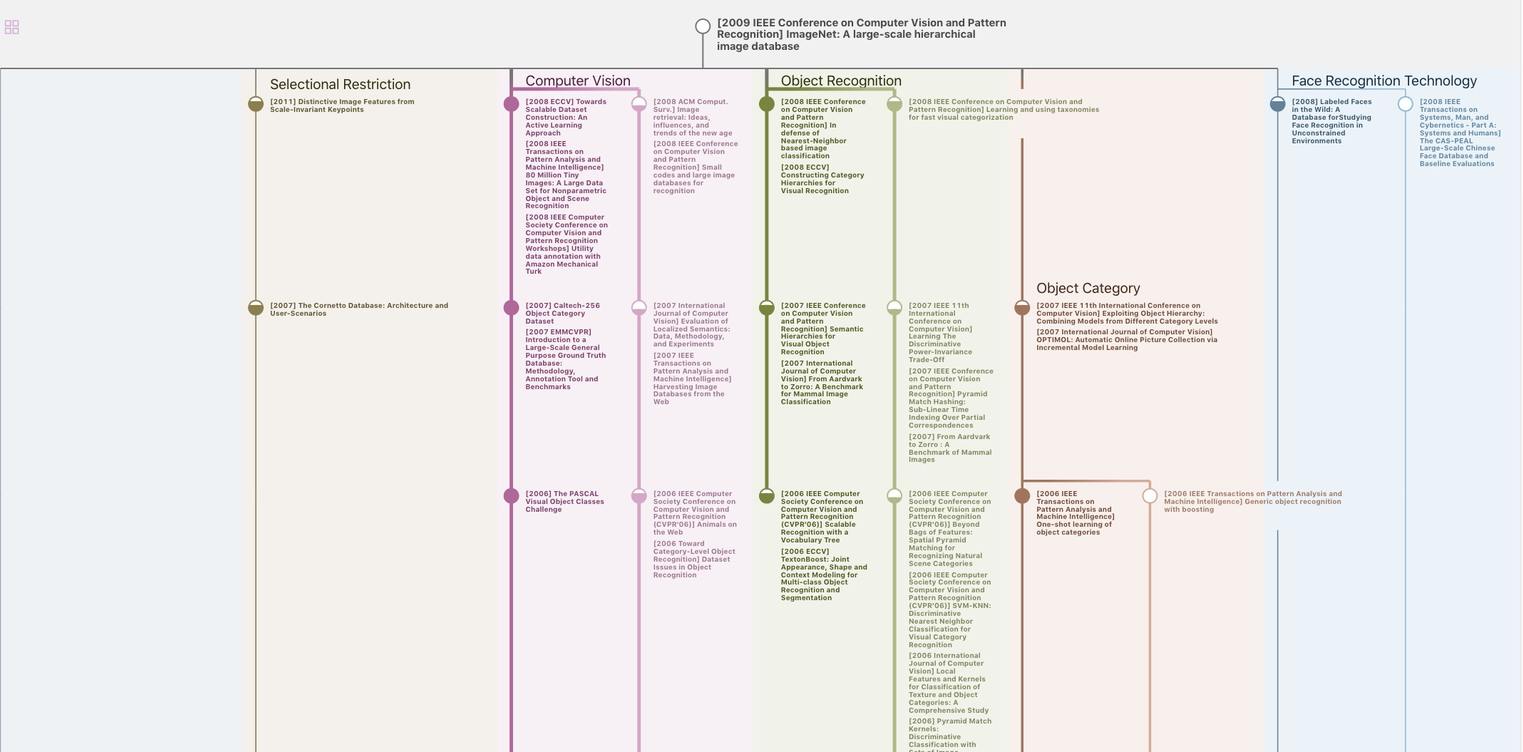
Generate MRT to find the research sequence of this paper
Chat Paper
Summary is being generated by the instructions you defined