A neuroergonomics model for evaluating nuclear power plants operators’ performance under heat stress driven by ECG time-frequency spectrums and fNIRS prefrontal cortex network: A CNN-GAT fusion model
CoRR(2024)
Abstract
Operators experience complicated physiological and psychological states when exposed to extreme heat stress, which can impair cognitive function and decrease performance significantly, ultimately leading to severe secondary disasters. Therefore, there is an urgent need for a feasible technique to identify their abnormal states to enhance the reliability of human-cybernetics systems. With the advancement of deep learning in physiological modeling, a model for evaluating operators’ performance driven by electrocardiogram (ECG) and functional near-infrared spectroscopy (fNIRS) was proposed, demonstrating high ecological validity. The model fused a convolutional neural network (CNN) backbone and a graph attention network (GAT) backbone to extract discriminative features from ECG time-frequency spectrums and fNIRS prefrontal cortex (PFC) network respectively with deeper neuroscience domain knowledge, and eventually achieved 0.90 AUC. Results supported that handcrafted features extracted by specialized neuroscience methods can alleviate overfitting. Inspired by the small-world nature of the brain network, the fNIRS PFC network was organized as an undirected graph and embedded by GAT. It is proven to perform better in information aggregation and delivery compared to a simple non-linear transformation. The model provides a potential neuroergonomics application to evaluate the human state in vital human-cybernetics systems under industry 5.0 scenarios.
MoreTranslated text
Key words
Graph attention network,Electrocardiogram,Functional near-infrared spectroscopy,Heat stress,Neuroergonomics
AI Read Science
Must-Reading Tree
Example
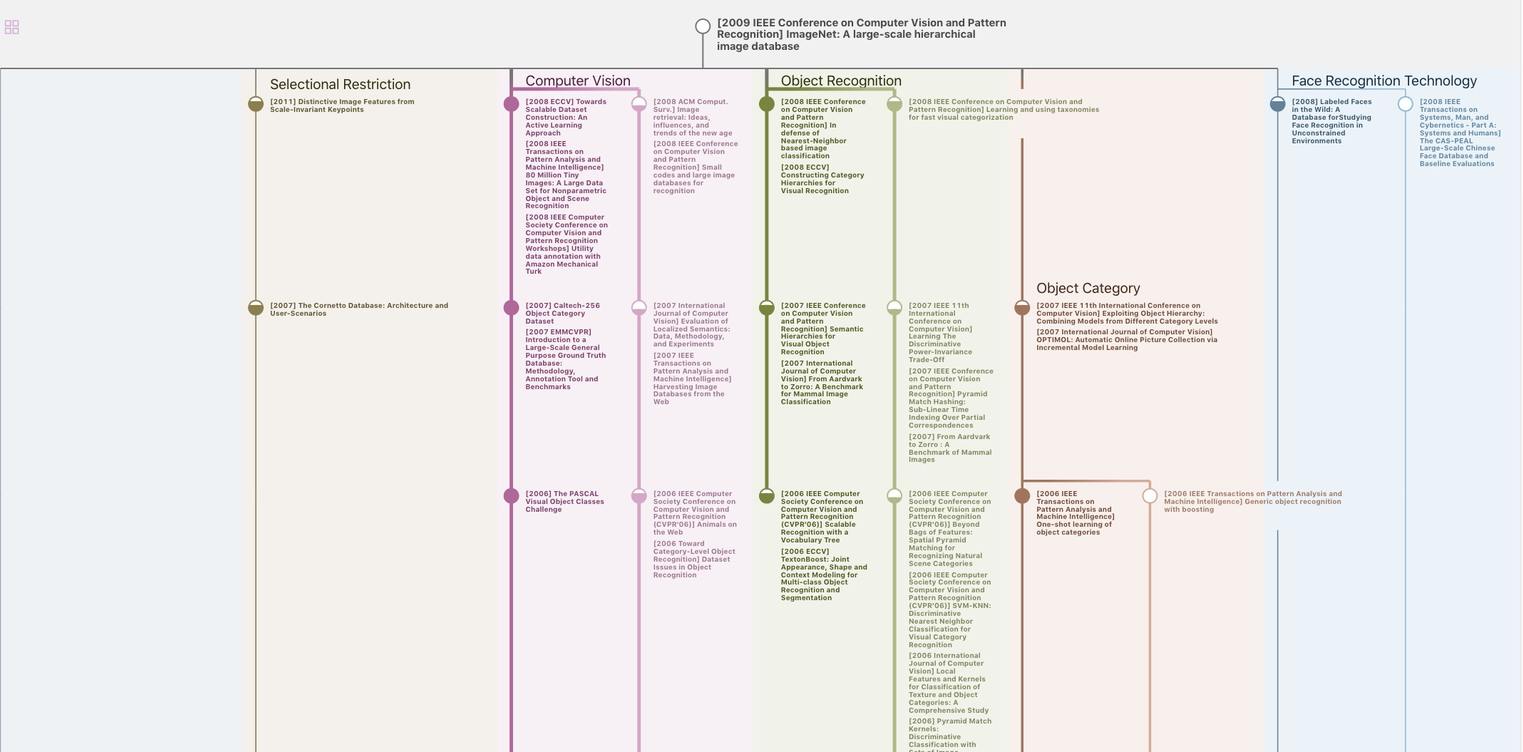
Generate MRT to find the research sequence of this paper
Chat Paper
Summary is being generated by the instructions you defined