Smooth Deep Saliency
CoRR(2024)
Abstract
In this work, we investigate methods to reduce the noise in deep saliency
maps coming from convolutional downsampling, with the purpose of explaining how
a deep learning model detects tumors in scanned histological tissue samples.
Those methods make the investigated models more interpretable for
gradient-based saliency maps, computed in hidden layers. We test our approach
on different models trained for image classification on ImageNet1K, and models
trained for tumor detection on Camelyon16 and in-house real-world digital
pathology scans of stained tissue samples. Our results show that the
checkerboard noise in the gradient gets reduced, resulting in smoother and
therefore easier to interpret saliency maps.
MoreTranslated text
AI Read Science
Must-Reading Tree
Example
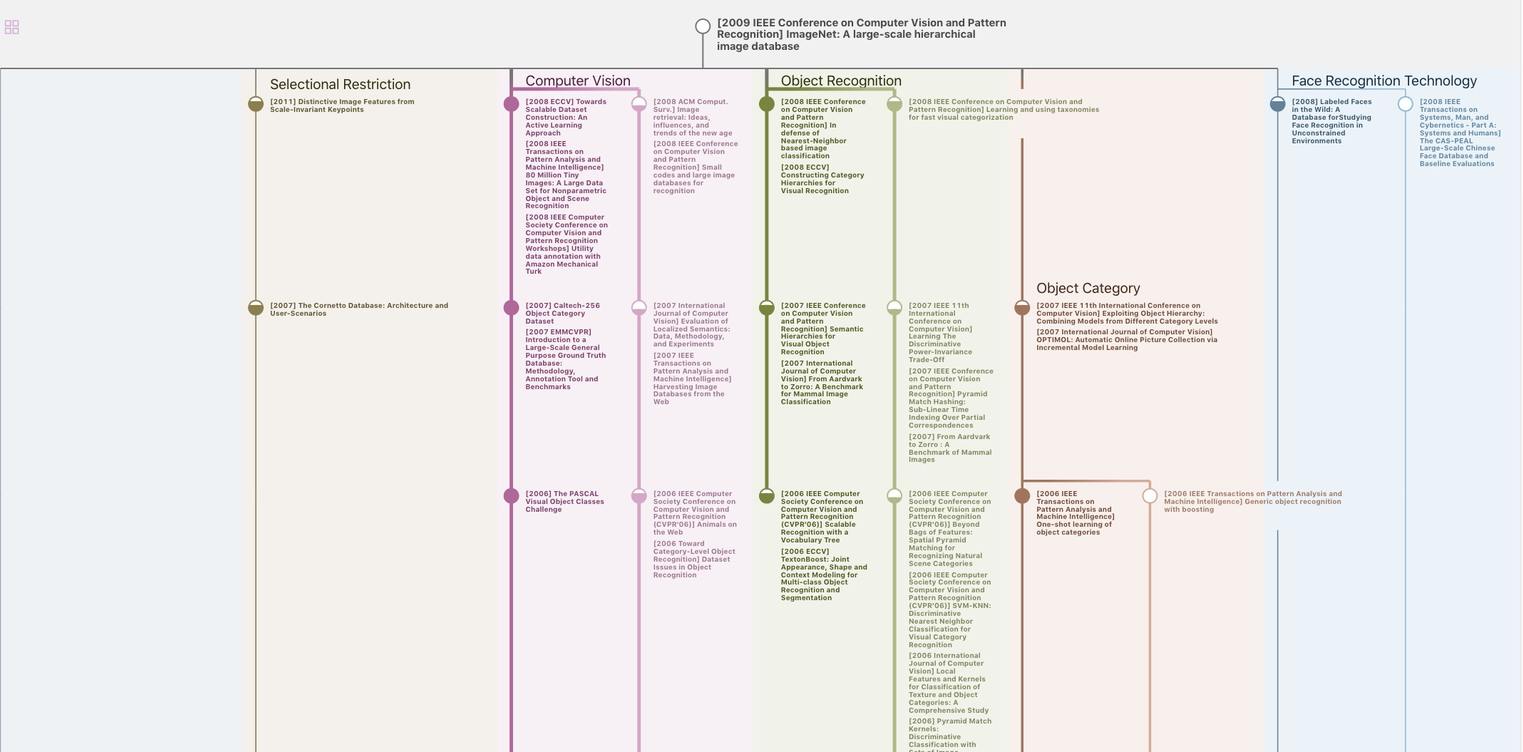
Generate MRT to find the research sequence of this paper
Chat Paper
Summary is being generated by the instructions you defined