DyGGAN: Traffic flow prediction based on generative adversarial network and dynamic graph convolution
2023 5th International Conference on Machine Learning, Big Data and Business Intelligence (MLBDBI)(2023)
摘要
Effective traffic management and planning hinge on precise short-term traffic flow forecasting, a challenging task given the non-Euclidean nature of traffic data and the intricate spatiotemporal dynamics within traffic networks. This research introduces DyGGAN, a novel model combining generative adversarial networks (GANs) and graph convolutional networks (GCNs) to overcome limitations of existing traffic flow prediction algorithms. DyGGAN dynamically adapts by utilizing a dynamic adjacency matrix, ensuring comprehensive learning of each node’s attributes and capturing nuanced spatiotemporal correlations. The model integrates a dynamic graph convolutional network (DGCN) with a spatial attention mechanism, allowing the generator network to adeptly capture dynamic spatial features. A seamless integration of a gated recurrent unit (GRU) network enables understanding of dynamic changes in traffic flow and capturing local temporal dependencies, while a temporal attention mechanism records global dynamic temporal correlations. The synergy of unsupervised and supervised training in DyGGAN produces accurate traffic forecasts, as validated through experiments on real datasets (PEMS04 and PEMS08), showcasing its state-of-the-art performance in addressing the complexities of short-term traffic flow forecasting.
更多查看译文
AI 理解论文
溯源树
样例
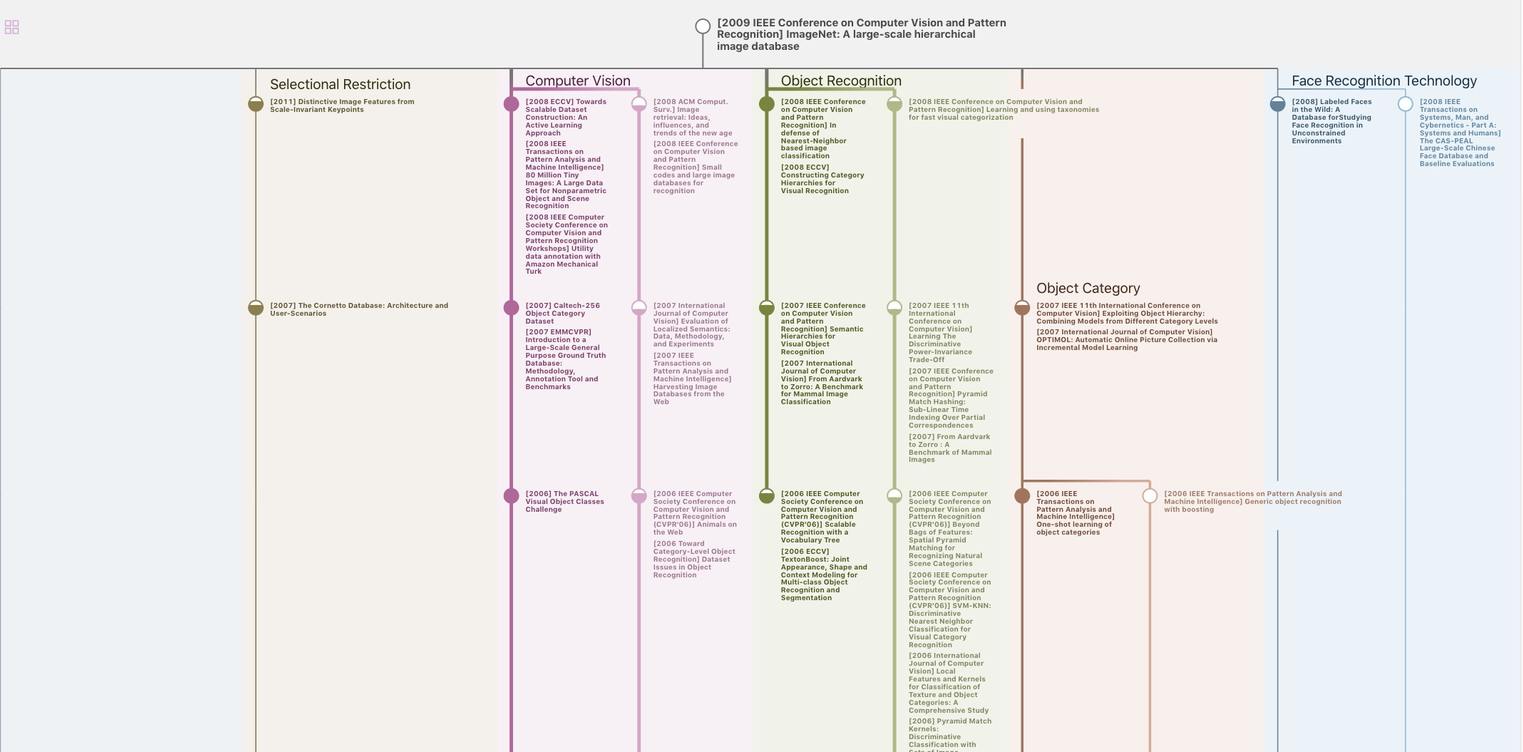
生成溯源树,研究论文发展脉络
Chat Paper
正在生成论文摘要