An Automatic Pipeline for Data Shift Detection and Mitigation to Improve Outcome Prediction of Traumatic Brain Injury
IMAGING INFORMATICS FOR HEALTHCARE, RESEARCH, AND APPLICATIONS, MEDICAL IMAGING 2024(2024)
摘要
Data shift, also known as dataset shift, is a prevalent concern in the field of machine learning. It occurs when the distribution of the data used for training a machine learning model is different from the distribution of the data the model will encounter in a real-world, operational environment (i.e., test set). This issue becomes even more significant in the field of medical imaging due to the multitude of factors that can contribute to data shifts. It is crucial for medical machine learning systems to identify and address these issues. In this paper, we present an automated pipeline designed to identify and alleviate certain types of data shift issues in medical imaging datasets. We intentionally introduce data shift into our dataset to assess and address it within our workflow. More specifically, we employ Principal Components Analysis (PCA) and Maximum Mean Discrepancy (MMD) algorithms to detect data shift between the training and test datasets. We utilize image processing techniques, including data augmentation and image registration methods, to individually and collectively mitigate data shift issues and assess their impacts. In the experiments we use a head CT image dataset of 537 patients with severe traumatic brain injury (sTBI) for patient outcome prediction. Results show that our proposed method is effective in detecting and significantly improving model performance.
更多查看译文
关键词
Deep learning,Data shift,Traumatic brain injury,Classification,Image registration
AI 理解论文
溯源树
样例
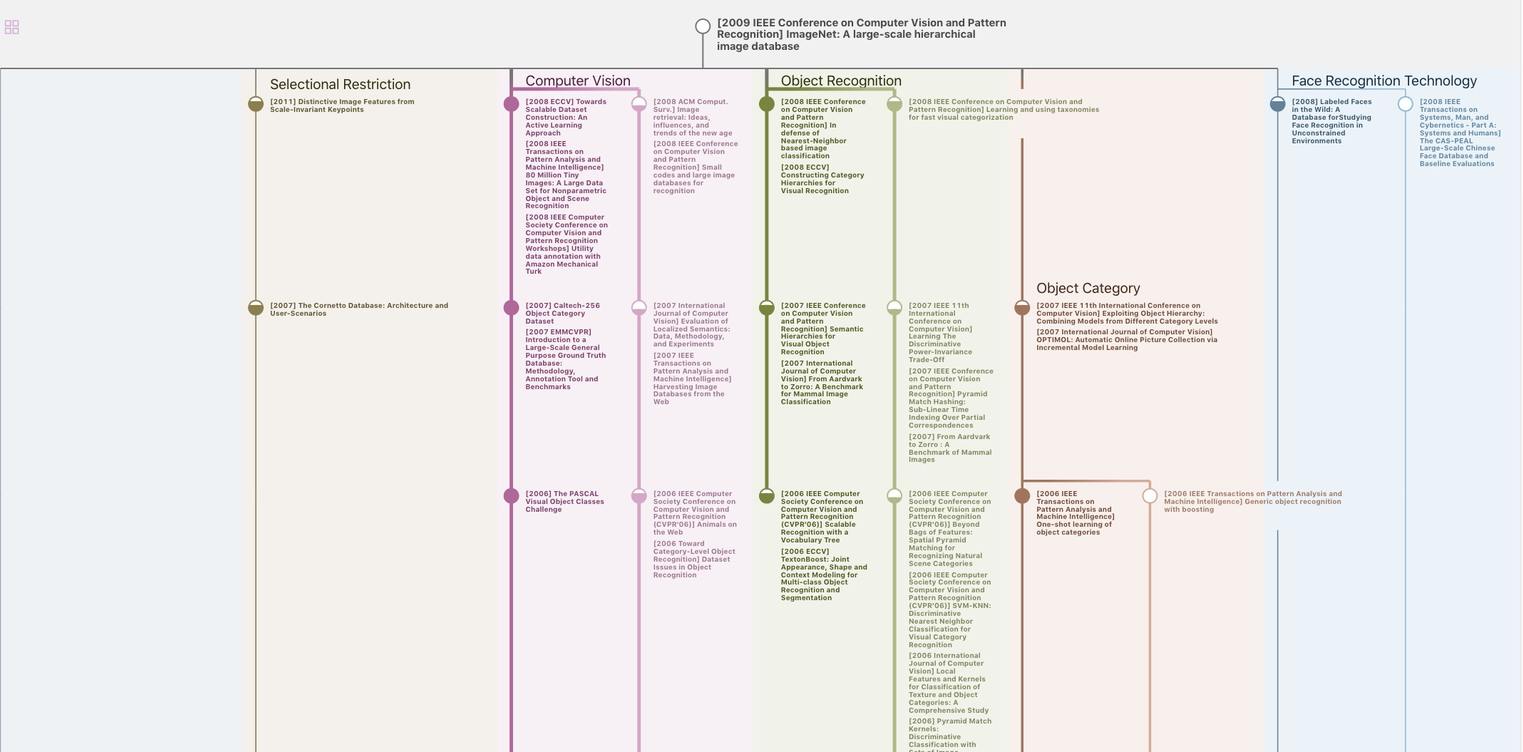
生成溯源树,研究论文发展脉络
Chat Paper
正在生成论文摘要