A predictive model for hypocalcaemia in dairy cows utilizing behavioural sensor data combined with deep learning
Computers and Electronics in Agriculture(2024)
Abstract
(Sub)clinical hypocalcaemia occurs frequently in the dairy industry, and is one of the earliest symptoms of an impaired transition period. Calcium deficiency is accompanied by changes in cows’ daily behavioural variables, which can be measured by sensors. The goal of this study was to construct a predictive model to identify cows at risk of hypocalcaemia in dairy cows using behavioural sensor data. For this study 133 primiparous and 476 multiparous cows from 8 commercial Dutch dairy farms were equipped with neck and leg sensors measuring daily behavioural parameters, including eating, ruminating, standing, lying, and walking behaviour of the 21 days before calving. From each cow, a blood sample was taken within 48 h after calving to measure their blood calcium concentration. Cows with a blood calcium concentration ≤2.0 mmol/L were defined as hypocalcemic. In order to create a more context based cut-off, a second way of dividing the calcium concentrations into two categories was proposed, using a linear mixed-effects model with a k-Means clustering. Three possible binary predictive models were tested; a logistic regression model, a XgBoost model and a LSTM deep learning model. The models were expanded by adding the following static features as input variables; parity (1, 2 or 3+), calving season (summer, autumn, winter, spring), day of calcium sampling relative to calving (0, 1 or 2), body condition score and locomotion score. Of the three models, the deep learning model performed best with an area under the receiver operating characteristic curve (AUC) of 0.71 and an average precision of 0.47. This final model was constructed with the addition of the static features, since they improved the model’s tuning AUC with 0.11. The calcium label based on the cut-off categorization method proved to be easier to predict for the models compared to the categorization method with the k-means clustering. This study provides a novel approach for the prediction of hypocalcaemia, and an ameliorated version of the deep learning model proposed in this study could serve as a tool to help monitor herd calcium status and to identify animals at risk for associated transition diseases.
MoreTranslated text
Key words
Dairy cattle,Hypocalcaemia,Sensors,Deep learning,Prediction,Transition period
AI Read Science
Must-Reading Tree
Example
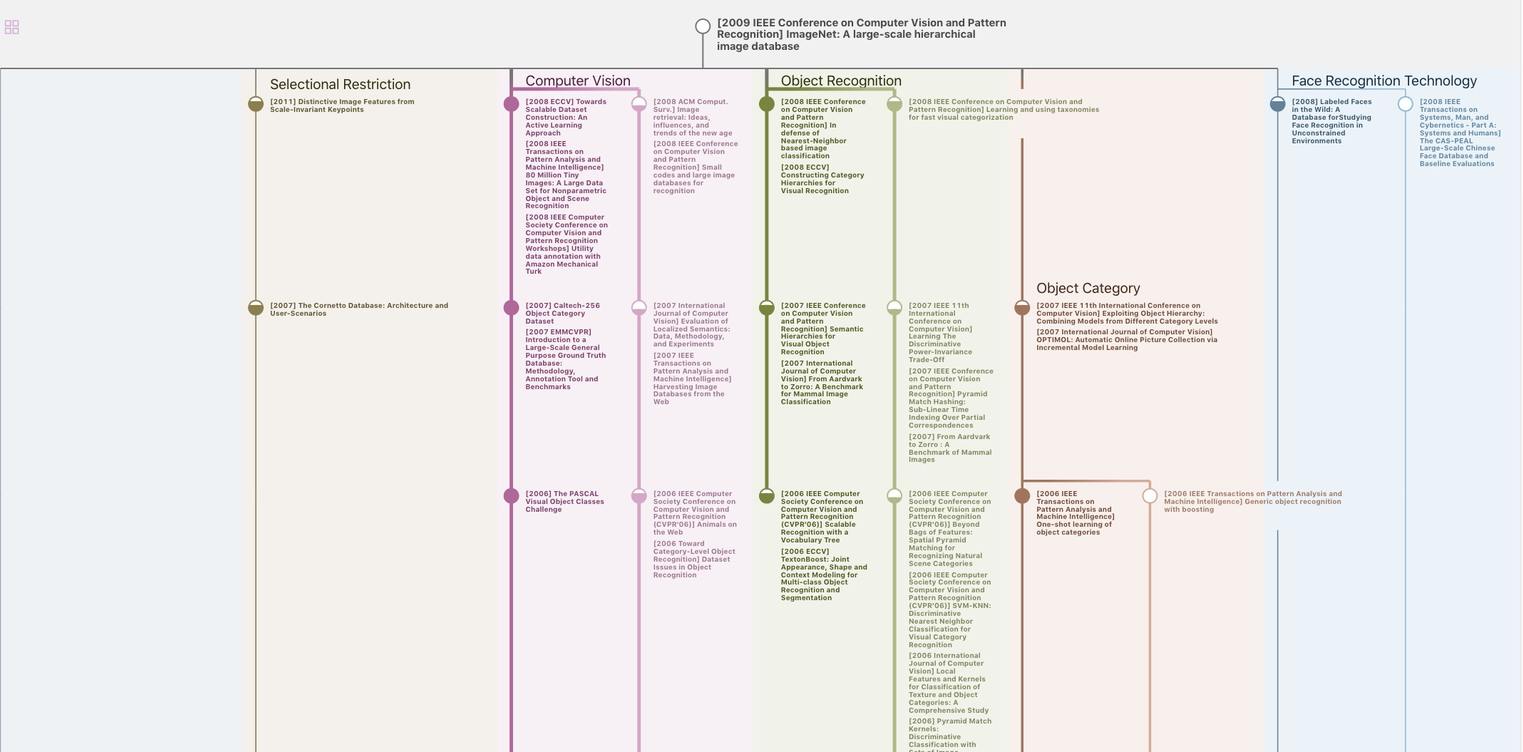
Generate MRT to find the research sequence of this paper
Chat Paper
Summary is being generated by the instructions you defined