Fine Tuning of large language Models for Arabic Language.
ACS/IEEE International Conference on Computer Systems and Applications(2023)
Abstract
In recent years, Long language models have made significant progress, enabling machines to interpret and process human language. However, the Arabic language presents unique challenges due to its rich morphology and diverse sentence structures. The development of specialized language models for Arabic question answering has implications for improved human- computer interaction, cultural preservation, and accessibility. This paper aims to enhance the comprehension and contextual understanding of Arabic-posed questions by leveraging the capabilities of the LLaMa language model and the XLNet transformer. The ARCD dataset, which mainly consists of an Arabic dataset for question-answering, was used to fine- tune the LLaMa 2.0 and XLNet. By utilizing LLaMA and XLNet transformers separately, This paper contributes to the construction of an NLP pipeline that can properly understand and process Arabic text to provide answers depending on a particular Arabic context by using LLaMA and XLNet transformers individually. It is important to note that Arabic datasets were not previously used to train the LLaMa language model. The LLaMA language model received accuracy scores of 93.70
MoreTranslated text
AI Read Science
Must-Reading Tree
Example
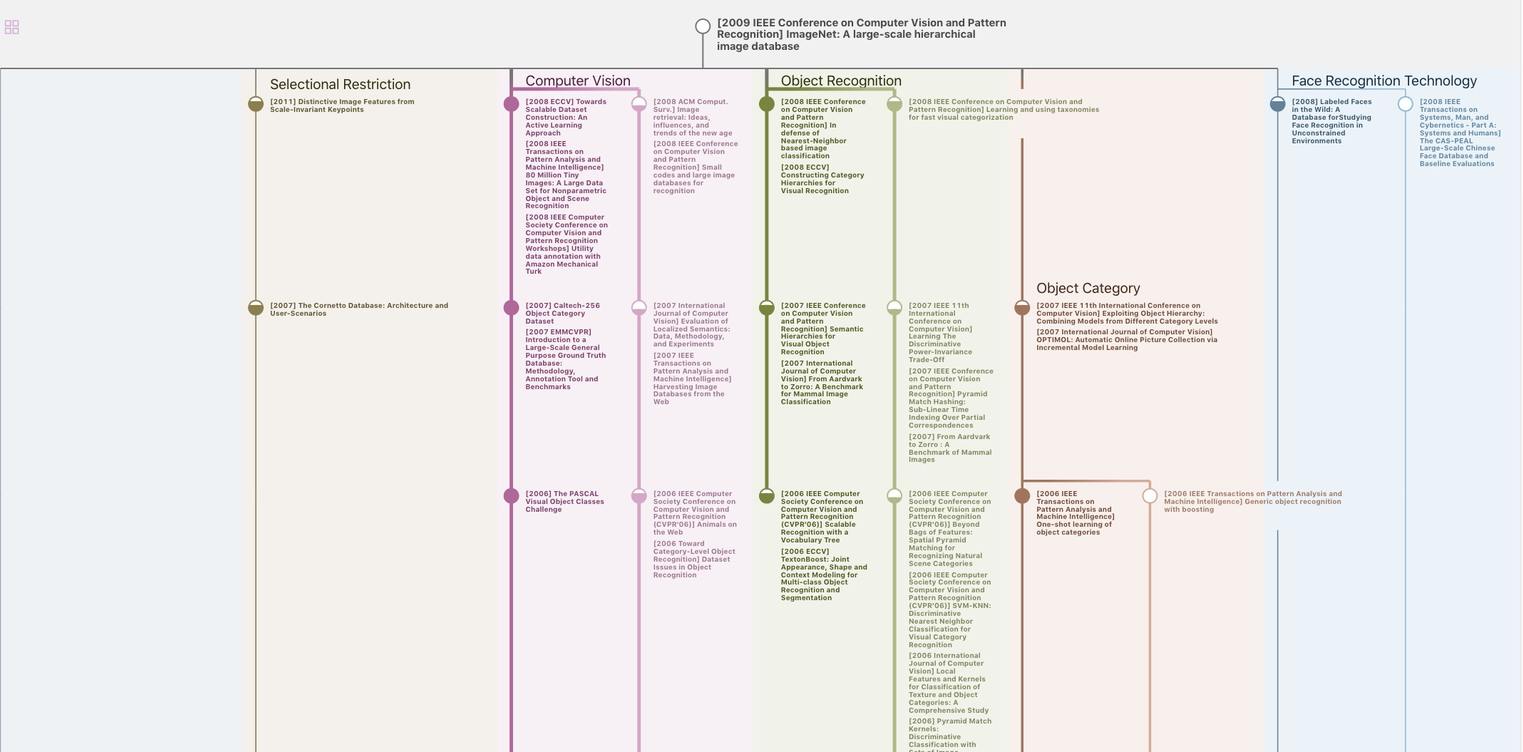
Generate MRT to find the research sequence of this paper
Chat Paper
Summary is being generated by the instructions you defined