Hybrid Deep Learning Approach for Hydraulic Fracture Morphology Diagnosis in Coalbed Methane Wells
ENERGY & FUELS(2024)
摘要
Accurately diagnosing hydraulic fracture morphology is crucial for coalbed methane (CBM) development and strategy formulation. However, achieving precise and efficient diagnosis of fracture morphology remains a challenge in complex geological and engineering environments. Therefore, this study proposes a hybrid deep learning method based on multivariate time series classification. In this method, the fracturing curve is compared to the "language" of the CBM reservoir, with fracture morphology representing the semantics of this "language". This method integrates a wavelet transform module and a deep encoder-decoder module. The wavelet transform module aims to reduce signal noise and enhance model robustness, while the deep encoder-decoder module extracts latent features from the fracturing curve. Integrating hydraulic fracture morphology recognition into a hybrid deep learning model composed of diverse deep neural networks tailored for specific predictive tasks maximizes the strengths of each network component. This approach was evaluated using real data from CBM blocks in Northern China, supported by ablation experiments, numerical simulations, and microseismic interpretation, demonstrating its effectiveness and applicability. In contrast to traditional time series deep learning models, this method enables more robust extraction of advanced temporal features and delivers high-precision predictions. This novel approach provides an effective solution for diagnosing hydraulic fracturing morphology and contributes to guiding refracturing.
更多查看译文
AI 理解论文
溯源树
样例
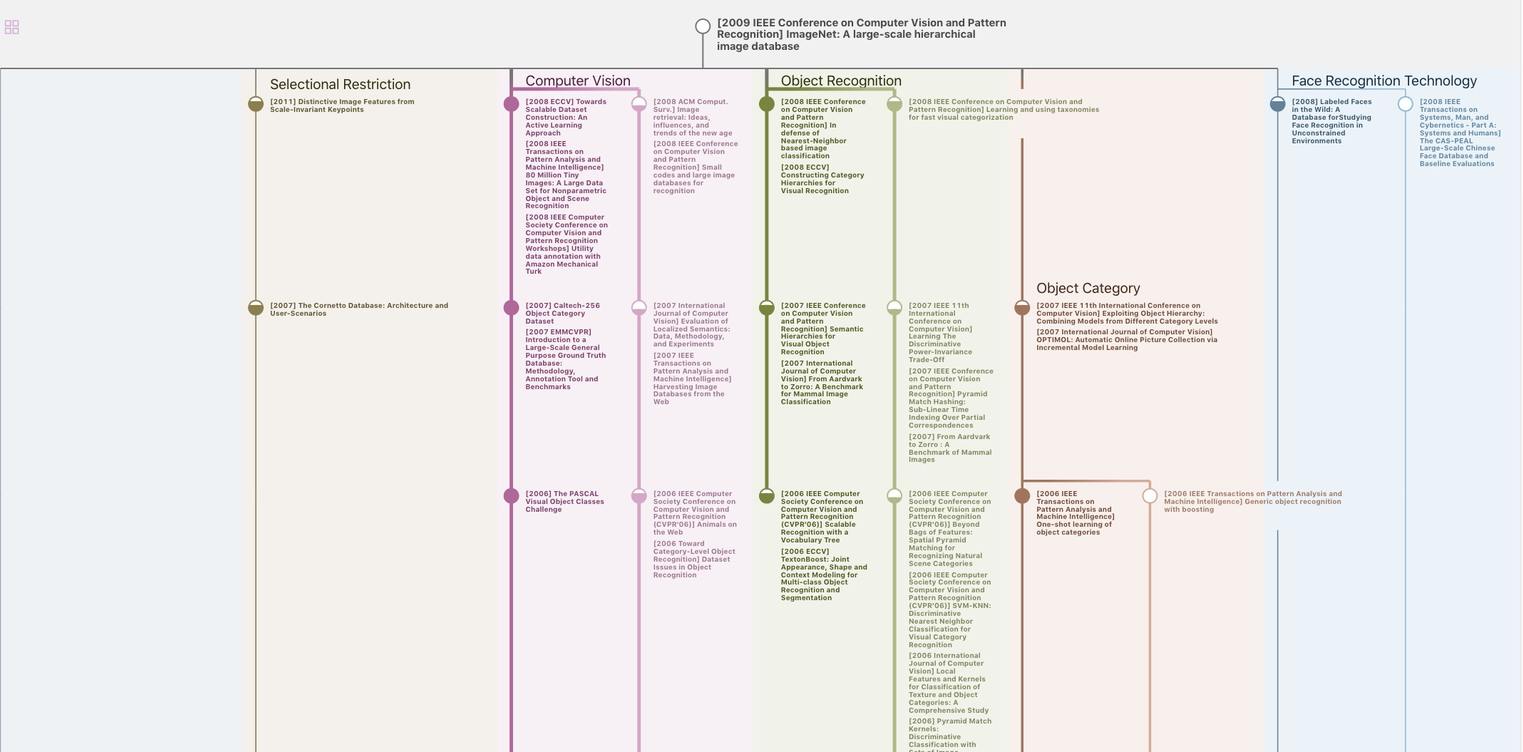
生成溯源树,研究论文发展脉络
Chat Paper
正在生成论文摘要