Comparing cardiac artifact removal algorithms for endovascular brain-computer interface recordings.
International Winter Conference on Brain-Computer Interface(2024)
摘要
Cardiac artifacts have been observed in brain recordings captured by the endovascular Stentrode(TM) device. These artifacts are an order of magnitude larger than underlying neural activity and impact upon brain-computer interface (BCI) performance. In this study, we compared six cardiac artifact removal algorithms and found that removing artifacts improved performance. The BCI classification accuracies for 11 “Rest vs. Go” sessions with one participant were compared for three widely used BCI decoding methods. Baseline performance before removing cardiac artifacts was 71.4% for decoding the whole power spectrum, which increased to 80% if we only decoded high gamma activity (80-300 Hz). Removing artifacts and decoding on the whole frequency spectrum gave 86% accuracy using amplitude-matched common average referencing (AMCAR). Using data from a single, best-performing channel increased performance to 89%. After correcting artifacts, raw data with a random forest classifier outperformed other BCI decoding methods. This research suggests that removing cardiac artifacts improves task-related neural feature extraction in low frequencies, which leads to better classification performance of endovascular BCIs.
更多查看译文
AI 理解论文
溯源树
样例
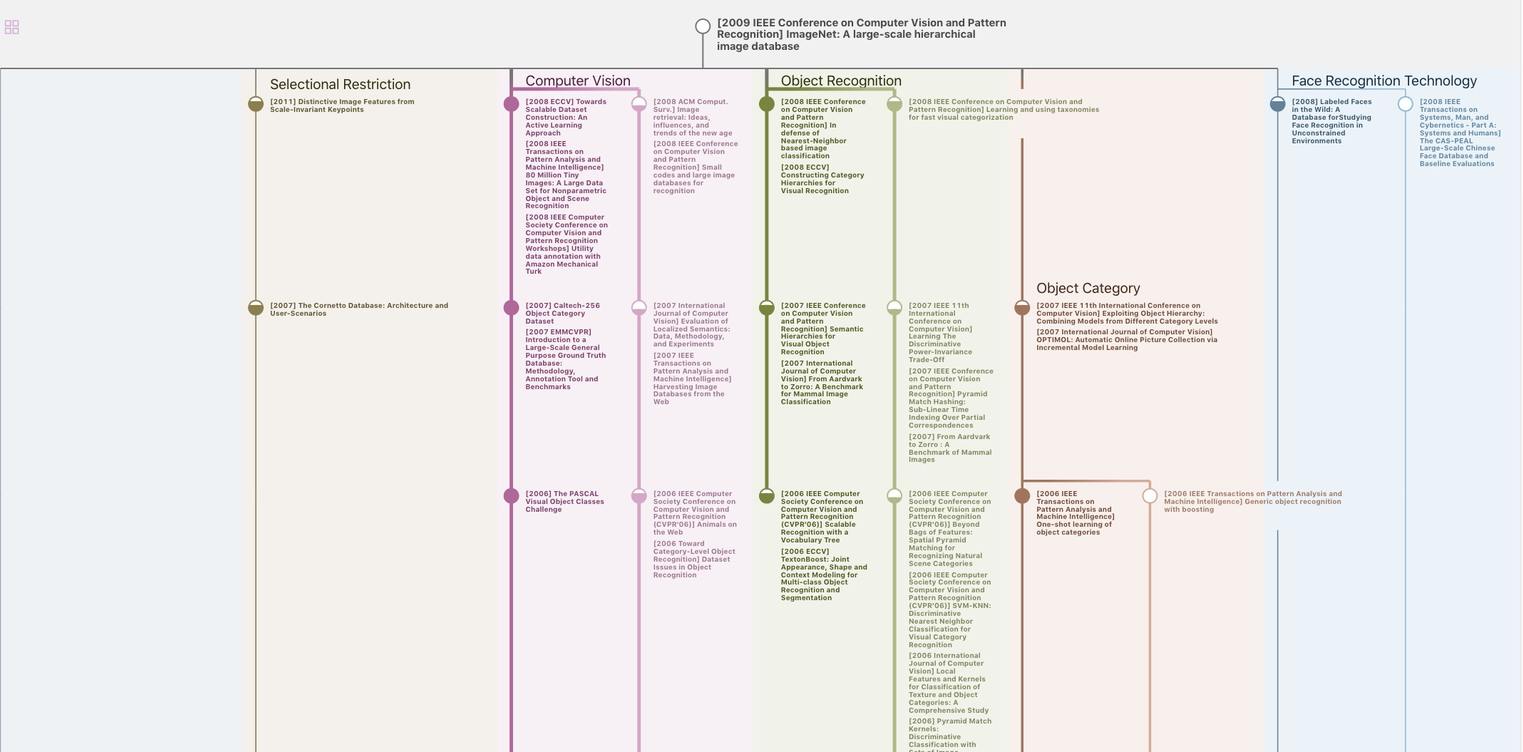
生成溯源树,研究论文发展脉络
Chat Paper
正在生成论文摘要