Low-Query-Cost Data-Free Black-Box Attacks
2023 5th International Conference on Machine Learning, Big Data and Business Intelligence (MLBDBI)(2023)
摘要
In data-free black box attacks, attackers are unable to access the training data and detailed model knowledge of the target model. Recently, methods based on Generative Adversarial Networks (GANs) have shown significant performance in such attacks. However, previous approaches encountered issues such as slow convergence and model collapse, which constrained the effectiveness of these attacks. These methods also overlooked the fact that model querying constitutes resource expenditure, since too many queries can raise the victim’s suspicions and lead to attack failure. To resolve this problem, we propose a data-free black box attack method with a low query cost. Our method is divided into three stages: firstly, a generative model is trained on a public image dataset, which is then used to generate a training dataset. Secondly, the target model is queried using this generated training dataset to obtain corresponding labels while training a substitute model. Finally, when the query budget has been depleted, Self-paced learning is employed to label the remaining training data and to further train the substitute model. Extensive experiments have shown that, compared to other black box attack methods, our approach achieves a higher rate of attack success with a smaller query budget.
更多查看译文
AI 理解论文
溯源树
样例
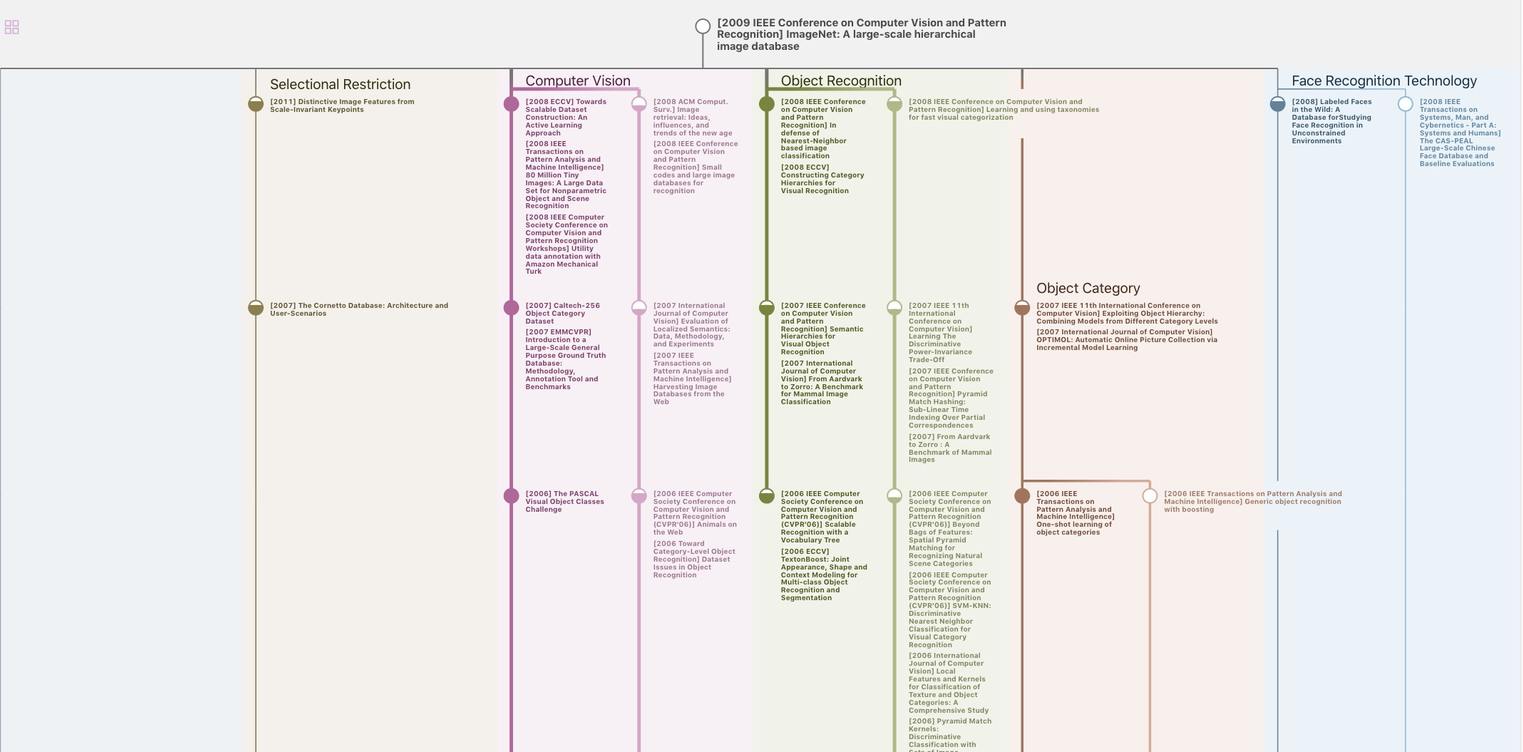
生成溯源树,研究论文发展脉络
Chat Paper
正在生成论文摘要