Natural Language Processing to Classify Caregiver Strategies for Participation-Focused Pediatric Rehabilitation
Archives of Physical Medicine and Rehabilitation(2024)
摘要
Research Objectives
There is limited use of artificial intelligence for undertaking the complex task of participation-focused goal setting in pediatric rehabilitation. We therefore explored using natural language processing (NLP) to automate the classification of participation-focused caregiver strategies generated by the Young Children's Participation and Environment Measure (YC-PEM) into key predictors of participation (i.e., the child's environment/context, activity competencies, preferences, and sense of self).
Design
Cross-sectional study design.
Setting
Home and community setting.
Participants
125 caregivers of young children receiving pediatric rehabilitation services in an early intervention (EI) service context. Most caregivers had pursued graduate education (56%). Their children were mostly male (56%), aged between 2 and 3 years (56%), and received services through EI because of developmental delay (68%).
Interventions
N/A.
Main Outcome Measures
Caregivers completed the YC-PEM with open-ended items asking caregivers to report on their strategies to support their child's participation in valued activities. This resulted in a total of 780 caregiver strategies that were manually and independently annotated by two research staff according to five classes (i.e., four key predictors of participation and a non-strategy class for irrelevant or indeterminate instances). Multinomial classification of these caregiver strategies was pursued across these five classes, experimenting with various classical machine learning and Transformer-based classification models commonly used in NLP (i.e., logistic regression, naïve Bayes, Bidirectional Encoder Representation from Transformers (BERT), and Bio-ClinicalBERT). The top-performing model was then used for subsequent experiments, including pipelined approaches classifying strategies into the five classes through stepwise classifications tasks that increase in their specificity.
Results
BERT achieved highest performance overall, with accuracy=64.47%, precision=0.73, recall=0.53 and F1=0.56. This Transformer-based model was, therefore, used for subsequent analyses testing classification tasks within pipelined approaches, which reached accuracy=58.06-88.15%, precision=0.56-0.82, recall=0.53-0.76 and F1=0.51-0.79.
Conclusions
Findings provide preliminary evidence for establishing an automated classification of caregiver strategies within participation-focused goal setting interventions under development that employ data from a Participation and Environment Measure.
Author(s) Disclosures
YC-PEM is licensed for distribution through CanChild Centre for Childhood Disability Research. Revenue from YC-PEM sales supports research and development activities.
更多查看译文
关键词
Pediatrics,Rehabilitation,Natural Language Processing,Caregiver
AI 理解论文
溯源树
样例
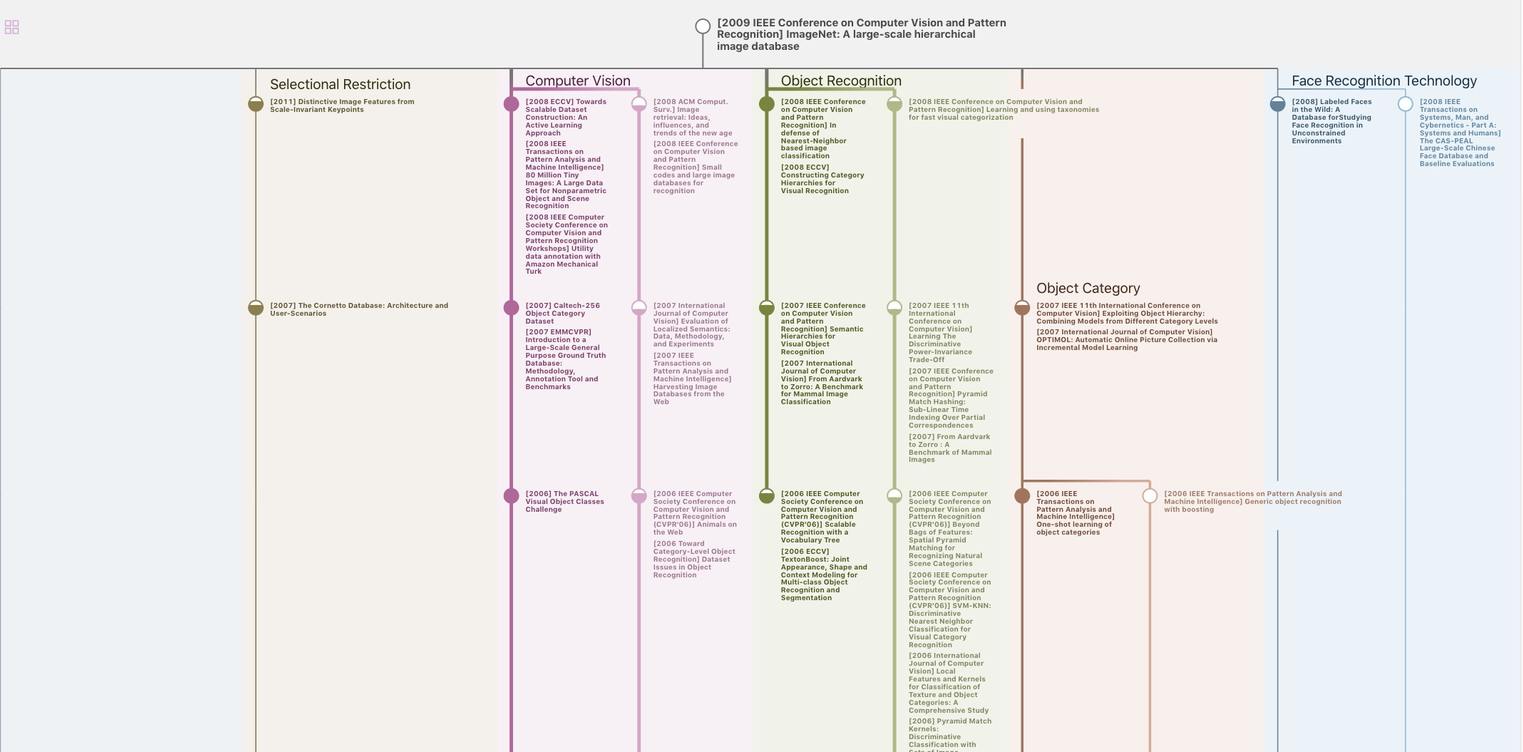
生成溯源树,研究论文发展脉络
Chat Paper
正在生成论文摘要