Genes in Humans and Mice: Insights from Deep learning of 777K Bulk Transcriptomes
crossref(2024)
Abstract
Mice are widely used as animal models in biomedical research, favored for their small size, ease of breeding, and anatomical and physiological similarities to humans1,2. However, discrepancies between mouse gene experiment results and the actual behavior of human genes are not uncommon, despite their shared DNA sequence similarity3-8. This suggests that DNA sequence similarity does not always reliably predict functional similarity. On the other hand, RNA expression of genes could offer additional information about gene function9,10. However, comprehensive characterization of genes through their expression can be challenging with traditional methods, due to the dynamic nature of gene expression and its high variability in different biological contexts. In this study, we undertook characterization and inter-species comparison of human and mouse genes by applying innovative deep learning methodologies on a large dataset of 410K human and 366K mouse bulk RNA-seq samples. This was achieved by using gene representations from our Transformer-based GeneRAIN model11,12. These gene representations, aggregating information from large gene expression datasets, provided insights beyond DNA sequence similarity, helping to elucidate differences in disease and phenotype associations between human and mouse genes. We propose that this approach will support future decision making around whether the mouse will be an appropriate model for studying specific human genes, and whether the results of specific mouse gene studies are likely to be recapitulated in humans. Our methodological innovations offer valuable lessons for future deep learning applications in cross-species omics data. The interspecies gene relationship findings from our study can contribute valuable insights to enhance our understanding of the biology and evolution of the two species.
### Competing Interest Statement
F.V. declares commercial association with OmniOmics.AI Pty Ltd.
MoreTranslated text
AI Read Science
Must-Reading Tree
Example
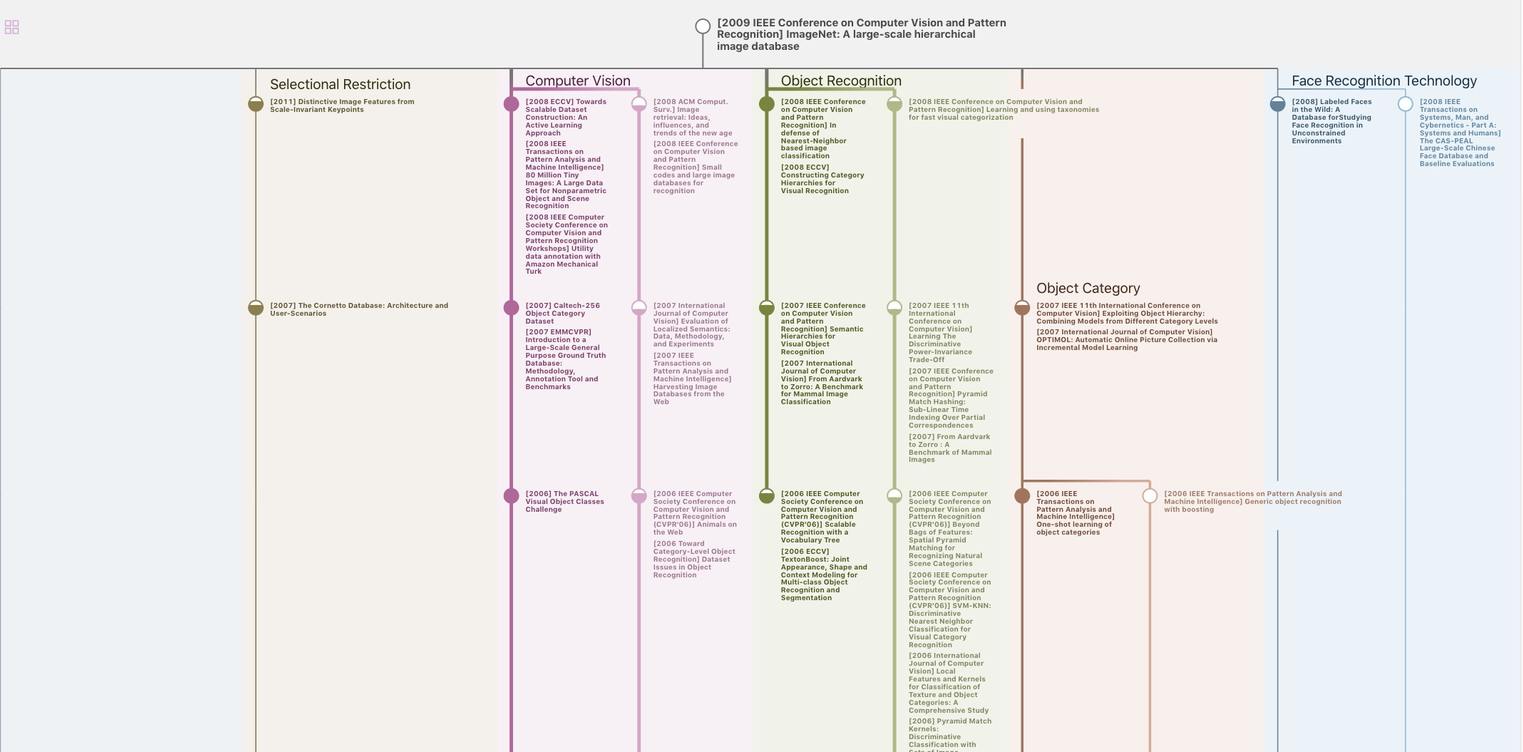
Generate MRT to find the research sequence of this paper
Chat Paper
Summary is being generated by the instructions you defined