Ensemble Methods with [18F]FDG-PET/CT Radiomics in Breast Cancer Response Prediction
PATTERN RECOGNITION AND MACHINE INTELLIGENCE, PREMI 2023(2023)
Abstract
Pathological complete response (pCR) after neoadjuvant che-motherapy (NAC) in patients with breast cancer was found to improve survival, and it has a great prognostic value in the aggressive tumor subtype. This study aims to predict pCR before NAC treatment with a radiomic feature-based ensemble learning model using both positron emission tomography/computed tomography (PET/CT) images taken from the online QIN-Breast dataset. It studies the problem of constructing an end-to-end classification pipeline that includes a large-scale radiomic feature extraction, a hybrid iterative feature selection and a heterogeneous weighted ensemble classification. The proposed hybrid feature selection procedure can identify significant radiomic predictors out of 2153 features extracted from delineated tumour regions. The proposed weighted ensemble approach aggregates the outcomes of four weak classifiers (Decision tree, Naive Bayes, K-nearest neighbour, and Logistics regression) based on their importance. The empirical study demonstrates that the proposed feature selection-cum-ensemble classification method has achieved 92% and 88.4% balanced accuracy in PET and CT, respectively. The PET/CT aggregated model performed better and achieved 98% balanced accuracy and 94.74% F1-score. Furthermore, this study is the first classification work on the online QIN-Breast dataset.
MoreTranslated text
Key words
Breast cancer,Ensemble learning,Radiomics,PET/CT,Pathologic complete response
AI Read Science
Must-Reading Tree
Example
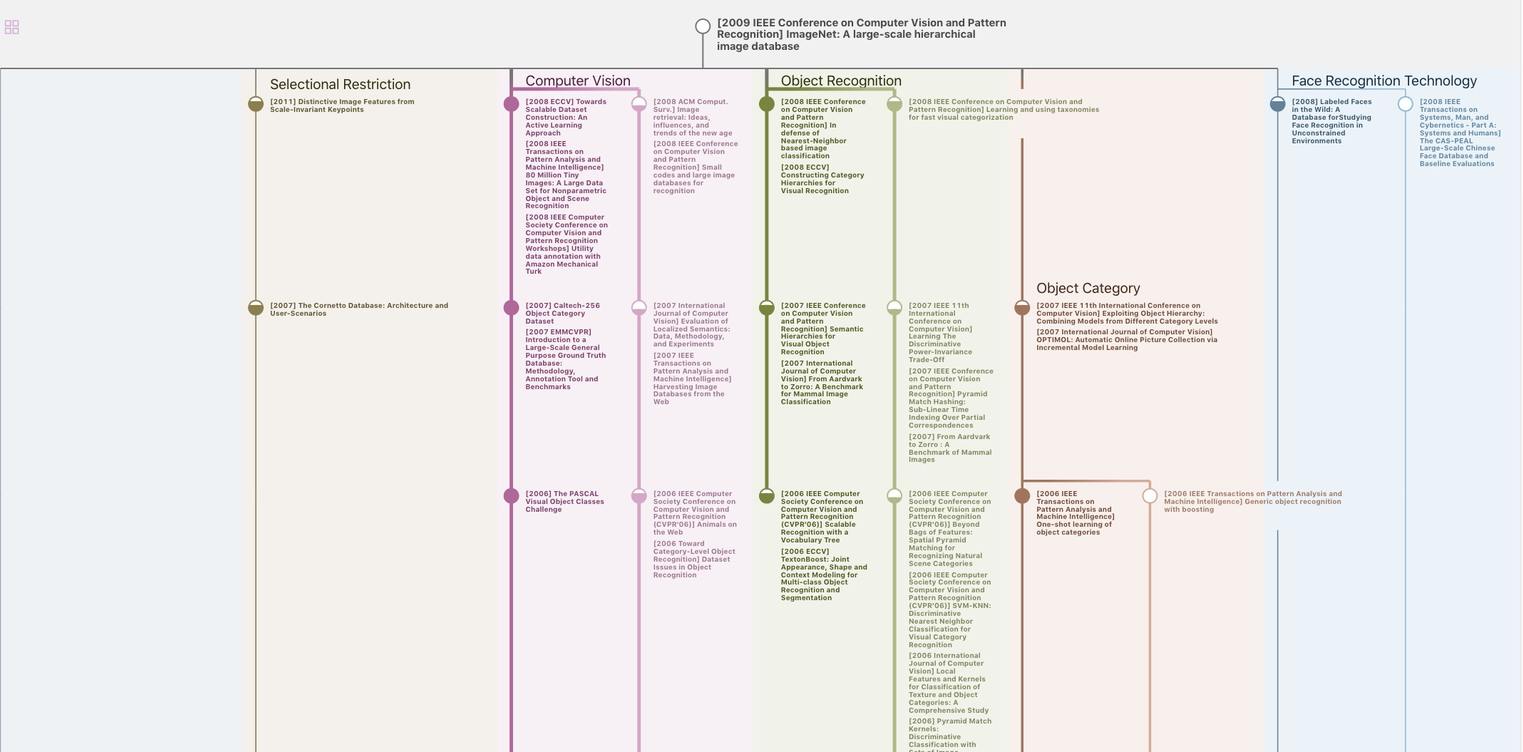
Generate MRT to find the research sequence of this paper
Chat Paper
Summary is being generated by the instructions you defined