East Asia Atmospheric River Forecast With a Deep Learning Method: GAN-UNet
JOURNAL OF GEOPHYSICAL RESEARCH-ATMOSPHERES(2024)
摘要
Accurate forecasting of atmospheric rivers (ARs) holds significance in preventing losses from extreme precipitation. However, traditional numerical weather prediction (NWP) models are computationally expensive and can be limited in accuracy due to inaccurate physical parameter settings. To overcome these limitations, we propose a deep learning (DL) model, called GAN-UNet, to forecast the AR occurrence, position, and intensity in East Asia. GAN-UNet can capture the complex nonlinear relationship between the inputs at the past moment, including the vertically integrated water vapor transport (IVT), zonal wind at 850 hPa (U850), and meridional wind at 850 hPa (V850), and the forecast output (IVT, U850, or V850), whose results are comparable to NWP models. In addition, the average model (AM) by integrating the results generated by GAN-UNet and European Centre for Medium-Range Weather Forecasts (ECMWF) outperforms all the NWP models selected in this study, demonstrating its potential to improve the performance of NWP through the DL method. Specifically, the 5-day average F1 scores of the AM are 0.777 and 0.845, whose values are significantly better than those obtained by ECMWF (0.712 and 0.794) in the two key regions of East Asia; The AM 5-day average intersection over unions are 0.706 and 0.688 while the values of ECMWF are 0.675 and 0.64; in terms of intensity forecast, GAN-UNet and AM exhibited lower differences in most of the intensity bins, except for the final bin with IVT more than 825 kg m-1 s-1. With this thorough analysis, GAN-UNet is shown as an effective model to forecast ARs. This work presents a deep learning (DL) model (called GAN-UNet) for the spatio-temporal atmospheric rivers (ARs) forecast. Our goal is to perform long lead-time AR event forecasts accurately and efficiently. The data from 1959 to 2022 over 40 degrees E-180 degrees E, 20 degrees S-60 degrees N are used for training, validation, and testing. The results show that the proposed GAN-UNet can forecast the AR occurrence, position, and intensity accurately and its performance is comparable with the state-of-the-art numerical weather prediction (NWP) model. In addition, the ensemble of GAN-UNet and the NWP model outperform all the NWP models selected in this study. However, The DL model still has certain limitation. Specifically, while GAN-UNet and the ensemble model demonstrate superior forecast capabilities for most intensity bins in AR events compared to NWP models, they are severely underestimated for the grids of the final bin. The results show that the DL model can effectively forecast AR events in East Asia, and further improve the forecast accuracy of NWP by combining it with NWP model, which holds significance in extreme precipitation forecast. A deep learning model (GAN-UNet) is developed for efficient and accurate atmospheric river forecast GAN-UNet achieves comparable performance to ECMWF and NCEP on atmospheric river forecast The outcomes obtained from the average of GAN-UNet and NWP exhibit superior performance compared to all the individual NWP models selected in this study
更多查看译文
AI 理解论文
溯源树
样例
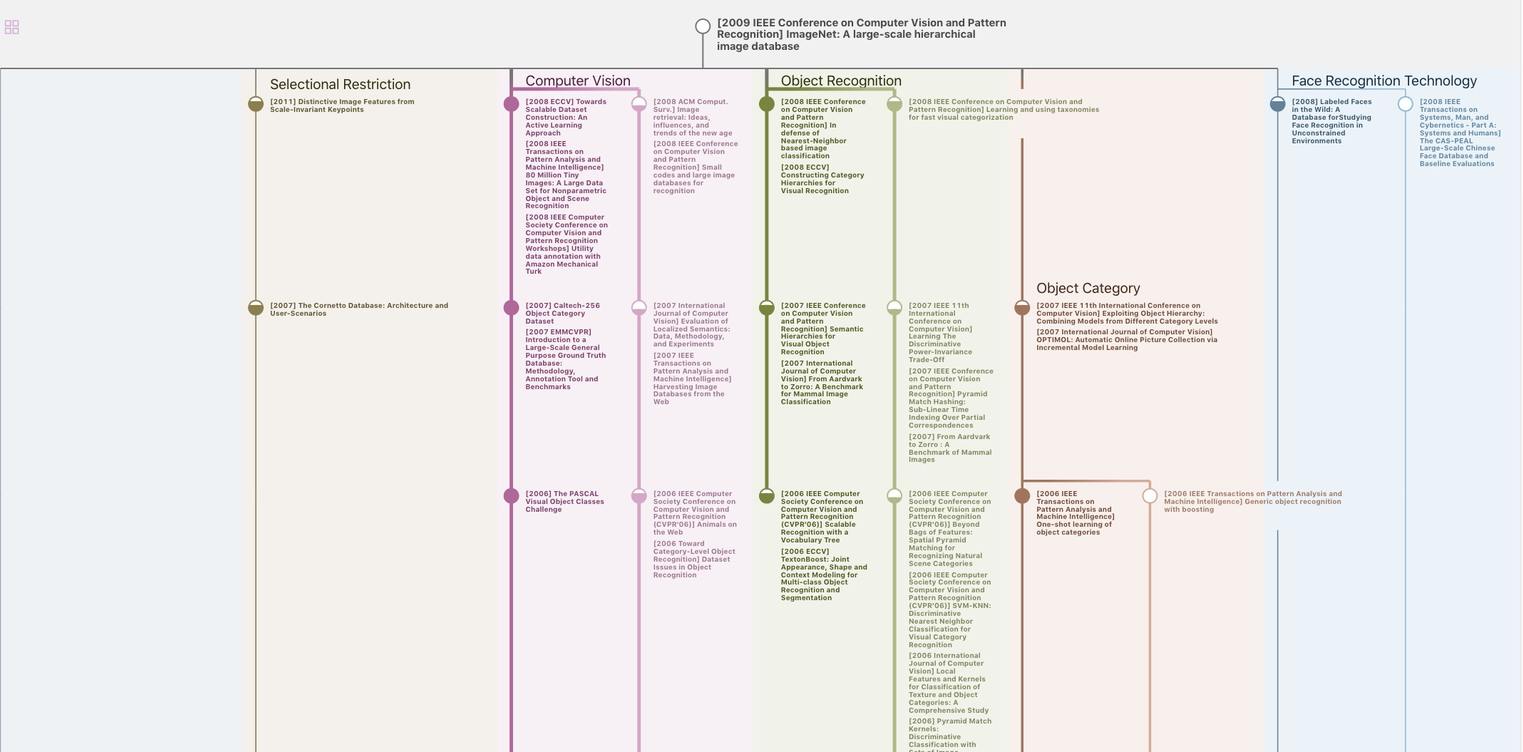
生成溯源树,研究论文发展脉络
Chat Paper
正在生成论文摘要