Risk assessment of atherosclerotic cardiovascular disease based on feature selection of L1-CBFS
BIOMEDICAL SIGNAL PROCESSING AND CONTROL(2024)
Abstract
To achieve risk assessment of atherosclerotic cardiovascular disease, the number of features directly affects the performance of the model. In this paper, we propose a L1 regularized mutual information feature selection algorithm based on copula (L1-CBFS). The data set was based on real medical visit data, including pulse wave velocities measured by the ultrafast pulse wave velocity (ufPWV) device. Firstly, the L1-CBFS algorithm was combined with four classifiers, namely, Gradient lifting tree (GBDT), Ultimate Gradient Enhancement (XGBoost), Light gradient Enhancement Machine (LightGBM) and Random Forest (RF), to evaluate the classification performance. Finally, the optimal feature subset that the feature selection algorithm contributes significantly to the target task is obtained. The experimental results show that L1-CBFS-LightGBM model has the best performance, the accuracy of 91.34%, macro precision of 91.39%, macro recall of 91.07%, and macro F1 score of 90.4%.
MoreTranslated text
Key words
ASCVD,CBFS,L1 regularization,Disease risk assessment
AI Read Science
Must-Reading Tree
Example
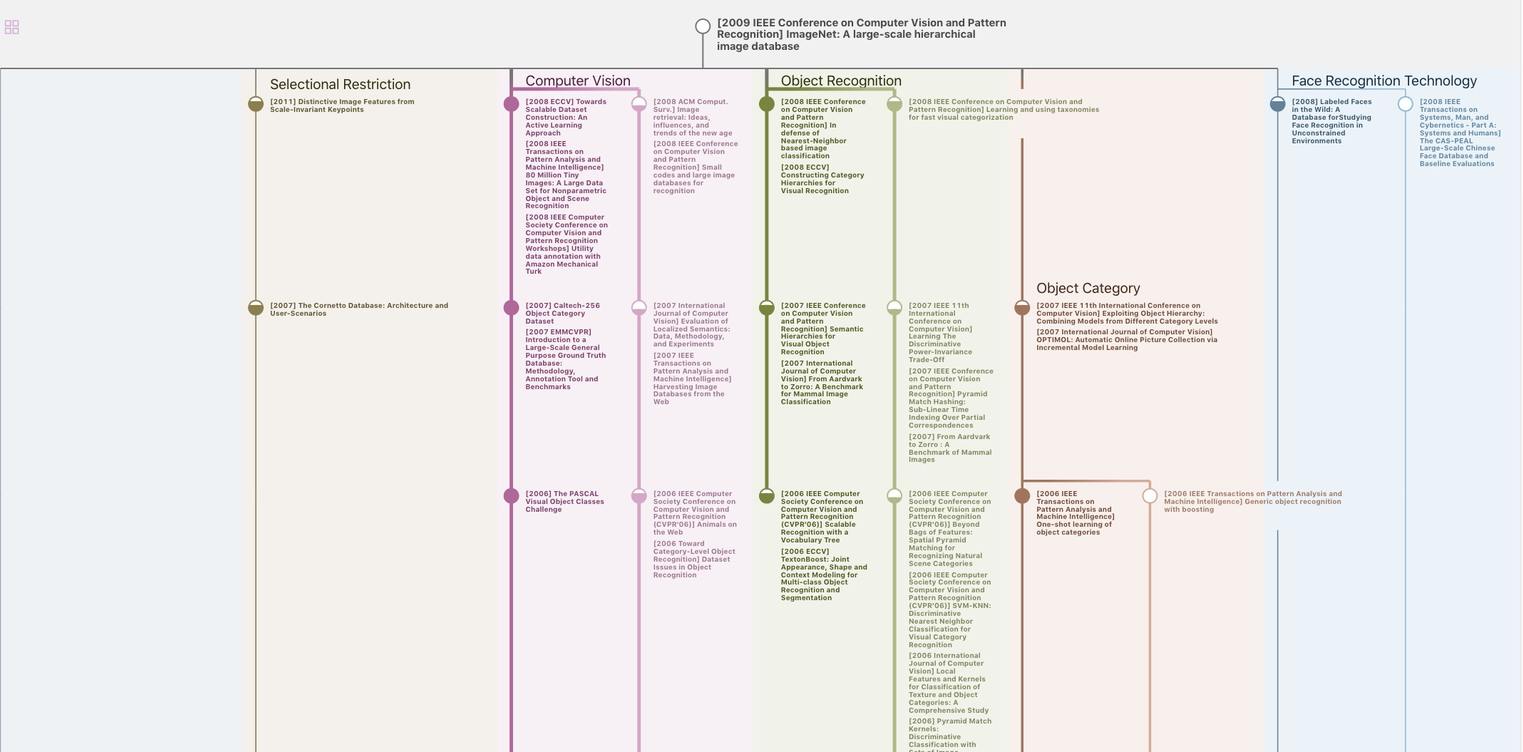
Generate MRT to find the research sequence of this paper
Chat Paper
Summary is being generated by the instructions you defined