BI-GCN: Bilateral Interactive Graph Convolutional Network for Recommendation
PROCEEDINGS OF THE 32ND ACM INTERNATIONAL CONFERENCE ON INFORMATION AND KNOWLEDGE MANAGEMENT, CIKM 2023(2023)
摘要
Recently, Graph Convolutional Network (GCN) based methods have become novel state-of-the-arts for Collaborative Filtering (CF) based Recommender Systems. To obtain users' preferences over different items, it is a common practice to learn representations of users and items by performing embedding propagation on a user-item bipartite graph, and then calculate the preference scores based on the representations. However, in most existing algorithms, user/item representations are generated independently of target items/users. To address this problem, we propose a novel graph attention model named Bilateral Interactive GCN (BI-GCN), which introduces bilateral interactive guidance into each user-item pair and thus leads to target-aware representations for preference prediction. Specifically, to learn the user/item representation from its neighborhood, we assign higher attention weights to those neighbors similar to the target item/user. By this manner, we can obtain target-aware representations, i.e., the information of the target item/user is explicitly encoded in the corresponding user/item representation, for more precise matching. Extensive experiments1on three benchmark datasets demonstrate the effectiveness and robustness of BI-GCN.
更多查看译文
关键词
Recommender Systems,Collaborative Filtering,Graph Convolutional Networks,Attention Mechanism
AI 理解论文
溯源树
样例
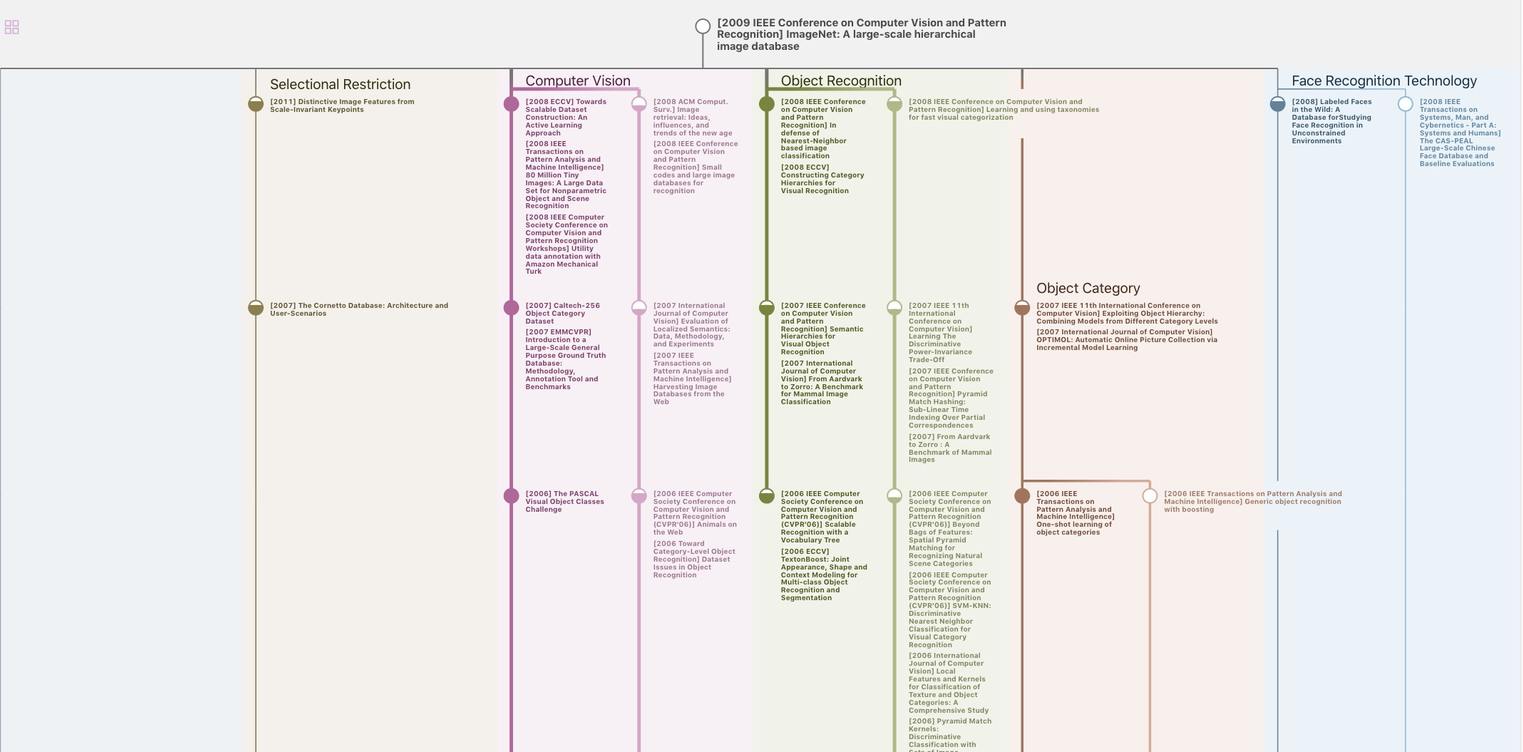
生成溯源树,研究论文发展脉络
Chat Paper
正在生成论文摘要