Loss and Likelihood Based Membership Inference of Diffusion Models
INFORMATION SECURITY, ISC 2023(2023)
摘要
Recent years have witnessed the tremendous success of diffusion models in data synthesis. However, when diffusion models are applied to sensitive data, they also give rise to severe privacy concerns. In this paper, we present a comprehensive study about membership inference attacks against diffusion models, which aims to infer whether a sample was used to train the model. Two attack methods are proposed, namely loss-based and likelihood-based attacks. Our attack methods are evaluated on several state-of-the-art diffusion models, over different datasets in relation to privacy-sensitive data. Extensive experimental evaluations reveal the relationship between membership leakages and generative mechanisms of diffusion models. Furthermore, we exhaustively investigate various factors which can affect membership inference. Finally, we evaluate the membership risks of diffusion models trained with differential privacy.
更多查看译文
关键词
Membership inference attacks,Diffusion models,Human face synthesis,Medical image generation,Privacy threats
AI 理解论文
溯源树
样例
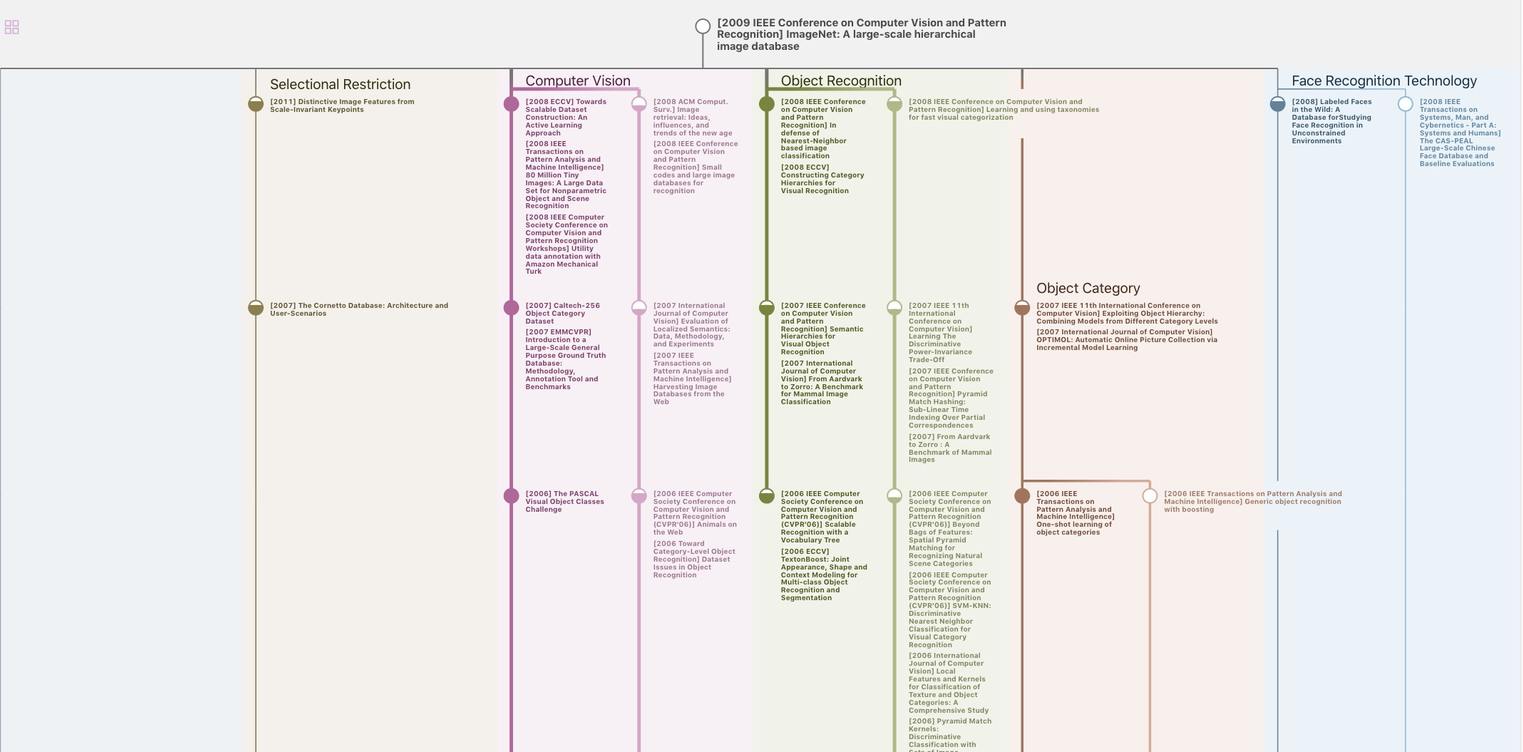
生成溯源树,研究论文发展脉络
Chat Paper
正在生成论文摘要